Abstract
A disassembly line is an effective disassembly system to recover end-of-life products. In real life, as end-of-life products are subject to varying degrees of wear and tear, task failure may occur in the disassembly process. In this paper, the task failure risks are considered, and an expected profit-based stochastic disassembly line balancing problem is studied. First, a mathematical model is presented to maximise the expected recovering profit with task failures. Then, a hybrid metaheuristic approach is developed to efficiently solve the proposed model, which is integrated with a variable neighbourhood descent method and an artificial bee colony algorithm. Finally, the effectiveness and robustness of the proposed algorithm are verified by three cases, and experiment results show that the solution performance of the proposed approach is superior to the other three existing methods.
Disclosure statement
No potential conflict of interest was reported by the author(s).
Data Availability Statement
The data used to support the findings of this study are available from the corresponding author upon request.
Additional information
Funding
Notes on contributors
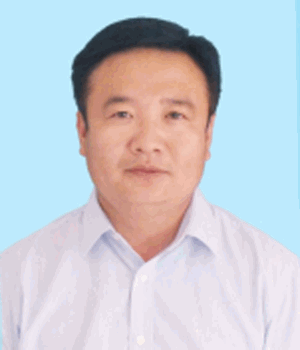
Hongfei Guo
Hongfei Guo received his Ph.D. degree, he is currently the Deputy Dean, Associate Professor, and Graduate Supervisor of the Institute of Internet of Things and Logistics Engineering of Jinan University. He serves as a specially appointed grassroots management expert for the management promotion of the State-owned Assets Supervision and Administration Commission of the State Council of the People's Republic of China. He is also a SME service consultant for the Ministry of Industry and Information Technology of the People's Republic of China, and the Deputy Secretary-General of the Industrial Engineering Branch of the Chinese Mechanical Engineering Society. His team was selected into the Inner Mongolia "Prairie Talents" High-level Talent Project, Inner Mongolia "Prairie Talents" Industry Innovation (Entrepreneurship) Talent Team and Guangzhou Industry Leading Talent Concentration Project – "Innovation Leading Team". His current research interests include Industrial Internet of Things, Digital Twins, and Intelligent Manufacturing.
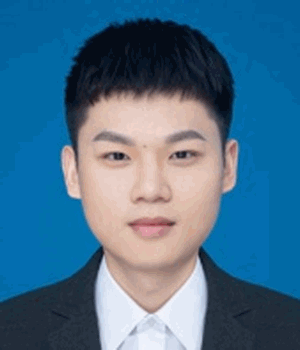
Linsheng Zhang
Linsheng Zhang received the B.S. degree in the logistics management from Yangtze University, China, in 2019. He is currently pursuing the M.S. degree with the School of Management, Jinan University (Guangzhou Campus), China. His main research interests include modelling, optimisation of scheduling problems and intelligent optimisation.
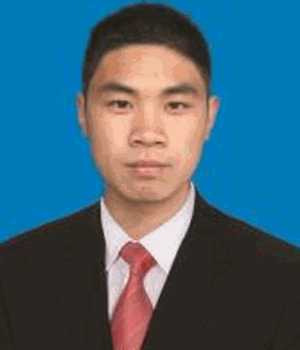
Yaping Ren
Yaping Ren received his B.S. degree in communications and transportation engineering from Liaocheng University, Liaocheng, China, in 2014, and his M.S. degree in Transportation College of Northeast Forestry University, China, in 2016. He received his Ph.D. degree in the School of Mechanical Science and Engineering from Huazhong University of Science and Technology (HUST), China, in 2019. He was also a visiting scholar in Environmental and Ecological Engineering (EEE) at Purdue University, U.S. from Sept. 2017 to August. 2019. He is currently an Associate Professor in the School of Intelligent Systems Science and Engineering, Jinan University (Zhuhai Campus). His research mainly focuses on industrial engineering, disassembly planning, transportation planning, decision making and optimisation methods.
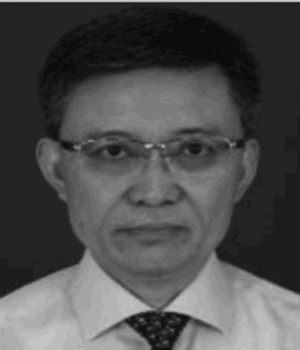
Yun Li
Yun Li (S’87–M’90–SM’17) received the B.S. degree in electronics science from Sichuan University, Chengdu, China, in 1984, the M.E. degree in electronic engineering from the Electronic Science and Technology of China (UESTC), Chengdu, China, in 1987, and the Ph.D. degree in parallel computing and control from the University of Strathclyde, Glasgow, U.K., in 1990. In 1989, he was a Control Engineer with the U.K. National Engineering Laboratory. In 1990, he was a Postdoctoral Research Engineer with the Industrial Systems and Control Ltd. From 1991 to 2018, he was a Lecturer, Senior Lecturer, and Professor with the University of Glasgow and has served as its Founding Director of the University of Glasgow Singapore. He is currently a Professor with the Dongguan University of Technology, Dongguan, China, and also a part-time Professor with the University of Strathclyde, Glasgow, U.K. He has published over 260 papers, one of which is the most popular article every month in the IEEE Transactions Control Systems Technology and another the 3rd in the IEEE Transactions Systems, Man, and Cybernetics – Part B: Cybernetics. He is the author of the popular interactive evolutionary algorithm (EA) courseware EA_demo (http://i4ai.org/EA-demo/) published online first, in 1997. His current research interest includes artificial intelligence for Industry 4.0. Dr. Li is an Associate Editor of the IEEE Transactions Evolutionary Computation and of the IEEE Transactions Emerging Topics in Computational Intelligence. He is a Chartered Engineer and an FRSA in the U.K., and has chaired the EPSRC funded first Industrial Systems in the Digital Age Conference -” Looking Beyond Industry 4.0” held in Glasgow, in 2017.
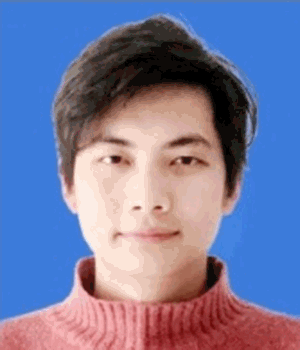
Zhongwei Zhou
Zhongwei Zhou received the B.S. degree in the engineering from Anhui Jianzhu University, China, in 2018. He is currently working toward the M.S. degree in the school of electronic information and automation at the university of Tianjin University of Science & Technology of Control Science and Engineering, China. His research mainly focuses on optimised decision.
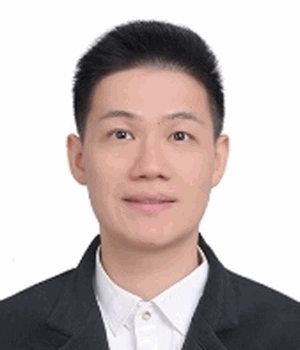
Jianzhao Wu
Jianzhao Wu received the M.S. degree in mechanical engineering from Ningbo University, Ningbo, China, in 2018. He is currently pursuing the Ph.D. degree in the State Key Laboratory of Digital Manufacturing Equipment and Technology in Huazhong University of Science and Technology with a specialisation in process modelling and intelligent optimisation methods for sustainable manufacturing systems. From October 2021 to Present, he is a Joint Ph.D. with the Department of Mechanical Engineering, National University of Singapore, Singapore.