Figures & data
Table 1 Common signs of correlation between nitrate concentrations [NO3] in river flows (daily values and time variability) and some hydrological variables: antecedent daily river flow (Qj–i), daily river flow (Qj) and baseflow index (BFI). CV: coefficient of variation.
Fig. 1 Reconstruction and segmentation of a daily hydrograph at an almost ungauged pollution-control site: a contribution to meta-analysis of river quality data sampling. Note that uncertainty bounds should bracket the simulated hydrograph (not shown). Segmentation was performed according to the procedure described in the section “Comparative Assessment”.
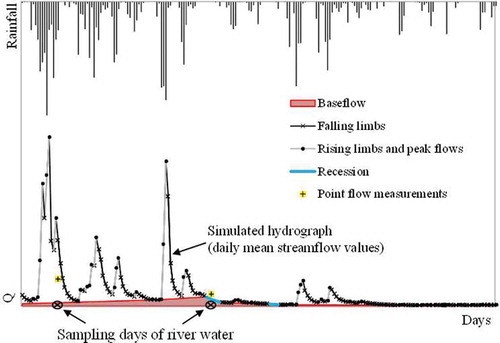
Fig. 2 Location of the 149 streamgauges and the 21 catchments of pollution-control sites used in this study.
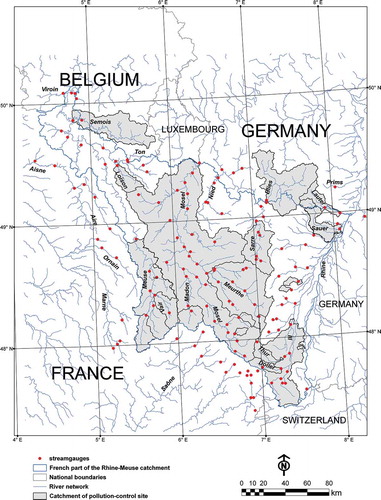
Table 2 Main characteristics of the 21 French pollution-control sites used in this study. See for locations.
Fig. 3 (a) Experimental (dots) and fitted variograms (solid line) used to model the spatial structure of Pearson’s r correlation coefficient for three representative streamgauges. (b) Observed and estimated correlations resulting from a leave‐one‐out cross‐validation of the respective variograms.
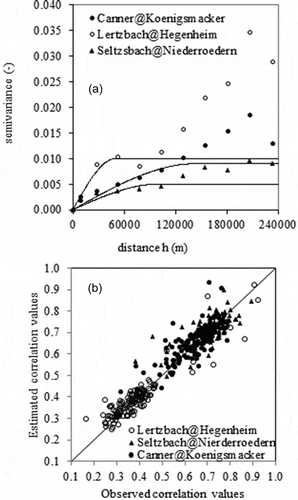
Fig. 4 Map showing the spatial distribution (ordinary kriging) of Pearson’s r values estimated between the daily streamflow time series at the Koenigsmacker streamgauge (Canner River) and all other streamgauges (•) in the study area. In the basic MC method, allowing only a single-best neighbour, the Koenigsmaker streamgauge would be used as a reference streamgauge for the Perl pollution-control site (▼) located on the Mosel River. Contours of the Canner@Koenigsmacker and Mosel@Perl catchments are also shown.
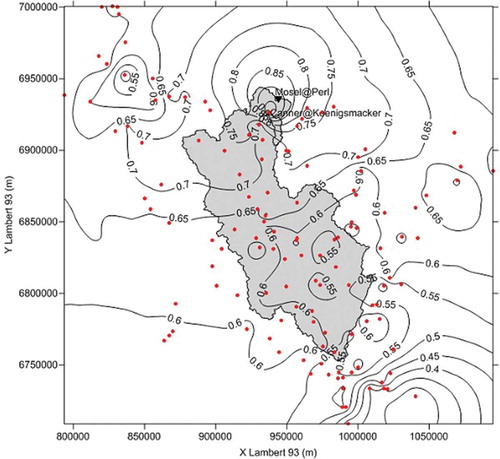
Fig. 5 Changes in mean and percentiles (10% and 90%) for the performance of the MC method according to the number of neighbour catchments,for the 21 French pollution-control sites used (1990–2009).
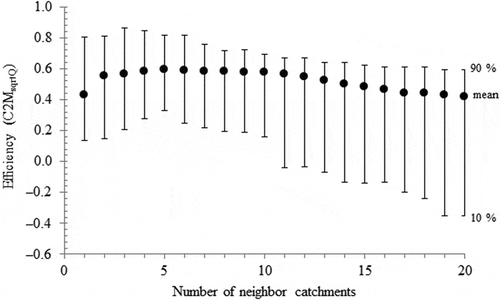
Fig. 6 Example (of the Lauter@Wissembourg catchment) of random streamflow data sampling for selecting the neighbour catchments (DISP and Q–Q methods), n = 30 d. Dots (○) are used for computing the error metric defined in equation (3). Note that point flow measurements were stopped after 2002.
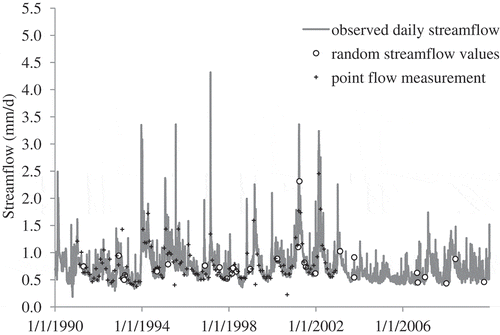
Fig. 7 Impact of the size of daily flow samples (n) and the number of NCs on the efficiency of the Q–Q method. Results are given for the 21 French pollution-control sites used in this study and the period 1990–2009.
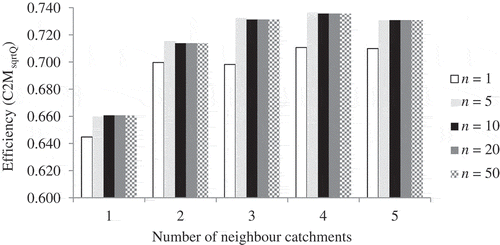
Table 3 Impact of the number n of streamflow values sampled from the daily observed hydrographs on the efficiency (C2M√Q) of the Q–Q method (option allowing four neighbours). Results are given for the 21 French pollution-control sites used in this study and the period 1990–2009.
Fig. 8 Comparison of efficiency for the four tested distance-based regionalization methods for the 21 French pollution-control sites used, 1990–2009. MC: map correlation method (option allowing five neighbours); SP: spatial proximity (output averaging); DISP: discrete parameterization of the GR4J rainfall–runoff model at the site of interest (option allowing four neighbours, n = 30 d, α = 0); Q–Q: streamflow–streamflow method (option allowing four neighbours, n = 10 d).
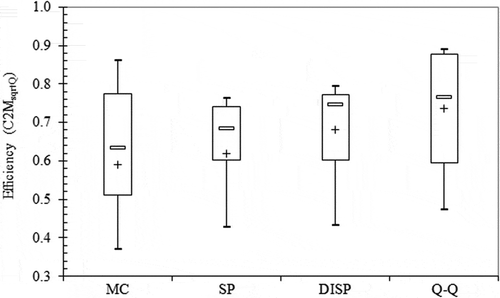
Table 4 Contingency table of days classified for different parts of the hydrograph. Results are given for the daily observed and simulated (Q–Q method) hydrographs of the River Mosel at Perl (11522 km2) for the period 1990–2009. Q–Q: streamflow-streamflow method (option allowing four neighbours, n = 10 days).
Fig. 9 Comparison of agreement for the four tested distance-based regionalization methods for the 21 French pollution-control sites used, 1990–2009: (a) rising limbs; (b) falling limbs; (c) recession (baseflows). See for legend.
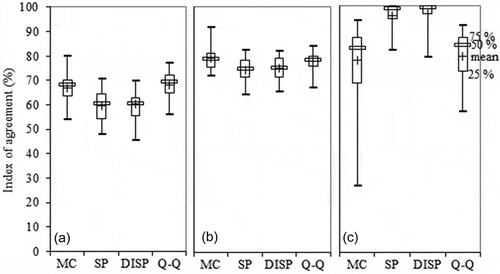
Fig. 10 Maps showing the neighbour catchment(s) (in grey) for the target catchment of the Doller@Reiningue (▼), 187 km2. (a) MC method; (b) SP method; (c) streamflow–streamflow method; and (d) DISP method. The four neighbours whose model parameters occur in more than half of the 100 simulations of n are indicated by white circles.
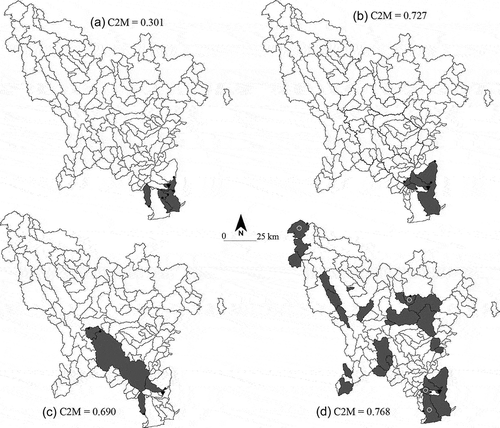
Fig. 11 Comparison between point flow measurements and daily streamflow values. (a) Mosel@Sierck (pollution-control site) vs Mosel@Perl (streamgauge station): 270 days; the mean annual streamflow is close to 145 m3/s. (b) Meurthe@Fraize: 156 days; the mean annual streamflow is close to 2 m3/s. Measurement period refers to 1990–2005 in both cases.
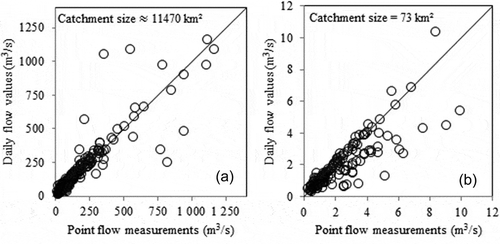
Fig. 12 Changes in mean and percentiles (10% and 90%) for the performance of the Q–Q method according to the reduction of the density of the donor streamgauge network for the 21 French pollution-control sites used, 1990–2009. Horizontal lines provide the mean efficiency of each of the three other regionalization methods considering the full (i.e. existing) donor streamgauge network, with the number of donor catchments for the current density level in parentheses. See for legend.
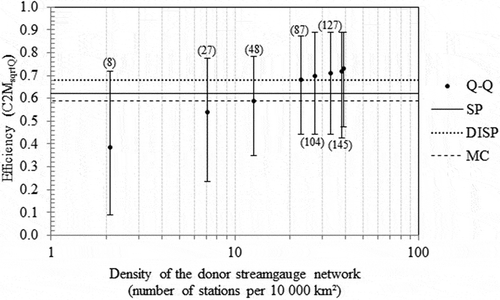