Figures & data
Table 1. Sentinel-1 SAR data list used in this study (the data between DOY 154 and DOY 178 is missing).
Figure 5. The study area in northern Hunan Province, China. The right-hand section provides an overview of the SAR data (R: 23 May 2017 VH polarization, G: 26 October 2017 VH polarization, B: 3 August 2017 VV polarization), roadmaps and sample spots of the field survey.
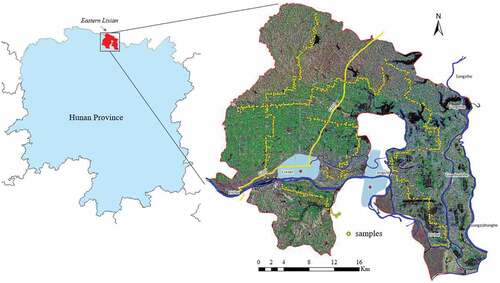
Table 2. Comparison of overall accuracy, kappa coefficient and F1 measure values conducted using the SVM-, RF-, and LSTM-based classifiers for Hunan.
Figure 8. VH intensity time-series curves for crops (the upper section) and their corresponding crop calendars (the lower section) for Hunan.
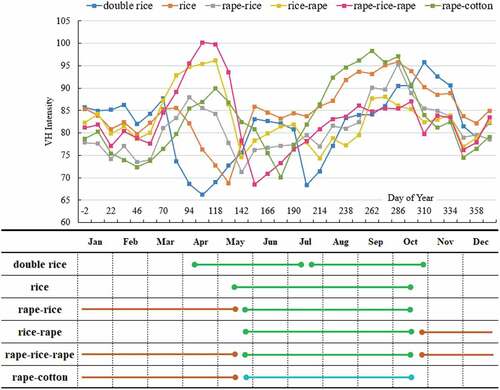
Table 3. Classification performance using multivariate and univariable time series for Hunan.
Table 4. Comparison of overall accuracy values for Guizhou using the SVM-, RF-, and LSTM-based classifiers.