Figures & data
Figure 2. Flow chart for SAS, including three steps: (a) producing continuous multiscale segments by SWA approach; (b) calculating features for each scale from the first step and combining them later to learn selfhood scales; (c) finishing the merging based on the learned selfhood scales and generating self-adaptive results.
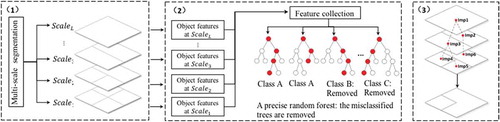
Figure 3. Flow chart for Segmentation by Weighted Aggregation (SWA) approach (Sharon et al. Citation2006).
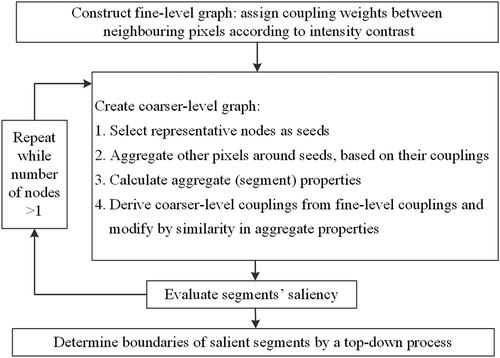
Figure 4. Self-adaptive merging based on learned selfhood scales. (a) is the simplified tree representation of the workflow for self-adaptive merging. Each object in level 0 represented by leaf node searches for an appropriate segment bottom-up according to the learned selfhood scale, indicated by the corresponding color. Then the tree splits into subtrees with father nodes representing new segments. (b) is the concrete merging illustration. It shows the merging process of objects step by step.
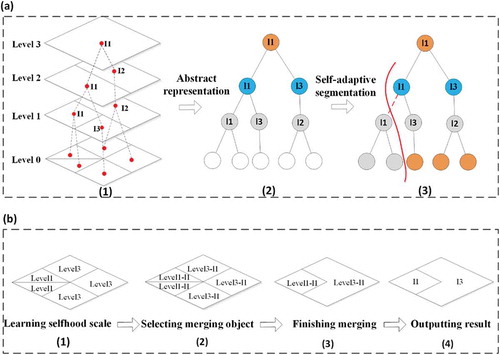
Figure 7. Selfhood scale learning results for (a) urban area and (b) farmland area. Each object was colored differently according to its selfhood scale learned from multiscale SWA results, ranging from level 5 to level 9.
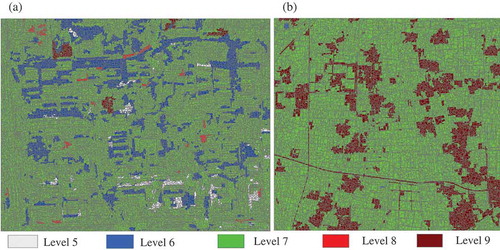
Table 1. The per-category proportions of different selfhood scales in urban area.
Table 2. The per-category proportions of different selfhood scales in farmland area.
Figure 8. A comparison between SWA and SAS results in urban area. (a) The original image and (b) the SAS result. A heterogeneous region, marked with yellow rectangle, is chosen to visually compare the six kinds of segmentation results at (c) level 5, (d) level 6, (e) level 7, (f) level 8, (g) level 9 of SWA results and (h) SAS result.
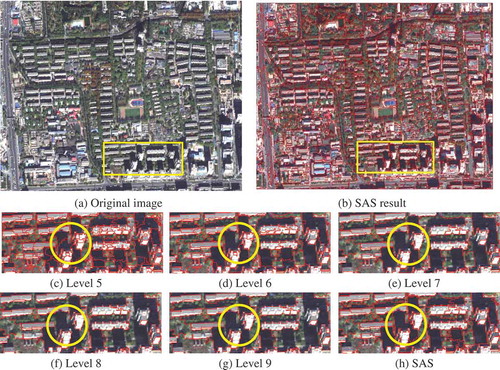
Figure 9. The manually delineated reference data used in geometry-based evaluation for (a) urban area and (b) farmland area.
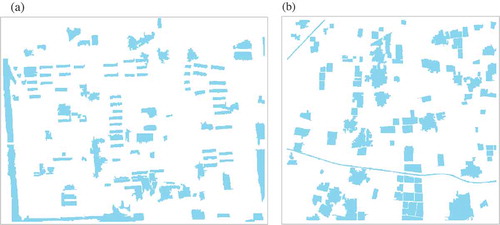
Figure 10. A comparison between SWA and SAS results in farmland area. (a) The original image and (b) the SAS result. A heterogeneous region, marked with yellow rectangle, is chosen to visually compare the six kinds of segmentation results at (c) level 5, (d) level 6, (e) level 7, (f) level 8, (g) level 9 of SWA results and (h) SAS result.
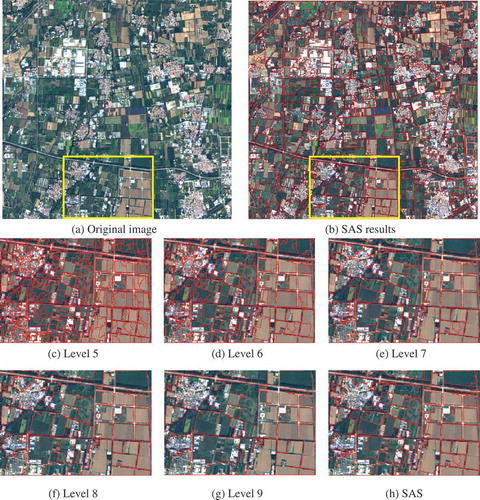
Table 3. Evaluation statistics of quantitative comparisons between SWA and SAS results (in urban area).
Table 4. Evaluation statistics of quantitative comparisons between SWA and SAS results (in farmland area).
Table 5. Evaluation statistics of quantitative comparisons between mean shift and SAS results (in urban area).
Table 6. Evaluation statistics of quantitative comparisons between mean shift and SAS results (in farmland area).