Figures & data
Figure 1. Map of the study area showing the Upper Mississippi River Basin (top) and its subwatersheds (bottom left and right). Stars indicate streamflow gauging stations used in this study. Note that the subwatershed downstream of the gauging stations are excluded in the models (shown by lighter colors). The sub-watershed IDs are labelled according to USGS Watershed Boundary Dataset (WBD) Hydrologic Units (HU). USGS gauge numbers are given for the watershed outlets.
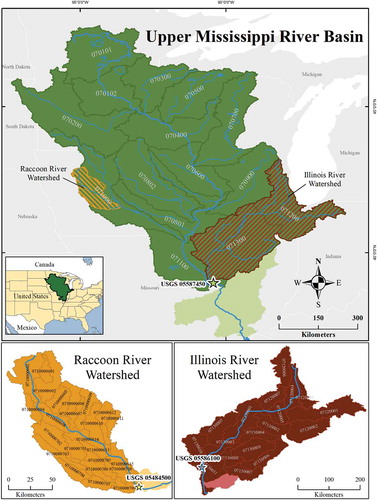
Table 1. Summary of watershed characteristics of the study sites.
Figure 2. Summary of data sources and processing applied on the dataset used in the MLM. Processed variables were resampled according to the HUs of each study site.
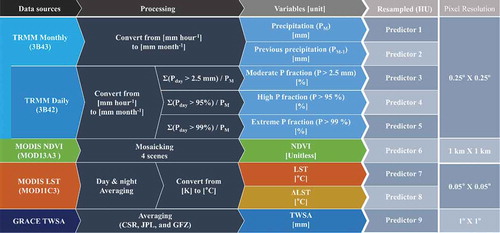
Figure 3. Decision tree processes (a) showing an example of covariant space where Y (predictand or target) is explained by X1 and X2 (predictors). The covariant space can be approximated by rectangular spaces (b) and (c), each representing the first node and entire nodes of the decision tree (d).
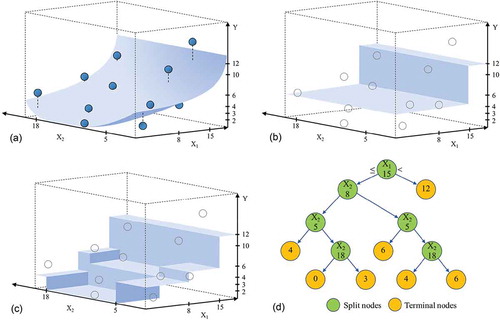
Table 2. Performance metrics for training and testing.
Figure 8. Scatterplots of model-simulated vs observed streamflow for training: (a) RRW, (c) IRW, and (e) UMRB; and testing: (b) RRW, (d) IRW, and (f) UMRB.
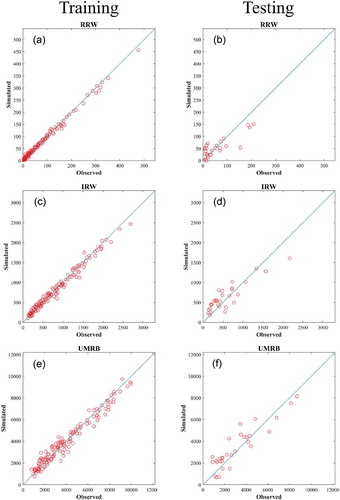
Figure 9. Time series plots of model-simulated streamflow and observed streamflow for the entire study period for (a) UMRB, (b) IRW, and (c) RRW.
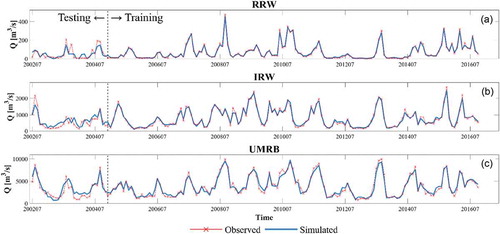
Figure 10. Relative importance of predictor variables for each watershed: (a) RRW, (b) IRW, and (c) UMRB.
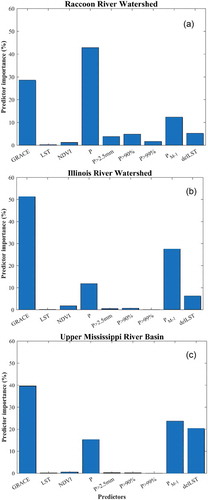
Figure 11. Map showing the relative contribution of sub-watersheds in simulating the MLMs for each watershed. Darker shading shows high relative importance and lighter shading shows low or no importance.
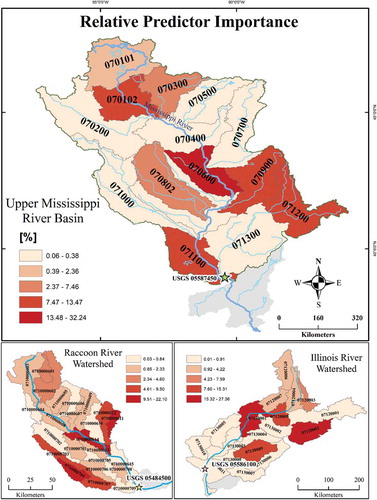
Table 3. Comparison of streamflow estimation performances between existing process-based model results (SWAT) and machine learning modellng (MLM) for each study site.