Abstract
Monoclonal antibodies have significantly advanced our ability to treat cancer, yet clinical studies have shown that many patients do not adequately respond to monospecific therapy. This is in part due to the multifactorial nature of the disease, where tumors rely on multiple and often redundant pathways for proliferation. Bi- or multi- specific antibodies capable of blocking multiple growth and survival pathways at once have a potential to better meet the challenge of blocking cancer growth, and indeed many of them are advancing in clinical development.1 However, bispecific antibodies present significant design challenges mostly due to the increased number of variables to consider. In this perspective we describe an innovative integrated approach to the discovery of bispecific antibodies with optimal molecular properties, such as affinity, avidity, molecular format and stability. This approach combines simulations of potential inhibitors using mechanistic models of the disease-relevant biological system to reveal optimal inhibitor characteristics with antibody engineering techniques that yield manufacturable therapeutics with robust pharmaceutical properties. We illustrate how challenges of meeting the optimal design criteria and chemistry, manufacturing and control concerns can be addressed simultaneously in the context of an accelerated therapeutic design cycle. Finally, to demonstrate how this rational approach can be applied, we present a case study where the insights from mechanistic modeling were used to guide the engineering of an IgG-like bispecific antibody.
Acknowledgments
We thank Lihui Xu, Jason Baum, Kathy Zhang, Yang Jiao, Neeraj Kohli, Steven Su and Rachel Rennard for developing and optimizing the experimental approaches described in this manuscript. We thank Brian Harms, Jeffrey D. Kearns and Bin Li for their valuable insights into computational techniques for modeling antibody based therapeutics. We greatly appreciate critical input on the manuscript from Seth Fidel, Kathleen Petrozzelli, Marco Muda and Stephen Sazinsky. We are indebted to Ulrik Nielsen and Birgit Schoeberl for championing the dual use of antibody engineering and network simulation in early therapeutic design.
Figures and Tables
Figure 1 Optimal design of bispecific antibodies. (A) Iterative simulation-guided therapeutic design cycle. (B) Schematic view of Ig-like bispecific molecule.
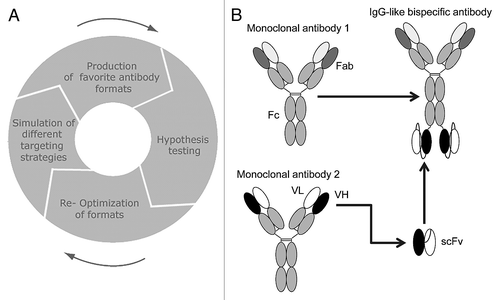
Figure 2 Simulation of the ErbB network predicts design of an optimal ErbB3 therapeutic. (A) Complexity of the ErbB network depicted graphically: ligand binding, receptor dimerization, receptor trafficking and intracellular signaling were captured in a mass-action based kinetic model. (B) Simulated perturbation of each protein in the ErbB network was used to identify the sensitivity of the downstream signal, phospho-Akt, towards each protein under either heregulin or betacellulin stimulation. (C) Dose responsiveness of Akt to an anti-ErbB3 antibody was examined for a variety of affinity binding constants through variation of the dissociation rate. (D) Different strategies to inhibit ErbB3 were examined computationally and experimentally. This figure was originally published by Schoeberl et al.Citation63 and is reprinted with permission.
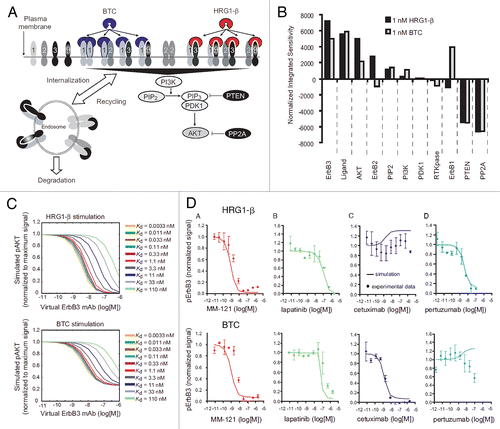
Figure 3 Ability of a bispecific to co-inhibit two pathways is dependent on relative receptor levels. The effect of a bispecific inhibitor on the active level of (A) GFR1, (B) GFR2 and (C) a common downstream signal, Akt, was simulated for cells expressing equal amounts of each target (red), ten times more of GFR1 (blue) and ten times more of GFR2 (green). Due to the lower affinity of the bispecific towards GFR1, potency of GFR1 inhibition decreases when the GFR2 level decreases (left, blue), indicating the effect of avidity. Failure to inhibit GFR1 potently leads to an inability to properly inhibit pAkt (right). The ability to inhibit GFR2 is unaffected by the level of GFR1 due the stronger affinity of the bispecific towards GFR2.
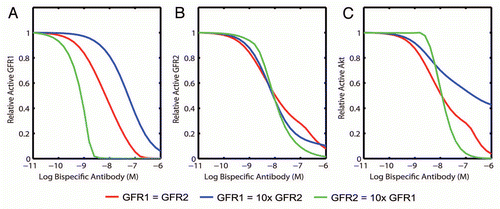
Figure 4 Simulation can be used to identify limitations of potential therapeutics and propose improvement strategies that can broaden clinical utility. A model describing the binding of a ligand-blocking bispecific inhibitor to two target receptors that both activate a common downstream signal (pAkt) was simulated with inhibitor pre-treatment of 1 h and dual ligand stimulation for 15 min. The dose-response of pAkt to the bispecific was investigated over a 1,000-fold range of each target receptor to create an IC50 response surface. (A) Simulation of a bispecific molecule with one binding moiety towards each target. (B) The IC50 response surface was re-simulated with a tetravalent bispecific inhibitor that increases the region of potent inhibition. (C) Simulation of a non-optimal IgG-like bispecific inhibitor. (D) The effect of affinity maturation of the tetravalent bispecific on downstream target inhibition was simulated by decreasing the monovalent dissociation rate towards GFR1 10-fold.
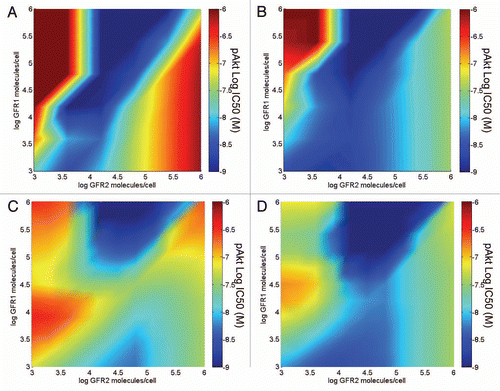
Figure 5 Concurrent optimization of scFv affinity and stability by covalent yeast display. (A) Binding of post thermal challenge isolated yeast displayed scFv modules to soluble GFR2-Fc by fluorescence-activated cell sorting. (B) Thermal challenge assay on optimized scFv modules covalently attached to yeast surface confirms their higher thermal stability. The residual binding activity to GFR2-Fc remaining after heat stress for 5 min at 65°C was measured by fluorescence-activated cell sorting.
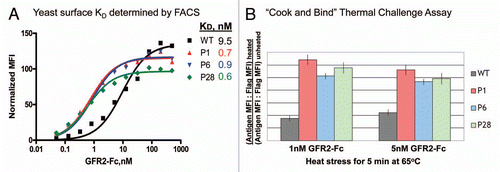
Figure 6 Comparative analysis of the properties of proof-of-concept and optimized bispecific antibodies. (A and B) Micro-scale triage composed of differential scanning fluorescence profiling and thermal inactivation assays allow the selection of bispecific with improved serum and aggregation stabilities. The proof-of-concept bispecific molecule is inferior to a stabilized one in both microscopic (measured by differential scanning fluorimetry (A)) and macroscopic properties [measured by percent of binding activity remaining after 3 day incubation in mouse serum at 37°C (B)]; (C) Optimized bispecific antibody display stronger cellular binding compared to proof-of-concept one. The binding to cancer cell line expressing both GFR1 and GFR2 was measured by fluorescence-activated cell sorting.
![Figure 6 Comparative analysis of the properties of proof-of-concept and optimized bispecific antibodies. (A and B) Micro-scale triage composed of differential scanning fluorescence profiling and thermal inactivation assays allow the selection of bispecific with improved serum and aggregation stabilities. The proof-of-concept bispecific molecule is inferior to a stabilized one in both microscopic (measured by differential scanning fluorimetry (A)) and macroscopic properties [measured by percent of binding activity remaining after 3 day incubation in mouse serum at 37°C (B)]; (C) Optimized bispecific antibody display stronger cellular binding compared to proof-of-concept one. The binding to cancer cell line expressing both GFR1 and GFR2 was measured by fluorescence-activated cell sorting.](/cms/asset/9fa80748-afb2-4394-8c6a-1936674cb4b3/kmab_a_10915299_f0006.gif)
Figure 7 A workflow for rational engineering of a bispecific antibody. The engineering path is separated in three major stages. At the proof-of-concept stage, we focus on identifying optimal design criteria and create 1–2 molecules that trigger the desired biological responses. At the therapeutic design stage, we pursue parallel optimization of the modules of the bispecific molecule. We assemble the antibody optimized modules into bispecific molecules and evaluate them relative to the optimal design criteria. At the candidate selection stage, the properties of the lead and differentiated back-ups are selected through normal scale assays.
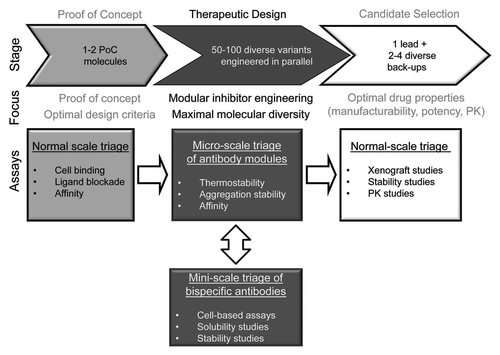