Abstract
We study the scheduling of production in a multi-item, failure-prone machine with setup times, with the objective of minimising long-run average inventory and backlog costs. We make two contributions to this problem, which has received significantly less attention compared to the standard stochastic economic lot scheduling problem (with random demand and production times). First, we adapt and implement a variety of existing scheduling policies and compare their performance through a set of numerical experiments spanning a wide range of operating conditions. This analysis allows us to identify important shortcomings of the policies, including their poor performance when items have widely different priorities (as dictated by the product of their cost and production rate parameters). Second, we leverage these insights to develop and analyse a new, easy-to-implement policy. This policy tightly controls the surplus of the highest-priority items using fixed base-stock levels and, for all other items, it determines their run lengths dynamically in such a way that long production runs become decreasingly likely with decreasing item priority. Our experiments show that our new policy greatly outperforms the benchmarking policies over a large and important set of operating regimes, comprising high-utilisation systems where the machine cycles through all items at a frequency comparable to or greater than the frequency of failure events.
Disclosure statement
No potential conflict of interest was reported by the authors.
Additional information
Notes on contributors
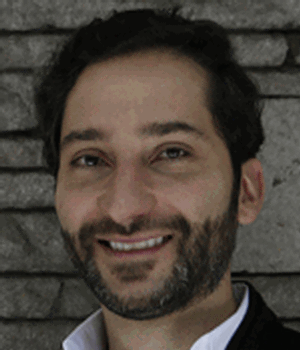
Fernando Tubilla
Fernando Tubilla is a senior director of operations research at Indigo Ag, where he leads the development and implementation of optimization algorithms for the company's grain and transportation marketplaces. Previously, he worked as a senior research scientist at Amazon Robotics, developing algorithms for inventory storage optimization, outbound flow control, and pick-work allocation, several of which have been deployed throughout Amazon's fulfillment centers. Dr Tubilla received his PhD in Systems Science from the Mechanical Engineering Department at MIT. His research interests span the areas of real-time resource allocation and scheduling in complex systems, industrial automation, logistics system optimization, and online marketplaces.
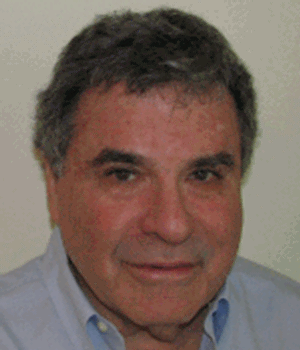
Stanley B. Gershwin
Stanley B. Gershwin is a Senior Lecturer (formerly a Senior Research Scientist) at MIT and Chief Scientific Officer of DILLYGENCE. Prior to joining MIT, Dr Gershwin worked for the Bell Telephone Laboratories and the C. S. Draper Laboratory. He is the author of “Manufacturing Systems Engineering” (Prentice-Hall, 1994) and numerous articles in the field of analytical methods applied to industry. His primary research and teaching activity has been in design and operation of manufacturing systems. His online courses, Manufacturing Systems I and II, are available through the EDX Platform. He has been a member of the IEEE Control Systems Society, the IEEE Robotics and Automation Society, INFORMS, the Institute of Industrial Engineers, and the Society of Manufacturing Engineers. He has received awards from IIE, INFORMS, and IEEE. He received a Bachelor's degree in Engineering Mathematics from Columbia University and a Master's and Doctorate in Applied Mathematics from Harvard University.