Abstract
The rational design of high-affinity inhibitors of poly-ADP-ribose polymerase-1 (PARP-1) is at the heart of modern anti-cancer drug design. While relevance of enzyme to DNA repair processes in cellular environment is firmly established, the structural and functional understanding of the main determinants for high-affinity ligands controlling PARP-1 activity is still lacking. The conserved active site of PARP-1 represents an ideal target for inhibitors and may offer a novel target at the treatment of breast cancer. To fill the gap in the structural knowledge, we report on the combination of molecular dynamics (MD) simulations, principal component analysis (PCA), and conformational analysis that analyzes in great details novel binding mode for a number of inhibitors at the PARP-1. While optimization of the binding affinity for original target is an important goal in the drug design, many of the promising molecules for treatment of the breast cancer are plagued by significant cardiotoxicity. One of the most common side-effects reported for a number of polymerase inhibitors is its off-target interactions with cardiac ion channels and hERG1 channel, in particular. Thus, selected candidate PARP-1 inhibitors were also screened in silico at the central cavities of hERG1 potassium ion channel.
Introduction
Poly [ADP-ribose] polymerase 1 (PARP-1) is an archetypical enzyme from the PARP group, which contains 18 identified proteins in humansCitation1. PARP-1 catalyzes the polymerization of ADP-ribose units from NAD+ co-enzymes (as donor group) on target proteinsCitation2. An entire family shares well-conserved catalytic domain with “PARP signature” motif. PARP-1 structural and functional organization is also well preserved with four major domains: (i) zinc-finger DNA-binding domain (DBD), (ii) caspase-cleaved domain, (iii) auto-modification domain, and (iv) a C-terminal catalytic domain containing an active site for the enzymeCitation3,Citation4. Because of its significance in control of DNA repair, PARP family has been considered as an important target for anti-cancer therapeuticsCitation5. It was suggested that a number of PARP-1 inhibitors could be effective against tumors with inherent DNA repair defects, such as common breast tumors with mutations in the DNA repair proteins BRCA1 or BRCA2. PARP inhibitors also showed considerable promises as part of therapeutic intervention in several other diseases, such as stroke, cardiac ischemia, cancer, inflammation, and diabetesCitation6–9. PARP inhibitors can be used as anti-cancer agents if a genetic treatment is considered; indeed they are already started to use for the treatment of triple-negative breast cancerCitation10. PARP catalyzes the cleavage of NAD+ into ADP and ADP-ribose. Expectably, most of the developed inhibitors resemble the nicotinamide moiety of NAD+ to achieve high-affinity binding to the corresponding catalytic pocket in PARP-1Citation10. The available X-ray data involving the catalytic domain of PARP-1 with novel bound inhibitors at the binding site leads virtual screening studies on PARP-1 inhibitorsCitation11.
Here molecular dynamics (MD) simulations are performed for better understanding of the inhibitor interactions with PARP-1 enzyme. All-atom MD simulations were then used for elucidation of inhibitor and enzyme dynamics. In the present work, structural and dynamical profiles of both target and inhibitors are thoroughly analyzed with derived MD trajectories. The functionally important transitions and structural features were defined with principle component analysis (PCA) and clustering analysis of MD trajectories. The data from MD simulations lead us an opportunity to explore the intermolecular ligand/protein interactions at atomic scale. In particular, MD simulations enabled comparison of the structural and dynamical re-arrangements between holo and apo states of PARP-1. A set of known drugs on the market is then tested to map their multi-functional potentials as potent PARP-1 inhibitors. The well-known feature of many polymerase inhibitors is their high cardiotoxicity due to blockade of hERG1 channel. It is well established that human ether-à-go-go related gene 1 (hERG1) K channel is overexpressed in many tumors and, therefore, pro-arrhythmic potential of all novel inhibitors is an important concern in structure driven drug development. To circumvent potential risks associated with drug-induced QT prolongation, we report on an in silico cardiotoxicity screening against previously developed hERG1 modelsCitation12–19 by our group.
Methodology
Input receptor structure
Crystal structure of PARP-1 (Protein Data Bank (PDB) code: 4HHY)Citation11 which involves a bound-novel inhibitor inside the binding site was taken from the X-ray structure (). Hydrogen atoms were added to the receptor by employing the Protein Preparation WizardCitation20 integrated in the Maestro molecular modeling suite of Schrodinger software package (Tripos, St. Louis, MO). The PROPKACitation21 program was used to assign the protonation states of amino acids as in physiological medium, i.e. the protonation and tautomeric states of Asp, Glu, Arg, Lys, and His residues were assigned at the pH of 7.4. The hydrogen atoms were then relaxed with a restrained minimization procedure.
Simulation protocol
The protein–ligand complex was simulated for 25 ns in 0.15 M KCl solution and in the presence of explicit water molecules. The TIP3P water model was employed. The aqueous medium was modeled by Visual Molecular Dynamics (VMD)Citation22 program. MD simulations were carried out using all-atom CHARMM36 force-field parameters with CMAP correctionsCitation23 implemented in NAMD 2.9 program package (Theoretical Biophysics Group, Baltimore, MD)Citation24. All bonds involving hydrogen atoms were restrained using SETTLECitation25 algorithm. The Langevin piston method with Langevin thermostat was used to maintain constant temperature (310 K) and constant pressure (1 atm) during simulations. Periodic boundary conditions (PBC) and particle mesh Ewald (PME) methods were used to ensure the correct treatment of long-range electrostatic interactions. The non-bonded cut-off was set as 10 Å for inter-atomic Lennard–Jones (LJ) and Coulombic interactions. Water molecules around protein were equilibrated for 1 ns by keeping the protein atoms at their fixed positions then the constraints on the atomic positions of protein atoms were reduced gradually. The MD trajectories were analyzed utilizing VMD, PyMOLCitation26, Bio3DCitation27, and R-based scripts. The snapshot picture of the simulation box at the end of the equilibration is given in .
Principal component analysis
Principal component analysis (PCA)Citation28 is a statistical approach to identify stable basins in high-dimensional data allowing for reducing dimensionality of the process. In order to implement PCA in MD simulations, 2000 trajectory frames derived throughout the MD simulations are incorporated into the Bio3D scripts. A binary MD trajectory file includes coordination data of protein atoms (Cα atoms used in this study). The coordinates of atoms were written in the column of matrix and they are aligned with respect to the initial conformer to remove the effect of diffusion of atoms during MD simulations in PCA.
Mathematical calculations of PCs from MD trajectories include two consequence steps:
(i) generation of the covariance matrix (C),
(1)
(ii) the next stage is diagonalization of the 3N × 3N covariance matrix C that can be applied by eigenvalue decomposition (EVD) as
(2)
In order to identify the principal dynamics of the MD simulations and separate it from the unrelated noise, we applied a PCA for the MD frames of the two systems by diagonalizing the positional fluctuations of covariance matrix (S) of the Cα atoms relative to average structure of a given conformation. We also performed dynamic cross-correlation maps (DCC) to show dynamic cross-correlated motions of Cα atoms in the trajectory re-oriented relative to an average structure.
MD simulations of biomolecules result in a collection of noisy states, which poses a considerable challenge in finding principles governing ligand binding to the receptor. A PCA can reduce complexity of the protein dynamics by mapping correlations existing in the trajectory.
Per-residue interaction energy calculations, and hydrogen bonding network
Per-residue interaction energy calculations, contact maps, and hydrogen bonding analyses were performed with the available plugin in VMD. The per-residue contributions to protein–ligand interaction energy were evaluated using a cut-off distance of 12 Å. The following criteria were used for the evaluation of hydrogen bonding: the angle cut-off values were <20° and the heavy atom–light atom distance cut-off was 3.0 Å.
Molecular docking simulations
A set of approved drugs was retrieved from Drug Bank databaseCitation29. The lowest energy conformers of the ligands were searched using LigPrep moduleCitation30 of Maestro SuiteCitation31. The ionization states and the partial charges of the ligand atoms were assigned according to the pH of 7.4. Molecular docking studies were performed with the Glide dockingCitation32 program. Glide has three scoring functions: extra-precision (XP), standard precision (SP), and high-throughput virtual screening (HTVS). In this study, initially the SP method was performed for large data set of inhibitors and top-docking poses were selected and re-docked using the XP method. The volume of the inner and outer active binding-site of PARP1 target was defined as (14 × 14 × 14) Å3 and (30 × 30 × 30) Å3, respectively. The active site was defined by the centroid rendered from inhibitor observed in crystallographic structure. For top-10 docking poses generated by Glide/XP, a multi-stage induced fit docking (IFD) method was also performedCitation33. For IFD, the van der Waals radius rendering for the complex was set by a factor of 0.5 and ligands were docked with the default Glide/SP. Then, PrimeCitation34 protocol was used to refine positioning of the protein side chains. Finally, the ligand poses were scored and top-poses were re-docked using standard Glide/XP without any scaling applied to van der Waals radius.
Results and discussion
In this study, a combined approach involving docking simulations, MD simulations, and PCA was applied to identify the key structural features governing effective inhibition of PARP-1. MD simulations performed on two different conformational states of PARP-1, named as apo and holo, were also used to compare state-dependent inhibitory mechanism. At the end of 25 ns MD simulations, the total energy, temperature, and volume fluctuations of the system reached to a plateau indicating the well-equilibrated system. compares the equilibrium structure obtained from MD simulations with the X-ray structure of PARP-1. The bound inhibitor displays very little relative displacement in the course of MD simulations relative to its position in the crystal structure ().
Figure 2. (a) The crystal structure of the PARP-1 complex (green) superimposed with the representative structure (red). (b) Equilibrated inhibitor pose and its interactions with active site residues.

Ligand interaction diagram was used to show 2D receptor–ligand interactions in a representative MD trajectory (). Five stable hydrogen-bonding interactions which were reported to be essential for drug stabilization in PARP-1-ligand complexes were reproduced in our simulations. These H-bonds are (i) between the inhibitor carbonyl's oxygen and the OH group of Ser904 amino acid; (ii) between the nitrogen atom in the ring of the ligand and backbone oxygen of the Gly863; (iii) between the amide backbone of Gly863 and carbonyl oxygen of the ligand; (iv) between the amide backbone of Ile879 and carbonyl oxygen of the ligand; (v) between carbonyl oxygen of the ligand and backbone OH group of the Arg878. Additionally, the number of hydrophobic interactions between the inhibitor and protein residues (i.e. Phe897, Trp861, Tyr907, Ala880, Ile872, and Ile895) are available.
Figure 3. Mainly observed conformations of inhibitor at the binding pocket throughout 25 ns MD simulations. 2D ligand interaction diagram for the representative complex structure has also been shown in the figure.

Clustering analysis
Clustering analysis in PARP-1–ligand complex allowed us to identify the stable binding poses of the ligand. The inhibitor in the active site cavity has a dominant conformation which comprises the 99% of frames in analyzed trajectories as shown in .
System stability analysis
The RMSD values for Cα atoms can serve as a measure for relative flexibility of the binding pockets in apo and holo states of PARP-1 (). To illuminate the effect of a given conformation on the stability of binding pocket, we calculated the RMSD values with respect to Cα atoms of the residues comprising binding site and participating in hydrogen bonding () using the protein X-ray diffraction structure. Two independent simulations with and without bound inhibitors, i.e. at the holo and apo states, were carried out to determine the effect of inhibitor on the protein dynamics. The RMSD values for Cα atoms of residues forming binding pocket showed a slightly higher RMSD in the apo state than in the holo state at the equilibration stage of the MD simulations. After the equilibration, the PARP-1 structure at both apo and holo forms did not display any significant conformational transitions (). The RMSD values for all Cα atoms of the PARP-1 protein at apo and holo states fluctuated around a mean value (1.83 Å and 1.65 Å for holo and apo forms, respectively) and remained almost the same during the equilibration ().
Figure 4. (a) RMSD versus time plot for all Cα atoms at the target; (b) RMSD versus time plot for Cα atoms of residues that are participating in hydrogen bonding with the inhibitor.
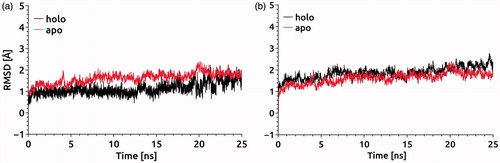
illustrates per-residue RMSF values for Cα atoms of the protein. The Cα atoms of the residues which involve in loops have higher flexibility than the ones in the α-helices and β-sheets at both apo and holo forms. The incorporation of the inhibitor induces some conformational changes as reflected in the regions formed by residues from 696 to 713 and also from 881 to 888. The RMSF plot confirms the experimentally observed H-bond interaction between the amino acids, Ser904, Gly863, and inhibitor at the active binding site at both apo and holo proteins. As expected, the apo form is more flexible than the holo form, inhibitor-bearing structure ().
Per-residue interaction energy analysis
The quantitative analysis of the interactions between the inhibitor and the amino acids of the residues in the binding pocket was done by calculating the interaction energies as an average of 25 ns MD simulations time. The interacting groups are categorized according to their position with respect to the inhibitor molecule. For better visualization, the structure of the inhibitor is divided into three segments and the interactions of the each segment with the specific amino acids, and their binding energies are shown in . The total non-bonded interaction energies including the H-bond of the first, second, and third segments are found to be −24, −14, and −16 kcal/mole, respectively. The first segment interacts via strong hydrophobic and weak π–π stacking interactions with Tyr907 and Tyr896, via H-bonding interactions with Ser904 and Gly863. The chemical structure of the first segment resembles the active pocket of nicotinamide adenine dinucleotide (NAD+) for which the strong H-bond interaction is reportedCitation35,Citation36. The non-bonded interactions are mostly van der Waals type and relatively stronger interactions involve His862, Tyr907, Tyr896, and Arg878 amino acids with the energies varying between −4 and −9 kcal/mole. This computational result is in a good agreement with the experimental finding which pointed out that His862 and Glu988 have significant contributions to the interactions at the active siteCitation35,Citation36. Among the PARP-1 active site amino acids, Glu988 is important for NAD+ catalysisCitation37,Citation38, Lys903 is critical for the DNA polymerization processCitation39. The second part of the inhibitor molecule forms strong hydrophobic interaction with His862 of beta sheet-like Glu988, it also participates in NAD+ bindingCitation40. The third segment of the inhibitor interacts with Ala880, Leu769, Ile879, Pro881, and Arg878 which plays a critical role in stabilizing the adenosine portion of the substrate NAD+Citation41.
Figure 6. The average ligand–target interaction energies between the given residues and the inhibitor.
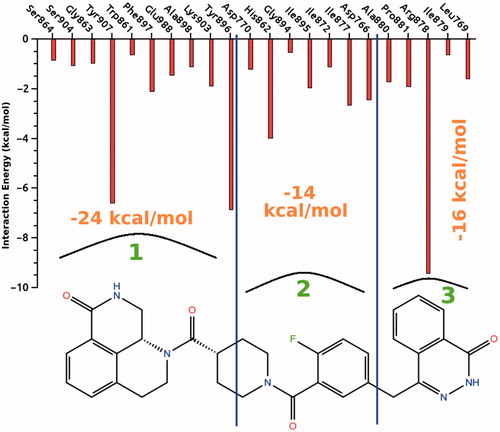
H-bond formation ratios during the MD simulations for the key-amino acids have been represented in Figure S1 in the Supplementary materials. The percentage of hydrogen bond occupancies of the key-amino acids during 25 ns MD simulations has been found as Gly863 (52%), Ser904 (33%), Arg878 (40%), and Ile879 (30%) (Figure S1, Supplementary materials).
PCA
Eigenvalues defining collective modes of fluctuations in apo and holo states of PARP-1 protein are shown in . Residue cross-correlation calculations allowed us to examine the effect of ligand on the conformational dynamics of the protein. It is clear from these calculations that inhibitor binding has an impact on a low-frequency harmonic mode propagating along an entire PARP-1 structure. illustrates positive (moving of amino acid residues in the same directions) and negative (moving of amino acid residues in opposite directions with respect to each others) correlations for studied systems. Positive and negative correlations between amino acid residues conclusively determine the conformational transition pathway during inhibitory treatments of protein. Higher negative correlations were significantly established between PARP-1 amino acids in the presence of inhibitor, the corresponded domains and residues have been indicated in correlation profile.
Protein conformation clustering
To elucidate the effects of drug binding on conformational dynamics of the protein, we employed RMS-based clustering analysis using Cα positionsCitation42. Reduction of the protein dynamics to Cα dynamics allows us to decrease the dimensionality of the system of interest by eliminating fast side-chain dynamics. shows the PC profile and displays the relation of variance versus applied eigenvalue mode at apo and holo states. The first four modes, i.e. PC1, PC2, PC3, and PC4, combine 43.0% and 55.1% of the variance for apo and holo forms, respectively. Unlike to the apo state which includes low occupancy for the PC1 (17.5%), the holo form of the first PC indicates a high-degree linear conformational transition with respect to the inhibitor (37.3% of the whole conformational transition). Highly fluctuated regions during MD simulations are analyzed using PCA and represented in Figure S2 in the Supplementary materials.
Molecular docking
Database mining allows rapid and exhaustive search of potential candidates for further biological testing. To improve its performance on the rational design of drug candidates, it can be matched to an array of De Novo drug design algorithmsCitation43. To initiate database development, we focus on an array of small ligands with known PARP-1 inhibition properties to create our initial hypothesis. The available drug-bank databases are analyzed and ∼9000 small molecules are considered as potential inhibitor molecules of PARP-1 target. Their properties such as molecular weight, ALogP, hydrogen bond numbers as acceptors and donors, molar refractivity, number of rotatable bonds, and polar surface area were used to filter out the ligands with a low degree of drug-likenessCitation44. The remaining compounds are then subjected to Glide/SP docking protocol. Docking scores of compounds which are better than −8 kcal/mole were selected as potential PARP-1 inhibitors. The Glide/XP and Glide/IFD protocols were then employed for the selected ligands as given in Figure S3 in the Supplementary materials.
In addition to test the validation of used docking protocols in examining the PARP-1 inhibitory activity of compounds, several known active and inactive PARP-1 inhibitors were selected and docked into the binding pocket of the target structure (). Each compound was identified as its ZINC database ID, experimental binding affinities (IC50)Citation45–52, and estimated docking scores were reported for individual compounds. While docking results showed higher (absolute) docking scores for PARP-1 active inhibitors, inactive PARP-1 molecules showed lower docking scores (). These results significantly suggest the usefulness of low time-consuming in silico methods in discovering of novel potent inhibitors.
Table 1. Experimental binding affinities and estimated docking scores (Glide/XP) of active and inactive PARP-1 inhibitors at interaction with PARP-1 target. Each compound is identified by its ZINC database ID.
MD simulationsCitation53 and SAR studiesCitation54 on PARP-1 inhibitor complexes revealed that the potent PARP-1 inhibition depends on the position of the carboxamide oxygen. This functional group is stabilized by hydrogen bonds that it forms with Ser904 and Gly863 in the PARP-1 NAD+ binding site. According to our docking results, the residues in the ligand binding-site included Ser904, Gly863, and also Arg865, Glu988, Tyr907, and Ser864.
Selected PARP-1 potent inhibitors were also tested in silico against their cardiotoxicity risks. They were docked into the central cavities of previously generated and validated human ether-a go-go related gene (hERG1) K-channel models by our groupCitation12–19. shows the Glide/XP docking scores of these drugs in hERG1. shows top-docking pose of carbergoline which has the lowest docking score at the pore domains of hERG1 K channel. Our results are in agreement with the experimental studies which indicate that cabergoline is a prolactinemic drug with low toxicity. It is an anticancer drug and controls the prolactin release in metastatic breast cancerCitation55,Citation56. The second identified anti-cancer drug is raloxifene. According to in vitro studies, raloxifene decreases and prevents breast cancer disease in womenCitation57. Idarubicin drug is characterized with a high affinity against PARP-1 enzyme and low hERG blocking properties. Idarubicin is a member of a generic group of anticancer drugs named as anthracycline antibioticsCitation58.
Figure 9. 2D ligand interaction diagram of cabergoline with the hERG pore domain and its 3D top-docking pose of at hERG1 K channel.

Table 2. Docking scores of selected 12 potent drugs against hERG1 channel pore domain (kcal/mol).
The selected molecules which show high docking scores at the active site of the PARP-1 target are re-screened at the hERG1 pore domain for checking in silico their cardiotoxicity risks. Molecules which show low predicted hERG1-blocking affinities at the central cavities of the channel can be considered as safer drugs.
Conclusions
In this study, we have combined molecular docking, MD simulations, and binding energy analysis on both holo and apo forms of PARP-1 target to obtain some insights into the conformations and interactions for binding of PARP-1 inhibitors. Our work indicated that the residues Ser904, Gly863, and Arg878 forming hydrogen bond with the ligand are highly responsible for the stability of the binding pocket. Especially, Ser904 and Gly863 are primarily corresponded for establishing the inhibitory potency of PARP-1 inhibitors. PC analysis merged with clustering to elucidate conformational transitions in the presence of PARP-1 inhibitor. PCA analysis showed that ligand binding to PARP-1 hinders protein dynamics compared with the ligand-free state. We also screened in silico a set of known compounds as PARP-1 inhibitor candidates both in principal target and against common off-target (central cavities of hERG1 K channel).
Supplementary material available online
Supplementary Figures S1-S3.
IENZ_1005011_SupplementaryMaterial.pdf
Download PDF (492.3 KB)Acknowledgements
Part of computations for the work described in this paper was supported by Turkish Scientific and Technical Research Council (TUBITAK) ULAKBIM High Performance Computing Center. S.D. acknowledges support from Bilim Akademisi – The Science Academy, Turkey, under the BAGEP program.
Declaration of interest
The authors report no conflicts of interest. The authors alone are responsible for the content and writing of this article.
References
- Amé JC, Spenlehauer C, De Murcia G. The PARP superfamily. BioEssays 2004;26:882–93
- Kim MY, Zhang T, Kraus WL. Poly(ADP-ribosyl)ation by PARP-1: “PAR-laying” NAD+ into a nuclear signal. Genes Dev 2005;19:1951–67
- D’Amours D, Desnoyers S, D’Silva I, Poirier GG. Poly(ADP-ribosyl)ation reactions in the regulation of nuclear functions. Biochem J 1999;342:249–68
- Kraus WL, Lis JT. PARP goes transcription. Cell 2003;113:677–83
- Griffin RJ, Curtin NJ, Newell DR, et al. The role of inhibitors of poly(ADP-ribose) polymerase as resistance-modifying agents in cancer therapy. Biochimie 1995;77:408–22
- Bai P, Cantó C. The role of PARP-1 and PARP-2 enzymes in metabolic regulation and disease. Cell Metab 2012;16:290–5
- Gibson B, Kraus WL. New insights into the molecular and cellular functions of poly(ADP-ribose) and PARPs. Nat Rev Mol cell Biol 2012;13:411–24
- Soldani C, Scovassi AI. Poly(ADP-ribose) polymerase-1 cleavage during apoptosis: an update. Apoptosis 2002;7:321–8
- De la Lastra CA, Villegas I, Sánchez-Fidalgo S. Poly(ADP-ribose) polymerase inhibitors: new pharmacological functions and potential clinical implications. Curr Pharm Des 2007;13:933–62
- Rios J, Puhalla S. PARP inhibitors in breast cancer: BRCA and beyond. Oncology (Williston Park) 2011;25:1014–25
- Ye N, Chen CH, Chen T, et al. Design, synthesis, and biological evaluation of a series of benzo[de][1,7]naphthyridin-7(8 H)-ones bearing a functionalized longer chain appendage as novel PARP1 inhibitors. J Med Chem 2013;56:2885–903
- Durdagi S, Deshpande S, Duff HJ, Noskov SY. Modeling of open, closed, and open-inactivated states of the hERG1 channel: structural mechanisms of the state-dependent drug binding. J Chem Inf Model 2012;52:2760–74
- Subbotina J, Yarov-Yarovoy V, Lees-Miller J, et al. Structural refinement of the hERG1 pore and voltage-sensing domains with ROSETTA-membrane and molecular dynamics simulations. Proteins Struct Funct Bioinf 2010;78:2922–34
- Mavromoustakos T, Durdagi S, Koukoulitsa C, et al. Strategies in the rational drug design. Curr Med Chem 2011;18:2517–30
- Durdagi S, Subbotina J, Lees-Miller J, et al. Insights into the molecular mechanism of hERG1 channel activation and blockade by drugs. Curr Med Chem 2010;17:3514–32
- Durdagi S, Zhao C, Cuervo JE, Noskov SY. Atomistic models for free energy evaluation of drug binding to membrane proteins. Curr Med Chem 2011;18:2601–11
- Durdagi S, Guo J, Lees-Miller JP, et al. Structure-guided topographic mapping and mutagenesis to elucidate binding sites for the human ether-a-go-go-related gene 1 potassium channel (KCNH2) activator NS1643. J Pharmacol Exp Ther 2012;342:441–52
- Buturak B, Durdagi S, Noskov SY, Ildeniz, TO. Designing of multi-targeted molecules using combination of molecular screening and in silico drug cardiotoxicity prediction approaches. J Mol Graph Model 2014;50:16–34
- Guo J, Durdagi S, Changalov M, et al. Structure driven design of novel human ether-a-go-go-related-gene channel (hERG1) activators. PLoS One 2014;9:e105553
- Madhavi Sastry G, Adzhigirey M, Day T, et al. Protein and ligand preparation: parameters, protocols, and influence on virtual screening enrichments. J Comput Aided Mol Des 2013;27:221–34
- Li H, Robertson AD, Jensen JH. Very fast empirical prediction and rationalization of protein pK a values. Proteins Struct Funct Genet 2005;61:704–21
- Humphrey W, Dalke A, Schulten K. VMD: Visual molecular dynamics. J Mol Graph 1996;14:33–8
- Guvench O, Mallajosyula SS, Raman EP, et al. CHARMM additive all-atom force field for carbohydrate derivatives and its utility in polysaccharide and carbohydrate–protein modeling. J Chem Theory Comput 2011;7:3162–80
- Nelson MT, Humphrey W, Gursoy A, et al. NAMD: a parallel, object oriented molecular dynamics program. Int J Supercomput Appl High Perform Comput 1996;10:251–68
- Miyamoto S, Kollman PA. SETTLE: an analytical version of the SHAKE and RATTLE algorithm for rigid water models. J Comput Chem 1992;13:952–62
- Rother K. Introduction to PyMOL. Methods Mol Biol Clift NJ 2005;635:0–32
- Grant BJ, Rodrigues APC, ElSawy KM, et al. Bio3d: an R package for the comparative analysis of protein structures. Bioinformatics 2006;22:2695–6
- Pearson K. LIII. On lines and planes of closest fit to systems of points in space. London, Edinburgh, Dublin Philos Mag J Sci 1901;2:559–72
- Wishart DS. DrugBank and its relevance to pharmacogenomics. Pharmacogenomics 2008;9:1155–62
- Chen IJ, Foloppe N. Drug-like bioactive structures and conformational coverage with the ligprep/confgen suite: comparison to programs MOE and catalyst. J Chem Inf Model 2010;50:822–39
- Maestro, Version 9.2. New York: Schrödinger, LLC; 2011
- Halgren TA, Murphy RB, Friesner RA, et al. Glide: a new approach for rapid, accurate docking and scoring. 2. Enrichment factors in database screening. J Med Chem 2004;47:1750–9
- Farid R, Day T, Friesner RA, Pearlstein RA. New insights about HERG blockade obtained from protein modeling, potential energy mapping, and docking studies. Bioorganic Med Chem 2006;14:3160–73
- Jacobson MP, Pincus DL, Rapp CS, et al. A hierarchical approach to all-atom protein loop prediction. Proteins Struct Funct Genet 2004;55:351–67
- Chen J, Peng H, He J, et al. Synthesis of isoquinolinone-based tricycles as novel poly(ADP-ribose) polymerase-1 (PARP-1) inhibitors. Bioorganic Med Chem Lett 2014;24:2669–73
- Ferraris DV. Evolution of poly(ADP-ribose) polymerase-1 (PARP-1) inhibitors: from concept to clinic. J Med Chem 2010;31:4561–84
- Morrison AR, Moss J, Stevens LA, et al. ART2, a T cell surface mono-ADP-ribosyltransferase, generates extracellular poly(ADP-ribose). J Biol Chem 2006;281:33363–72
- Hara N, Tsuchiya M, Shimoyama M. Glutamic acid 207 in rodent T-cell RT6 antigens is essential for arginine-specific ADP-ribosylation. J Biol Chem 1996;271:29552–5
- Ruf A, Rolli V, de Murcia G, Schulz GE. The mechanism of the elongation and branching reaction of poly(ADP-ribose) polymerase as derived from crystal structures and mutagenesis. J Mol Biol 1998;278:57–65
- Ruf A, Mennissier de Murcia J, de Murcia G, Schulz GE. Structure of the catalytic fragment of poly(AD-ribose) polymerase from chicken. Proc Natl Acad Sci USA 1996;93:7481–5
- Hannigan K, Kulkarni SS, Bdzhola VG, et al. Identification of novel PARP-1 inhibitors by structure-based virtual screening. Bioorganic Med Chem Lett 2013;23:5790–4
- Shenkin PS, Mcdonald DQ. Cluster analysis of molecular conformations. J Comput Chem 1994;15:899–916
- Mauser H, Guba W. Recent developments in de novo design and scaffold hopping. Curr Opin Drug Discov Devel 2008;11:365–74
- Lipinski CA, Lombardo F, Dominy BW, Feeney PJ. Experimental and computational approaches to estimate solubility and permeability in drug discovery and development settings. Adv Drug Deliv Rev 2012;64:4–17
- Costantino G, Macchiarulo A, Camaioni E, Pellicciari R. Modeling of poly(ADP-ribose)polymerase (PARP) inhibitors. Docking of ligands and quantitative structure-activity relationship analysis. J Med Chem 2001;44:3786–94
- Li JH, Serdyuk L, Ferraris DV, et al. Synthesis of substituted 5[H]phenanthridin-6-ones as potent poly(ADP-ribose)polymerase-1 (PARP1) inhibitors. Bioorganic Med Chem Lett 2001;11:1687–90
- Steinhagen H, Gerisch M, Mittendorf J, et al. Substituted uracil derivatives as potent inhibitors of poly(ADP-ribose)polymerase-1 (PARP-1). Bioorganic Med Chem Lett 2002;12:3187–90
- Lehtiö L, Jemth AS, Collins R, et al. Structural basis for inhibitor specificity in human poly(ADP-ribose) polymerase-3. J Med Chem 2009;52:3108–11
- Jagtap PG, Baloglu E, Southan GJ, et al. Discovery of potent poly(ADP-ribose) polymerase-1 inhibitors from the modification of indeno[1,2-c]isoquinolinone. J Med Chem 2005;48:5100–3
- Jones P, Altamura S, Boueres J, et al. Discovery of 2-{4-[(3S)-piperidin-3-yl]phenyl}-2H-indazole-7-carboxamide (MK-4827): a novel oral poly(ADP-ribose)polymerase (PARP) inhibitor efficacious in BRCA-1 and -2 mutant tumors. J Med Chem 2009;52:7170–85
- Penning TD, Zhu GD, Gandhi VB, et al. Discovery and SAR of 2-(1-propylpiperidin-4-yl)-1H-benzimidazole-4-carboxamide: a potent inhibitor of poly(ADP-ribose) polymerase (PARP) for the treatment of cancer. Bioorganic Med Chem 2008;16:6965–75
- Ferraris D, Ficco RP, Pahutski T, et al. Design and synthesis of poly(ADP-ribose)polymerase-1 (PARP-1) inhibitors. Part 3: in vitro evaluation of 1,3,4,5-tetrahydro-benzo[c][1,6]- and [c][1,7]-naphthyridin-6-ones. Bioorganic Med Chem Lett 2003;13:2513–18
- Ruf A, De Murcia G, Schulz GE. Inhibitor and NAD+ binding to poly(ADP-ribose) polymerase as derived from crystal structures and homology modeling. Biochemistry 1998;37:3893–900
- Suto MJ, Turner WR, Arundel-Suto CM, et al. Dihydroisoquinolinones: the design and synthesis of a new series of potent inhibitors of poly(ADP-ribose) polymerase. Anticancer Drug Des 1991;6:107–17
- Frontini L, Lissoni P, Vaghi M, et al. Enhancement of the efficacy of weekly low-dose taxotere by the long acting anti-prolactinemic drug cabergoline in pretreated metastatic breast cancer. Anticancer Res 2004;24:4223–6
- Howell SJ, Anderson E, Hunter T, et al. Prolactin receptor antagonism reduces the clonogenic capacity of breast cancer cells and potentiates doxorubicin and paclitaxel cytotoxicity. Breast Cancer Res 2008;10:R68
- DeMichele A, Troxel AB, Berlin JA, et al. Impact of raloxifene or tamoxifen use on endometrial cancer risk: a population-based case-control study. J Clin Oncol 2008;26:4151–9
- Lopez M, Di Lauro L, Papaldo P, et al. Phase II trial with oral idarubicin in advanced breast cancer. Invest New Drugs 1986;4:39–42