Figures & data
Figure 1. (a) A residual unit proposed in ResNet-101 (He et al. Citation2016a). (b) An improved residual unit proposed in ResNet-101-v2 (He et al. Citation2016b).
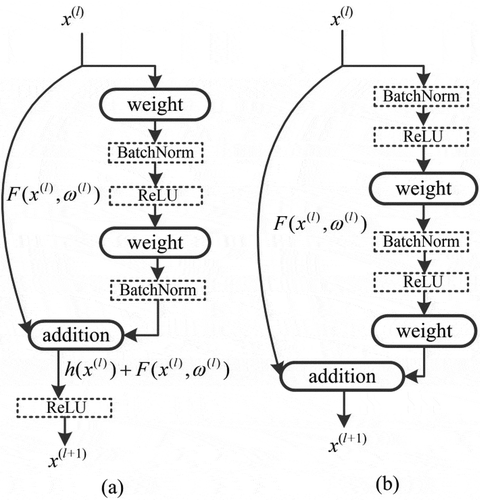
Figure 2. A building block with a bottleneck design for ResNet-101 (He et al. Citation2016a).
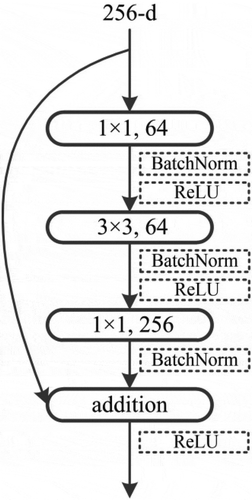
Figure 3. Overview of the PSPNet (Zhao et al. Citation2017a).
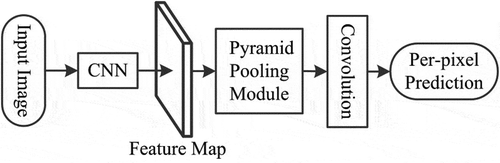
Figure 4. Pyramid pooling module (Zhao et al. Citation2017a).
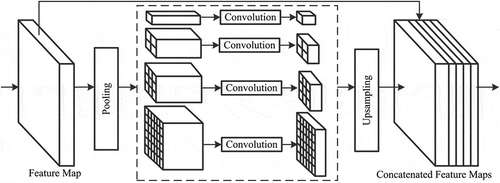
Table 1. The dimensions of 16 TOP tiles in the training set and 17 TOP tiles in the testing set.
Table 2. Experimental results with a different number of auxiliary losses. Loss 1, 2 and 3 are shown in .
Table 3. Evaluation of results in the Vaihingen dataset using the full reference set.
Table 4. OAs of results in the Vaihingen dataset using the reference set with eroded boundaries.
Figure 6. Example results of test images in the Vaihingen dataset. (a) The original image, (b) the results of SP-SVL-3, (c) the results of CNN-HAW, (d) the results of CNN-FPL, (e) the results of PSPNet and (f) our results. White: impervious surfaces, Blue: buildings, Cyan: low vegetation, Green: trees, Yellow: cars, Red: clutter/background. (Best viewed in color version).
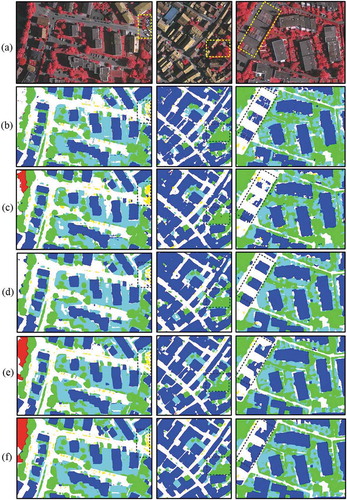
Table 5. Evaluation of results in the Potsdam dataset using the full reference set.
Table 6. OAs of results in the Potsdam dataset using the reference set with eroded boundaries.
Figure 7. Example results of test images in the Potsdam dataset. (a) the original image, (b) the results of SP-SVL-3, (c) the results of CNN-HAW, (d) the results of CNN-FPL, (e) the results of PSPNet and (f) our results. White: impervious surfaces, Blue: buildings, Cyan: low vegetation, Green: trees, Yellow: cars, Red: clutter/background. (Best viewed in color version).
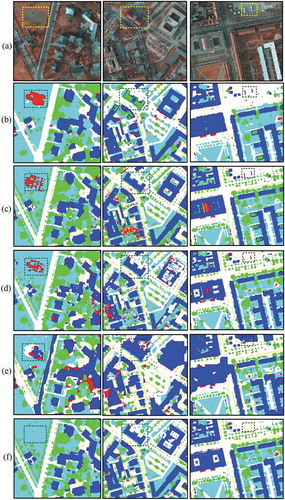