Figures & data
Figure 1. Block diagram of the proposed method for classification between pesticide exposed and fresh water fish.
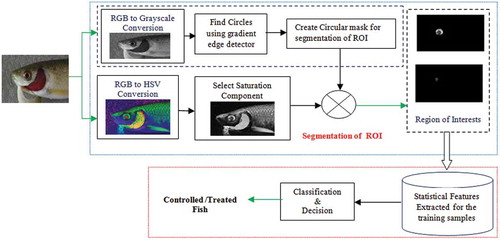
Figure 2. ROI segmentation in fish (a) Original RGB image (b) Grey scale image (c) Binary mask of ROI (d) Extracted S channel using binary mask.
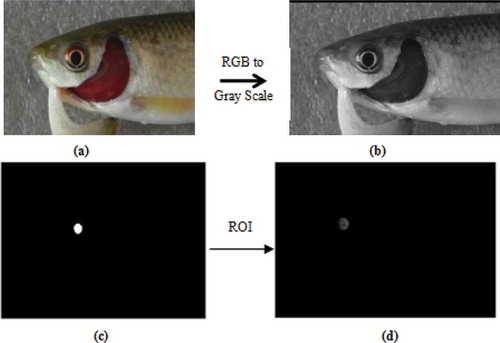
Figure 3. ROI segmentation in fish (a) Original RGB image (b) Saturation channel image (c) ROI (eye) (d) ROI (pupil of the eye).
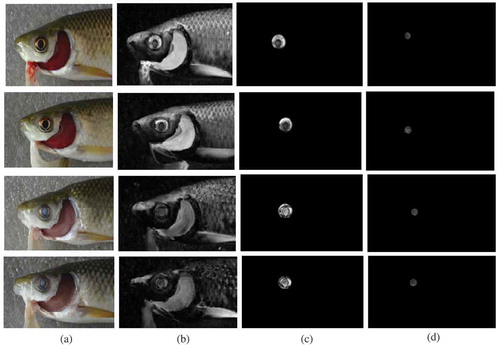
Table 1. Appearance of Eye.
Table 2. p-value of extracted features on basis of pixel intensity.
Figure 8. Process of Artificial neural network used for classification of treated and untreated fish.
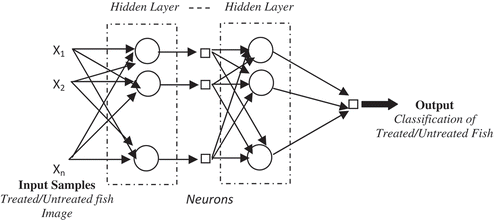
Table 3. Statistical parameters of ROI (pupil of the eye) on basis of pixel intensity for untreated and treated fish.
Table 4. Statistical parameters of ROI (eye) on basis of pixel intensity for untreated and treated fish.
Table 5. Accuracy of classification using different classifier for pupil of the eye as ROI.
Table 6. Computation time taken by proposed method for ROI segmentation andfeature extraction (for some samples).