Figures & data
Table 1. Hydrological variables inferred from satellite images planimetric properties. ACC: accuracy; RMSE: root mean square error; RRMSE: relative root mean square error (divided by mean of observations); r2: coefficient of determination; : predicted value,
: observed value
Figure 1. Location of the São Francisco River reach used as test area showing the in situ hydrological stations (left) and geographical context in the South American continent (right)
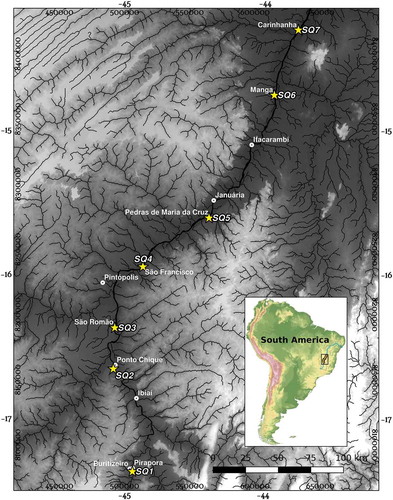
Table 2. Station location
Figure 4. K-fold cross-validation example for k = 5. Light grey: training data, Dark grey: test data. The method first divides the data set in k subsets, then uses k – 1 subsets for training while keeping the remaining one for validation. k iterations are performed, each one testing a model build from a different fold generating a validation estimate. The mean absolute error (MAE) was used here
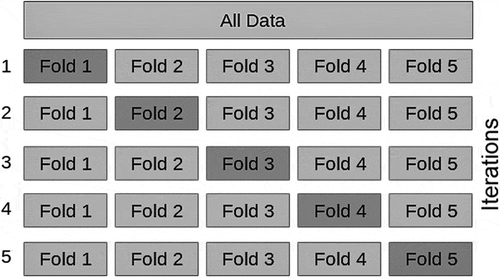
Figure 5. The three different methods of virtual (width) stations definitions: (a) individual width () at-a-station, (b) systematic average width stations (
) calculated for the whole river reach every 10 km and (c) selected average width stations (
) defined by statistically selecting river sections with the most width variations
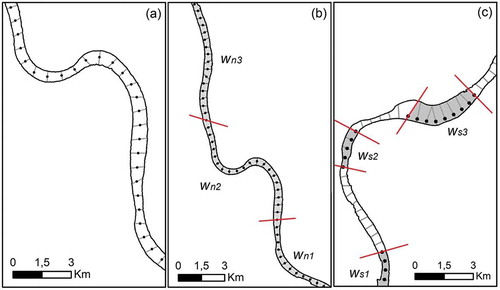
Figure 6. Illustration of the set of 952 river cross-sections widths organized by quartiles (25% = 55.5 m, 50% = 90.62 m, 75% = 228.86 m)
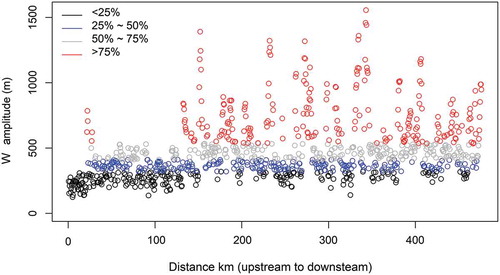
Table 3. Transferred discharge (QWQ) of station SQ3 to a virtual station 64 km downstream that coincides with station SQ4. The PFE gives an idea of the errors generated by the approach
Figure 7. Transferred discharge data (SQ QWQ) of station SQ3 to a point 64 km downstream that coincides with station SQ4.
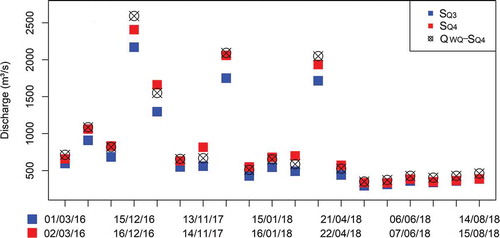
Figure 9. Evaluation metrics used to compare the results from the three discharge estimation methods: (a) individual width, ; (b) systematic average,
and (c) selected average,
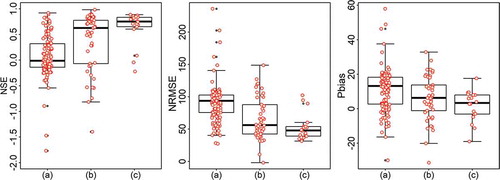
Figure 10. The best curves for the relationship by method and the respective analysis sections in the river
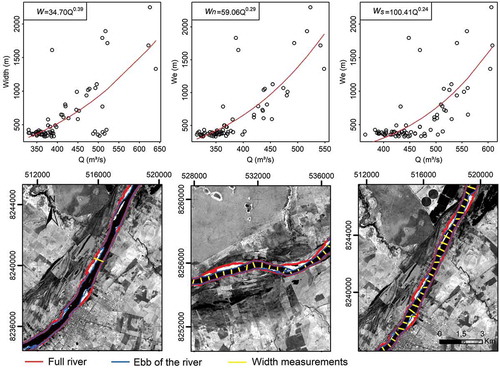
Table 4. Best fit power functions achieved by each method
Table 5. Models method. Bold values indicate best model results
Figure 12. Daily in situ discharge (Qobs) data (line) compared with estimated discharge calculated from the method for the period March 2016–August 2018
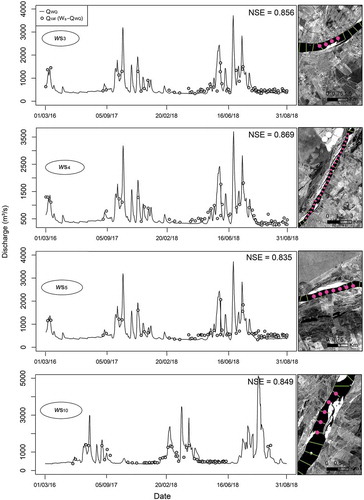
Figure 14. Relationships between (a) exponent and width amplitude, and (b) NRMSE and width amplitude
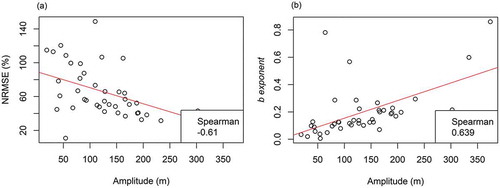
Figure A1. Top Sentinel-1B SAR image of the Pampulha Reservoirs complex. Bottom: difference maps between the SAR image classification results and the precise delineation. Grey pixels represent false negative errors (omitted pixels) and black pixels represent false positive errors (falsely included pixels). Reproduced with permission from the authors
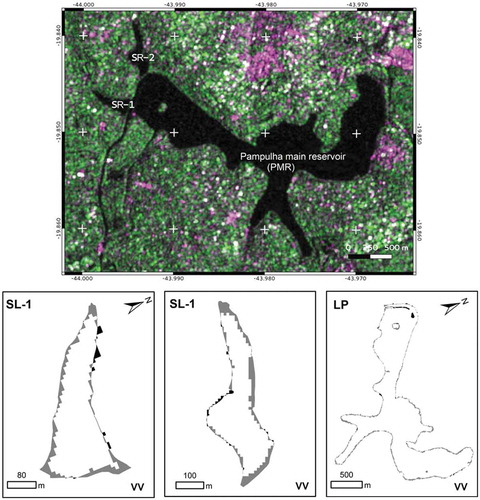
Figure A2. Left: PlanetScope false colour image. Right: water surfaces extracted from Lansat-8, Sentinel-1 and Sentinel-2 images. The background cyan colour shows the reference surface from the Planet Scope image used for determination of accuracy. Reproduced with authorization from the authors
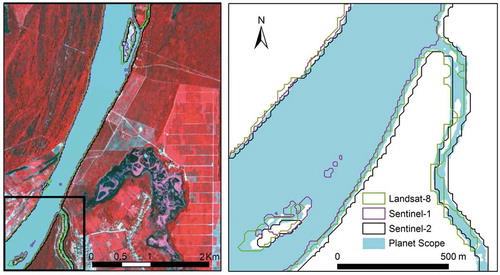
Table A1. List of images acquired with some of their specification and the associated water stage level
Table A2. Water surface classification accuracy by sensor, spectral bands and polarizations