Figures & data
Fig. 1 Plot of FPR against normalized effect size, with power kept constant throughout the curves by varying n. The dashed blue line shows the FPR calculated by the p-less-than method. The solid blue line shows the FPR calculated by the p-equals method. This example is calculated for an observed p-value of 0.05, with power kept constant at 0.78 (power calculated conventionally at p = 0.05), with prior probability P(H1) = 0.5. The sample size needed to keep the power constant at 0.78 varies from n = 1495 at effect size = 0.1, to n = 5 at effect size = 2.0 (the range of plotted values). The dotted red line marks an FPR of 0.05, the same as the observed p-value. Calculated with Plot-FPR-v-ES-constant-power.R, output file: FPR-vs-ES-const-power.txt (supplementary material).
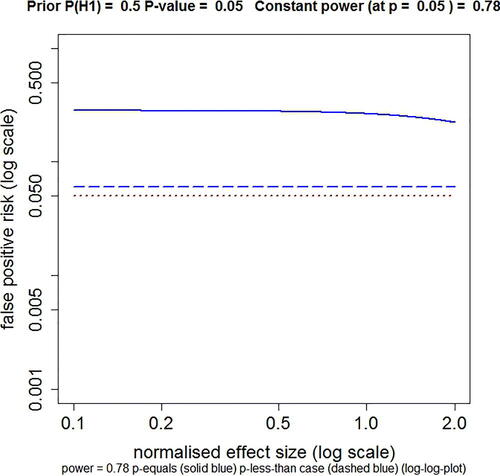
Fig. 2 FPR plotted against n, the number of observations per group for a two independent sample t-test, with normalized true effect size of 1 standard deviation. The FPR is calculated by the p-equals method, with a prior probability P(H1) = 0.5 (EquationEquation A6(A6)
(A6) ). Log–log plot. Calculations for four different observed p-values, from top to bottom these are: p = 0.05 (blue), p = 0.01 (green), p = 0.001 (red), p = 0.0001 (orange). The power of the tests varies throughout the curves. For example, for the p = 0.05 curve, the power is 0.22 for n = 4 and power is 0.9999 for n = 64 (the extremes of the plotted range). The minimum FPR (marked with gray-dashed lines) is 0.206 at n = 8. This may be compared with the FPR of 0.27 at n = 16, at which point the power is 0.78 (as in Colquhoun, (Citation2014), (2017)). Values for other curves are given in the print file for these plots (Calculated with Plot-FPR-vs-n.R. Output file: Plot-FPR-vs-n.txt. supplementary material).
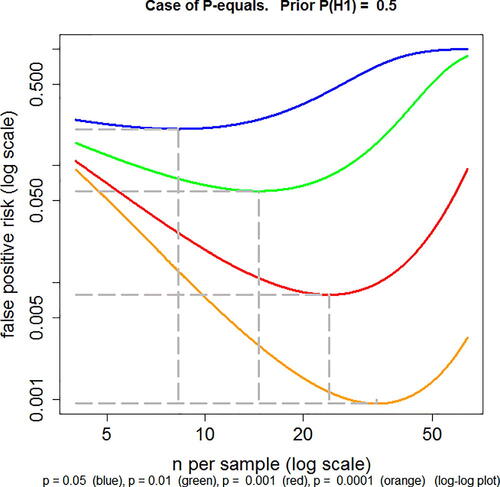
Table 1 Calculations done with web calculator http://fpr-calc.ucl.ac.uk/, or with R scripts (see the appendix).
Fig. 3 Comparison of three approaches to calculation of FPR in the case of a simple alternative hypothesis. Solid blue line: the p-equals method for t-tests described in Colquhoun (Citation2017), and EquationEquations (A5)(A5)
(A5) and Equation(A6)
(A6)
(A6) . Dashed blue line: the Sellke–Berger approach, using EquationEquations (A8)
(A8)
(A8) and Equation(A6)
(A6)
(A6) . Dotted blue line: the Goodman approach, calculated using EquationEquations (A9)
(A9)
(A9) and Equation(A6)
(A6)
(A6) . Dotted red line is where points would lie if FPR was equal to the p-value. This example is calculated for n = 16, normalized effect size = 1 and prior probability P(H1) = 0.5. Log–log plot. Calculated with Plot-FPR-vs-Pval + Sellke-Goodman.R (supplementary material).
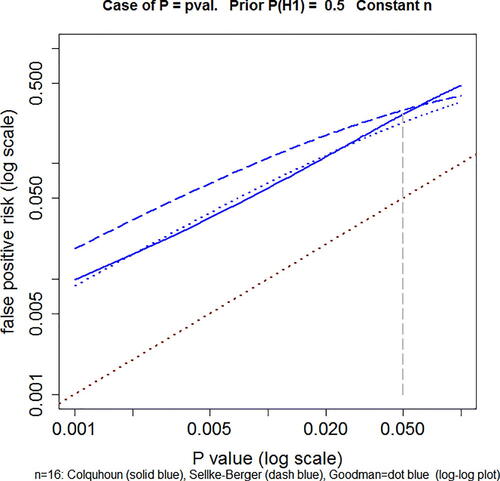
Table 2 Response in hours extra sleep (compared with controls) induced by (–)-hyoscyamine (A) and (–)-hyoscine (B). From Cushny and Peebles (Citation1905).
Fig. A1 Definitions for a null hypothesis significance test: reproduced from Colquhoun (Citation2017). A Student’s t-test is used to analyze the difference between the means of two groups of n = 16 observations. The t value, therefore, has 2(n – 1) = 30 d.f. The blue line represents the distribution of Student’s t under the null hypothesis (H0): the true difference between means is zero. The green line shows the noncentral distribution of Student’s t under the alternative hypothesis (H1): the true difference between means is 1 (1 SD). The critical value of t for 30 d.f. and p = 0.05 is 2.04, so, for a two-sided test, any value of t above 2.04, or below –2.04, would be deemed “significant.” These values are represented by the red areas. When the alternative hypothesis is true (green line), the probability that the value of t is below the critical level (2.04) is 22% (gold shaded): these represent false negative results. Consequently, the area under the green curve above t = 2.04 (shaded yellow) is the probability that a “significant” result will be found when there is in fact a real effect (H1 is true): this is the power of the test, in this case 78%. The ordinates marked y0 (= 0.526) and are used to calculate likelihood ratios for the p-equals case.
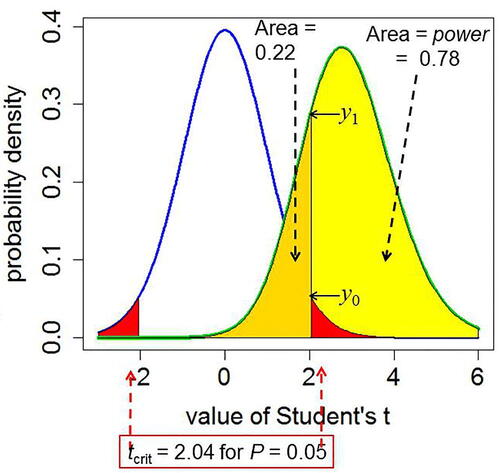