ABSTRACT
This paper describes a novel method of training a semantic segmentation model for scene recognition of agricultural mobile robots exploiting multiple publicly available datasets that are different from the target greenhouse environments. Semantic segmentation models require abundant labels given by tedious manual annotation for training. Although unsupervised domain adaptation (UDA) is studied as a workaround for such a problem, existing UDA methods assume a source dataset similar to the target dataset, which is not available for greenhouse scenes. In this paper, we propose a method to train a semantic segmentation model for greenhouse images leveraging multiple publicly available datasets not dedicated to greenhouses. We exploit segmentation models pre-trained on each source dataset to generate pseudo-labels for the target images based on agreement of all the pre-trained models on each pixel. The proposed method allows for effectively transferring the knowledge from multiple sources rather than relying on a single dataset and realizes precise training of semantic segmentation model. We also introduce existing state-of-the-art methods to suppress the effect of noise in the pseudo-labels to further improve the performance. We demonstrate that our proposed method outperforms existing UDA methods and a supervised SVM-based method.
GRAPHICAL ABSTRACT
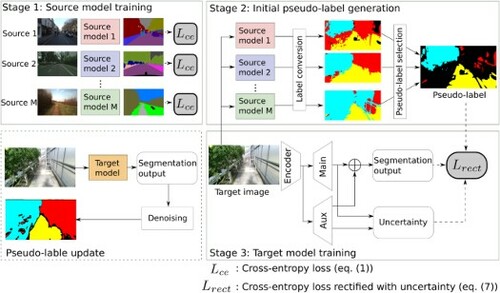
Disclosure statement
No potential conflict of interest was reported by the author(s).
Additional information
Notes on contributors
Shigemichi Matsuzaki
Shigemichi Matsuzaki received the associate degree in engineering from National College of Technology, Kumamoto College in 2016, and the bachelor's and the master's degrees in engineering from Toyohashi University of Technology, Aichi, Japan in 2018 and 2020, respectively. He is currently pursuing the PhD degree at Toyohashi University of Technology. His research interests include deep learning in robot perception, mobile robots in unstructured environments, and mobile service robots.
Jun Miura
Jun Miura received the BEng degree in mechanical engineering, and the MEng and DrEng degrees in information engineering from The University of Tokyo, Tokyo, Japan, in 1984, 1986, and 1989, respectively. In 1989, he joined the Department of Computer-Controlled Mechanical Systems, Osaka University, Suita, Japan. Since April 2007, he has been a Professor with the Department of Computer Science and Engineering, Toyohashi University of Technology, Toyohashi, Japan. From March 1994 to February 1995, he was a Visiting Scientist with the Computer Science Department, Carnegie Mellon University, Pittsburgh, PA. He has published over 240 articles in international journal and conferences in the areas of intelligent robotics, mobile service robots, robot vision, and artificial intelligence. He received several awards, including the Best Paper Award from the Robotics Society of Japan, in 1997, the Best Paper Award Finalist at ICRA-1995, and the Best Service Robotics Paper Award Finalist at ICRA-2013
Hiroaki Masuzawa
Hiroaki Masuzawa received the associate degree in engineering from National College of Technology, Hachinohe College in 2006, received the bachelor's and the master's degree in engineering from Toyohashi University, Aichi, Japan in 2008 and 2010, respectively. He is currently a project research associate of Department of Computer Science and Engineering, Toyohashi University of Technology. His research interests include application of artificial intelligence to agricultural robots.