Figures & data
3. Detection results when applying the traditional models to high-spatial-resolution images. A. Original image. B. Detection result using the Itti et al.’s model. C. Detection result using the Achana method. The disk-shaped detected regions make it difficult to obtain accurate detection results with the Itti et al. model. The Achanta model regarded some unexpected regions as ROIs.
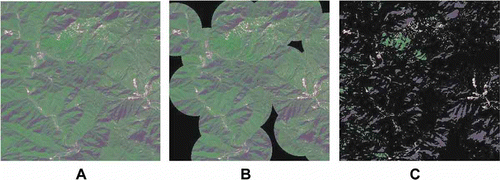
4. Framework of the adaptive spatial subsampling visual attention model. The key steps in the model are shown with a blue background.
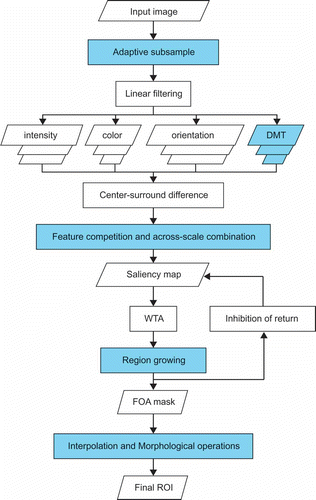
5. Response of the DMT with different p, q Combinations. A. Original image. B. p = 0, q = 0. C. p = 0, q = 1. D. p = 1, q = 0. E. p = 1, q = 1. When p and q form an even-odd combination, the DMT responds strongly for regions with great changes of intensity in the vertical orientation. For the odd-even combination, the DMT responds strongly for the regions with great changes of intensity in the horizontal orientation. The odd-odd combination leads to a smooth result. The even-even combination produces a result similar to the input image.
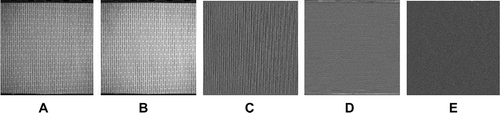
6. Examples of conspicuity maps and final saliency maps. A. Original image. B. Iintensity map. C. Color map. D. Orientation map. E. DMT map. F. Saliency map. The numbers below the images are the corresponding coefficient obtained using our feature competition strategy.
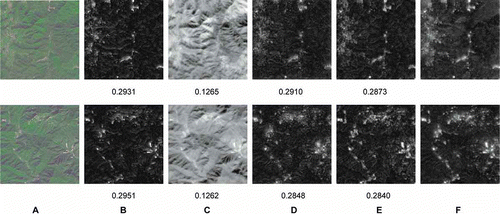
1 Processing Time When Different Levels of Subsampling Are Applied.
2 Processing Time of the Itti et al., Achanta et al., and the ASS-VA Modelsa.
8. Detection results when applying different levels of subsampling. A. Original image. B–F. Detection results with the subsample level p = 0…4. When the subsample level increases, some tiny regions are lost and the detection results become less accurate.
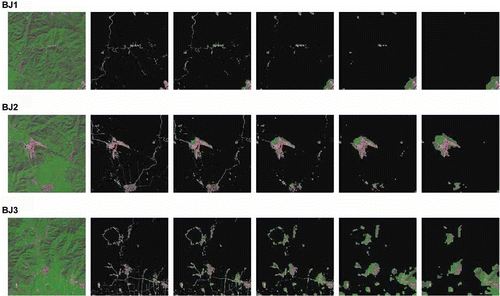
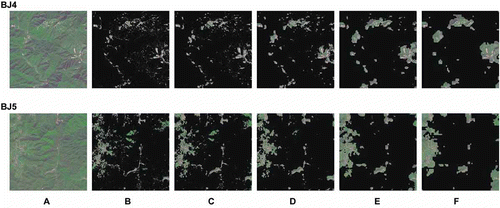