Figures & data
Figure 1. a) Cluster analysis. An overview of how the dimension reduction occurs. 1. The original matrix may have n number of columns with n variables. 2. In the example a matrix of 5 dimensions is depicted, and then exemplified that it’s not possible to plot a 5 dimension plot. 3. Depicts the step of covariance matrix creation. 4. The covariance matrix selection gives way to the eigenstuff calculation, the eigenstuff includes the eigenvectors (λ), and eigenvalues (ν), which together build the principal components. 5. Principal components now can be compared against each other, creating a 2D plot. 6. The PCs create now a reduced matrix in comparison with the input matrix, allowing an easier interpretability. b) Dendrogram of a hierarchical cluster analysis. The Dendrogram depicts where the leaves and nodes are localized. The HCA was able to find two clusters, green and red. c) K means example. The image shows where the random centroids were placed after the final iteration. The distance border, commonly Euclidean distance, helps to the data points to find the closest distance to the closest centroid.
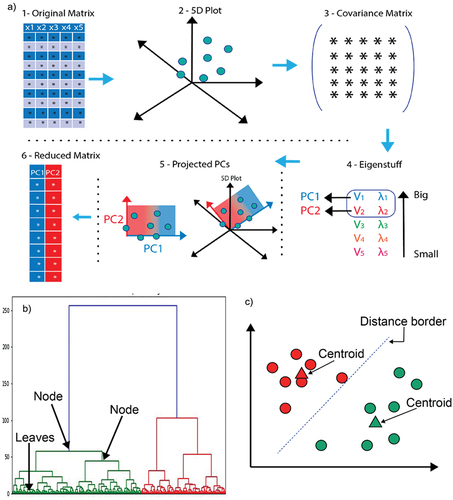
Figure 2. Overall structure of an ANN. Each ANN consists of different process units, and layers. The activation function works within each process unit.
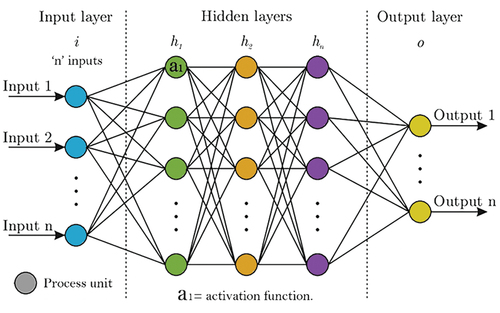
Table 1. Highlights of the supervised models presented. This table aims to provide an overall overview of advantages and disadvantages of the machine learning supervised methods.