Figures & data
Figure 1 Schematic diagram of the setup for FT-NIR measurement of pear fruit in the wavelength of 800–2500 nm.
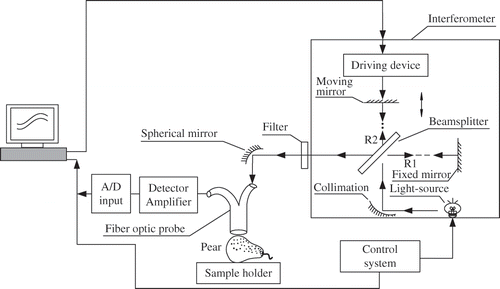
Figure 2 Typical spectra of log (1/R) and the first derivate of the reflectance reciprocal, D1 log (1/R) for pear fruit.
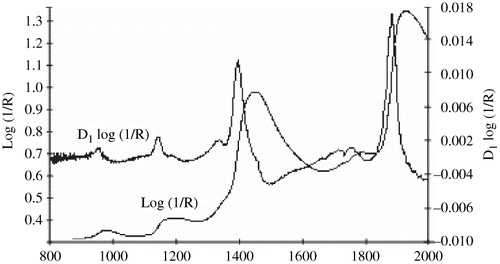
Table 1 Summary statistics of parameters of pear samples in this experiment
Table 2 Means, ranges, and SDs of pear samples of calibration and validation sets
Table 3 Correlation coefficients between SSC and TA parameters about 248 pear samples
Table 4 The results for SSC and TA calibration models of PLS regression methods for log (1/R) using different spectral correction in the wavelength range of 814–1834 nm
Table 5 The results for calibration models of PLS regression methods for the log (1/R), means values of RMSEC, RMSEP, and RMSECV, the correlation coefficients of determination, r with 814–1834 nm, 1155–1834 nm, and 814–1155 nm wavelength ranges
Table 6 The results for calibration models of PLS and PCR methods for the log (1/R), first derivative D 1log (1/R), and its second derivative D 2 log (1/R), means values of RMSEC and the coefficients of determination, r 2
Figure 3 Calibrations of partial least square regression by the FT-NIR system versus laboratory measurements of soluble solids content of pear fruit.
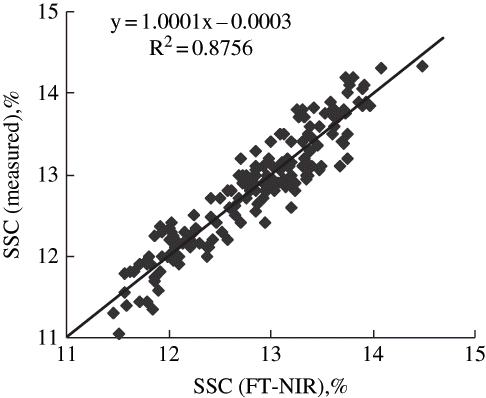
Table 7 The results for prediction errors of PLS regression method and r 2, the coefficients of determination for the log (1/R) based on about 33% of the data set, for SSC and TA parameters
Figure 4 Predictions of partial least square regression by the FT-NIR system versus laboratory measurements of soluble solids content of pear fruit.
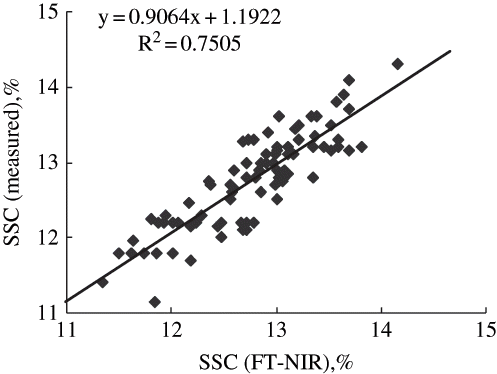
Figure 5 Predictions of partial least square regression by the FT-NIR system versus laboratory measurements of titratable acidity of pear fruit.
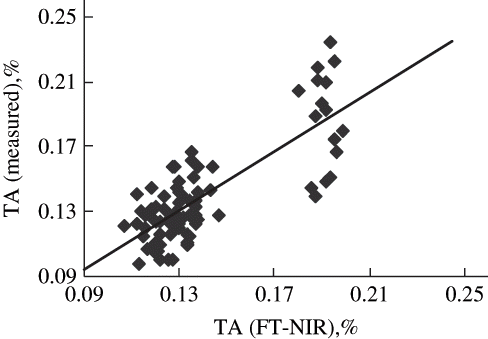