Figures & data
Figure 1. (a) mono-label classification (ml) with two land-use classes (gray and black) and (b) Multi-Label classification (ML) with cells belonging to both classes (squares split by the two colors).
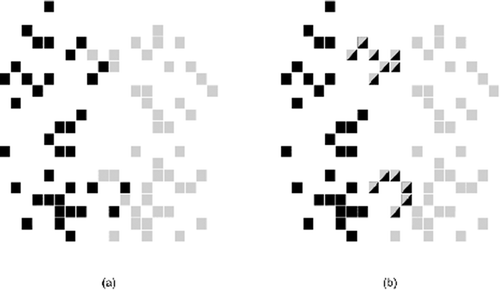
Figure 3. Conversion of a vector map to a raster map with ML: (a) vector data containing polygons with land-use codes; (b) rasterizing vector data; (c) the value of each grid in raster space depends on the land-use code of the polygons which cover them.
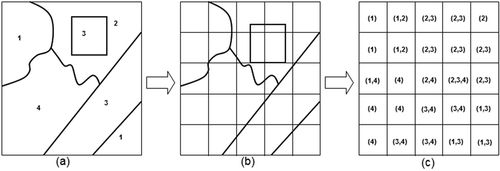
Figure 4. Architectures of the neural network BP-MLL (in the left) and BP (in the right) showing input, hidden and output layers.
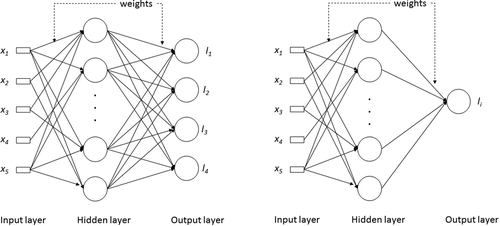
Figure 5. Processing steps of the ML-CA-LTM model. Note that the estimated label set for a given cell is fixed by a threshold which is to be optimized, as suggested by Zhang and Zhou (Citation2006).
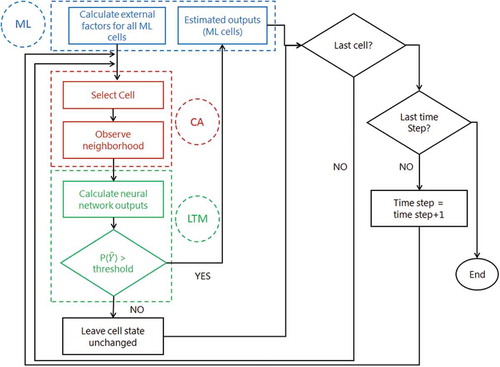
Table 1. Example of a testing set.
Table 2. Driving features as input of the model.
Table 3. Multi-label land-use data in 2007.
Figure 8. Architecture of the ML-CA-LTM model (drivers X1 − X16 are explained in ; cell states are agriculture (1), forest (2), industrial (3) and urban (4)).
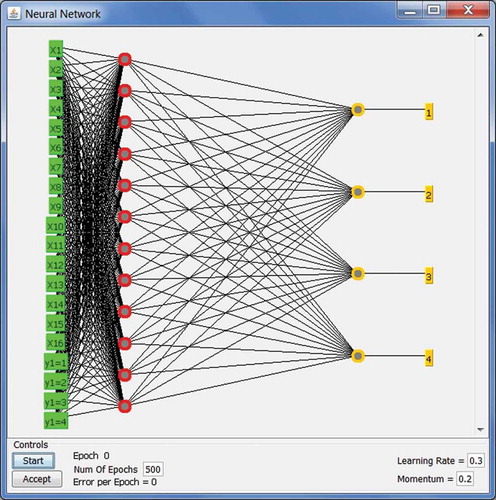
Table 4. Assessing the performance of the ML-CA-LTM model.
Figure 11. Confusion matrix with multi-labeling, observed versus predicted label set in 2007 (Note: normalized values between 0 and 100: each value in the original confusion matrix is divided by the sum of its corresponding line and multiplied by 100).
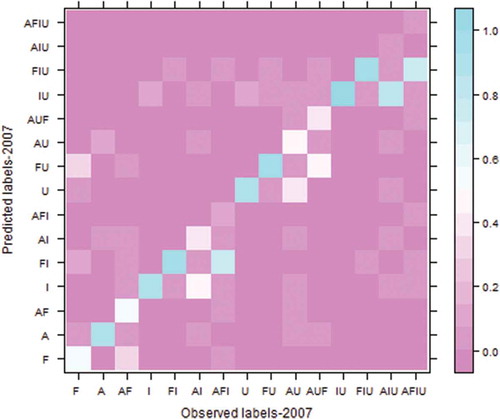
Table 5. Confusion matrix with mono-labeling, observed vs. predicted label set in 2007.
Table 6. Calculated accuracy metrics for ML-CA-LTM and ml-CA-LTM models (k = 3 and h = 12); based on 100 replications.