Figures & data
Figure 1. Sample from the Philips Consumer Lifestyle BV shavers dataset.
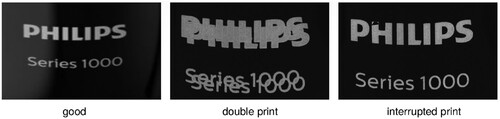
Figure 2. Sample from the Philips Consumer Lifestyle BV deco cap dataset.
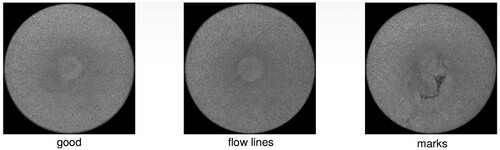
Figure 3. Sample from the Philips Consumer Lifestyle BV shaft dataset.
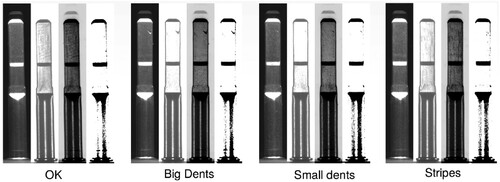
Table 1. Datasets description, describing label types and the number of data instances per label for each dataset.
Table 2. Brief description of experiments performed: their aim, method utilised, and relevant metrics.
Table 3. Description of machine learning models used across the experiments.
Table 4. Dataset composition for different degrees of imbalance. The rate describes the number of defective samples we consider w.r.t. the original dataset.
Table 5. AUC ROC measured for classified images in a binary classification setting (defective vs. non-defective) for one-shot learning. The best results for each input type are bolded, and the second-best results are highlighted in italics. We report the 95% confidence intervals.
Table 6. AUC ROC measured for classified images in a binary classification setting (defective vs. non-defective) for five-shot learning. The best results for each input type are bolded, and the second-best results are highlighted in italics. We report the 95% confidence intervals.
Table 7. AUC ROC (one vs. rest) measured for classified images in a multiclass classification setting for one-shot learning. The best results for each input type are bolded, and the second-best results are highlighted in italics. We report the 95% confidence intervals.
Table 8. AUC ROC (one vs. rest) measured for classified images in a multiclass classification setting for five-shot learning. The best results for each input type are bolded, and the second-best results are highlighted in italics. We report the 95% confidence intervals.
Table 9. AUC ROC measured for models in a binary classification setting (defective vs. non-defective) for one-shot learning. The best results are bolded, and the second-best results are highlighted in italics. We report the 95% confidence intervals.
Table 10. AUC ROC measured for models in a binary classification setting (defective vs. non-defective) for five-shot learning. The best results are bolded, and the second-best results are highlighted in italics. We report the 95% confidence intervals.
Table 11. AUC ROC (one-vs-rest) measured for models in a multiclass classification setting for one-shot learning. The best results are bolded, and the second-best results are highlighted in italics. We report the 95% confidence intervals.
Table 12. AUC ROC (one-vs-rest) measured for models in a multiclass classification setting for five-shot learning. The best results are bolded, and the second-best results are highlighted in italics. We report the 95% confidence intervals.
Table 13. AUC ROC measured for multiclass classification, considering DRAEM anomaly maps as inputs. The anomaly maps are obtained from a DRAEM model trained with all available images. We specify different degrees of artificial imbalance on top of the given one (mix rate). The exact composition of the datasets for each mix rate is described in Table . We report the 95% confidence intervals. The models using anomaly map features are bolded when performing better than those trained solely on images.
Figure 4. Plots showing AUC ROC performance based on sampling iterations for six active learning techniques and three datasets. The following naming conventions are used for the series: random (random sampling), margin (margin sampling), uncertain (uncertainty sampling), emb_resnet (EMBResNet-50), emb_pmf (EMBPMF), and GradCAM (EMBGradCAM).
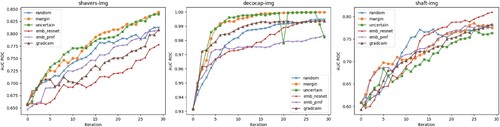
Table 14. AUC ROC measured for models in a multiclass classification setting. The best results are bolded, and the second-best results are highlighted in italics. We report the 95% confidence intervals.
Table 15. Comparison of best models, considering Image (I) and Image+Heatmap (I+H) input features. The results were taken from Table and Table regarding the binary classification case.
Table 16. Comparison of best models, considering Image (I) and Image+Heatmap (I+H) input features. The results were taken from Table and Table regarding the multiclass classification case.
Data availability statement
The data is not available due to restrictions.