Figures & data
Table 1. Safranal quantification for different saffron samples using UV–visible spectroscopy
Figure 2. PCA score plot for the characterization of 33 saffron samples having different aroma strengths.
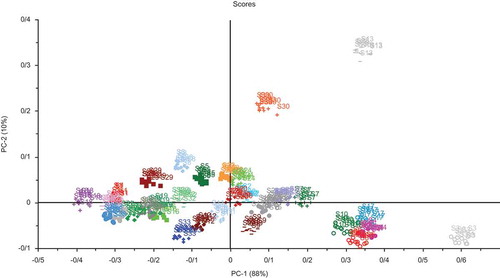
Figure 4. MLP model A-correlations between the predicted and actual values, from left to right: training, validation, and test sets (data of all the aroma features with 30 neurons in the hidden layer).
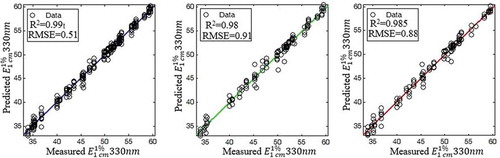
Figure 5. MLP model B-correlations between the predicted and actual values, from left to right: training, validation, and test sets (all the aroma features excluding the less-effective ones and 30 neurons in the hidden layer).
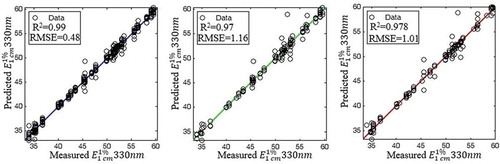
Figure 6. MLP model C-correlations between the predicted and actual values, from left to right: training, validation, and test sets (only the more effective aroma features with 30 neurons in the hidden layer).
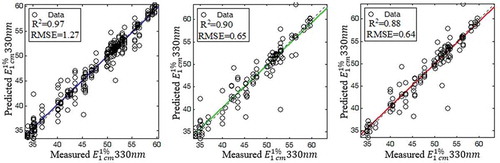
Figure 7. MLP model D- correlations between the predicted and actual values, from left to right: training, validation, and test sets (data from MOS sensors excluding the less-effective sensors and 50 neurons in the hidden layer).
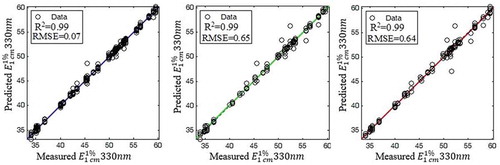
Figure 9. RBF model E, correlations between the predicted and actual (data of all the aroma features).
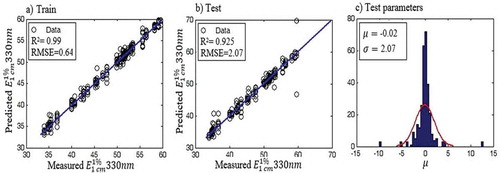