Figures & data
Figure 1. Observation extents in the West Castle Watershed (WCW); (a) elevation gradient in the WCW, with crosshatched shading showing areas that were visible in Mountain Legacy Project (MLP) imagery, (b) SPOT 6 RGB image of the WCW, 150 cm resolution, captured 31 July 2014, copyright 2014 CNES – shaded areas show the Alpine Treeline Ecotone (ATE) region of the watershed, 1700–2200 m a.s.l.
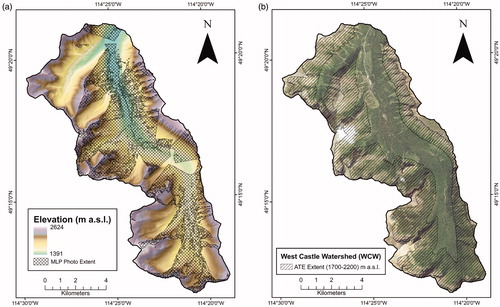
Figure 2. Image classification workflow, adapted from McCaffrey and Hopkinson (Citation2017); (a) an oblique image of Mount Haig, in the West Castle Watershed (WCW); (b) a 20 m fishnet applied to the orthogonal Mount Haig view shed; (c) fish net grid projected back to oblique view using WSL monoplotting tool; (d) oblique image with ordinal canopy cover classification, red = no cover, yellow = low vegetation, light green = partial canopy, dark green = full canopy; (e) orthogonal image with canopy cover classification; (f) change class for Alpine Treeline Ecotone (ATE) elevation (1700–2200 m a.s.l.), red = vegetation decrease, beige = no change, green = vegetation increase. Background images in a, c, and d copyright Mountain Legacy Project 2016, and in b, e, f, copyright CNES 2014.
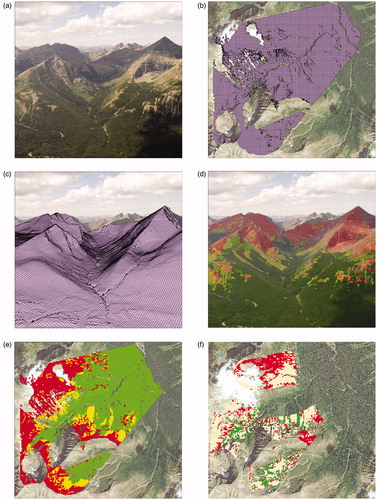
Figure 3. Canopy change classification for area observed in Mountain Legacy Project (MLP) imagery, at Alpine Treeline Ecotone (ATE) elevation. Areas of vegetation decrease, in red, occur predominantly on warm slopes, while areas of vegetation decrease, in green, occur on cool slopes.
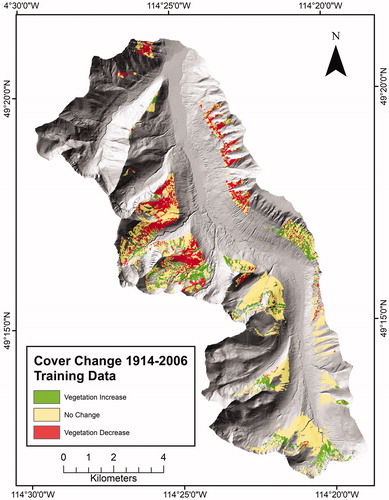
Figure 4. Variables used in random forest model training; (a) elevation, (b) compound topographic index (CTI), (c) curvature, (d) slope, (e) annual solar radiation, (f) aspect, (g) fire occurrence, (h) surficial geology; and model validation, (i) fractional cover.
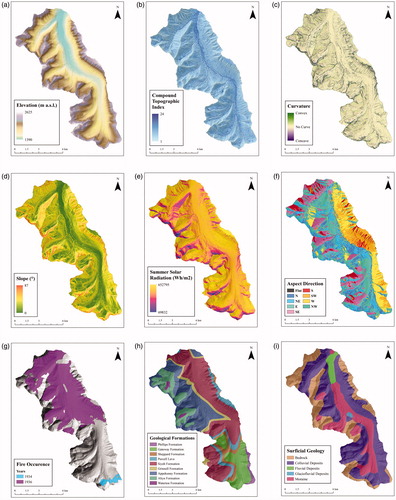
Table 1. Summary of continuous variables used in random forest model.
Table 2. Summary of categorical variables used in random forest model.
Table 3. Confusion matrix for vegetation change classes, with overall accuracy, precision, recall, and Cohen’s Kappa scores.
Figure 5. Predicted Alpine Treeline Ecotone (ATE) canopy change classification in areas of the West Castle Watershed (WCW) that were unobserved in Mountain Legacy Project (MLP) imagery. Predictions were also provided for observed areas, which are labeled as training areas (shaded). Vegetation decrease is generally predicted on warm, fire-exposed aspects, and vegetation increase is predicted on cool, non-fire exposed aspects.
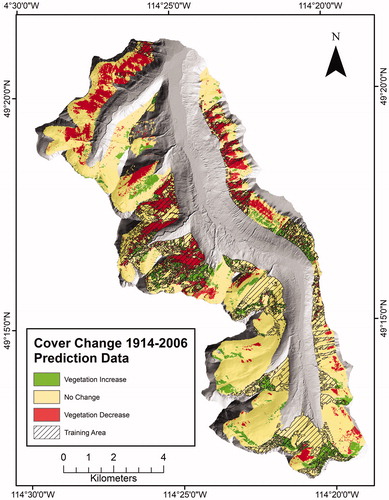
Figure 6. Boxplots of LiDAR-derived fractional cover measurements, comparing Alpine Treeline Ecotone (ATE) canopy cover change classifications. On the left, observed change classes, and on the right, change classes predicted in the random forest model. In both conditions, fractional cover in the vegetation increase class is significantly higher than the in the vegetation decrease class.
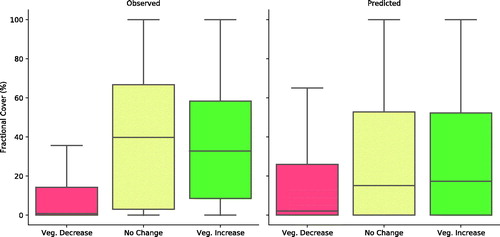
Figure 7. Boxplot showing permutation importance for the 21 variables used in the initial model accuracy assessment. Great accuracy reduction is associated with higher importance, as the model loses more accuracy when these variables are permuted. PET: Potential Evapotranspiration; CTI: Compound Topographic Index.
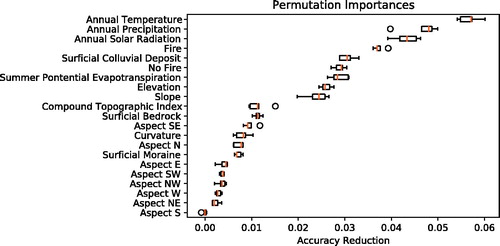
Figure 8. Pearson correlation matrix for the continuous model variables. PET: Potential Evapotranspiration; CTI: Compound Topographic Index.
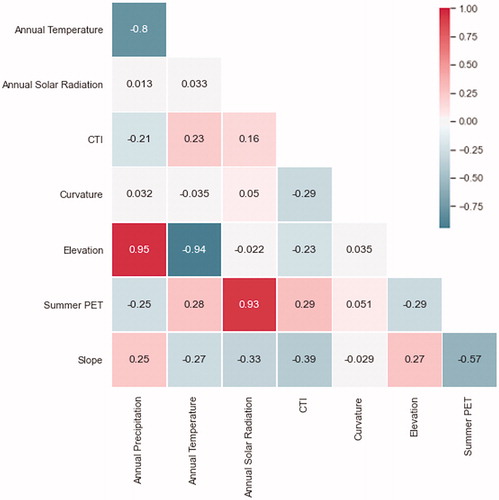
Figure 9. Boxplot showing permutation importance for the five model variables selected by the recursive feature elimination algorithm.
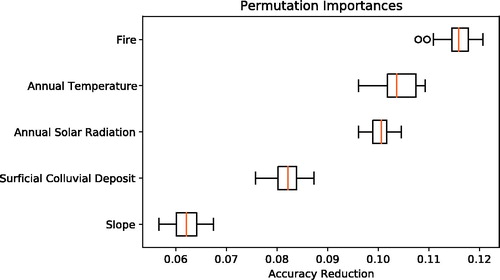