Figures & data
Figure 1. Study areas and samples location. (a) Santa Catarina state; (b) Curitibanos municipality; (c) Zoom showing the areas location (Google Earth); (d) Area 1; (e) Area 2.
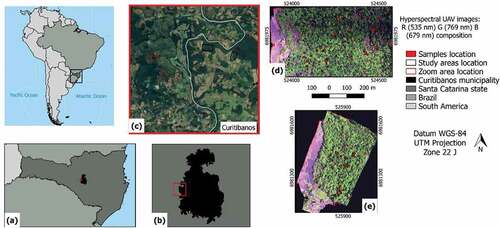
Table 1. Characteristics of the camera, flight and data acquired in the study areas.
Table 2. Tree species and their respective successional groups and number of ITCs and pixels for each tree species used in the classification process according to the study area (A1 = Area 1 and A2 = Area 2).
Table 3. Description of features used in this study.
Table 4. Description of the datasets according to the features used in the classification process.
Figure 2. Average accuracy of the 5-fold cross validation procedure to find the best SVM parameters for each dataset. Note: This figure shows the best (right) and worst (left) dataset result for Area 1.
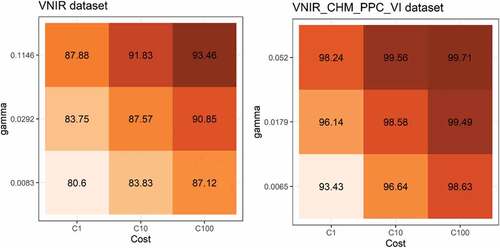
Figure 3. CNN architecture for Area 1 with 25 bands and 15 classes (14 tree species plus “background” class).
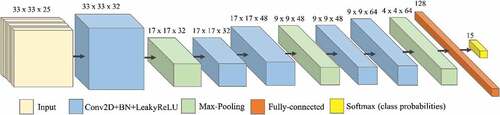
Figure 4. Variable importance according to the JM distance for each study area. The features are described in .
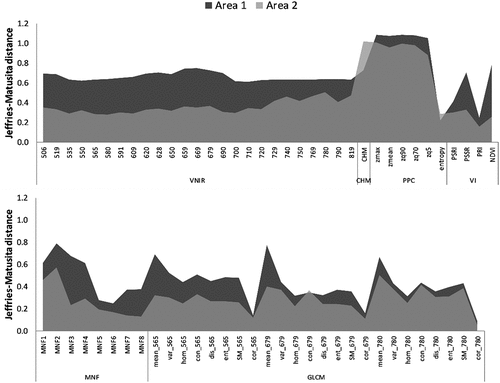
Figure 5. Mean radiance (with standard deviation) of the tree species classified in Area 1 (a) and Area 2 (b).
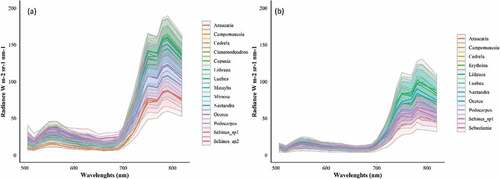
Figure 6. Boxplots showing the distribution of the PPC (zmax and zq5) and CHM values of the tree species samples in Area 1 (a and b) and Area 2 (c and d). The central lines within each box are the medians. The boxes edges represent the upper and lower quartiles. Outliers are plotted individually.
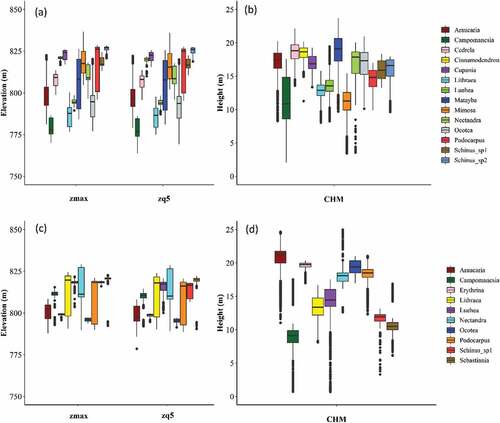
Table 5. Classification results according to the area/classifier/dataset/approach. The best result for each classifier/approach is highlighted.
Figure 7. Differences in overall accuracy with the inclusion of features and approaches in relation to the VNIR dataset, using the SVM and RF classifiers.
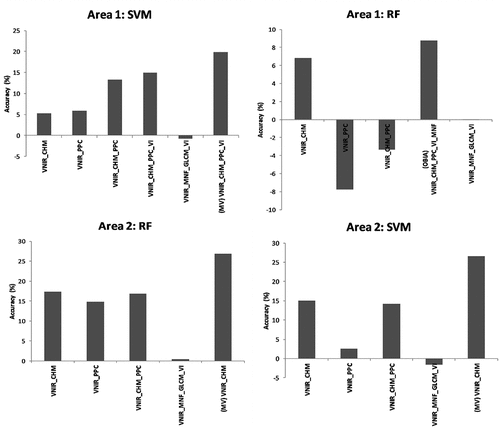
Figure 8. F-measures of each tree species class for Area 1 according to the classifier. Black bars indicate the minimum and maximum values varying according to the employed dataset.
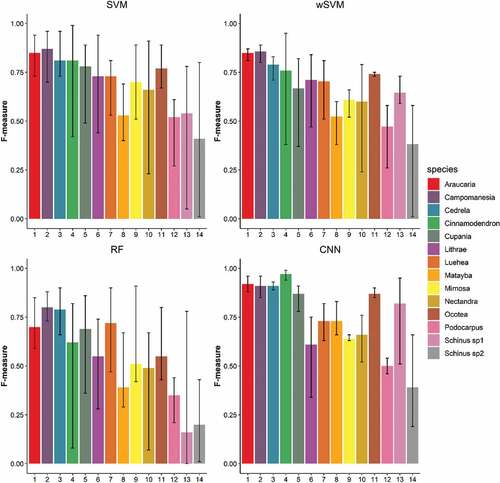
Figure 9. F-measures of each tree species class for Area 2 according to the classifier. Black bars indicate the minimum and maximum values varying according to the dataset.
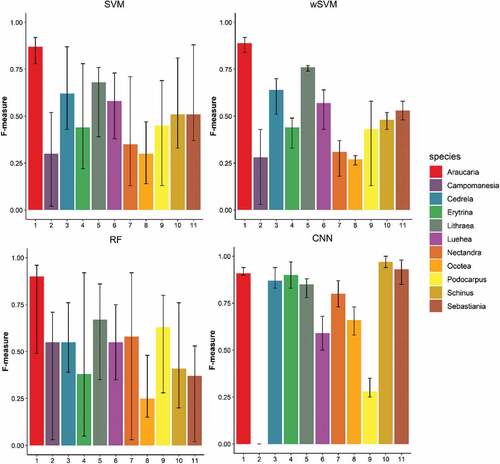
Figure 10. Some of the confusion matrices of Area 1 (top) and Area 2 (bottom). Note: Classes identified according to the species ID presented in .
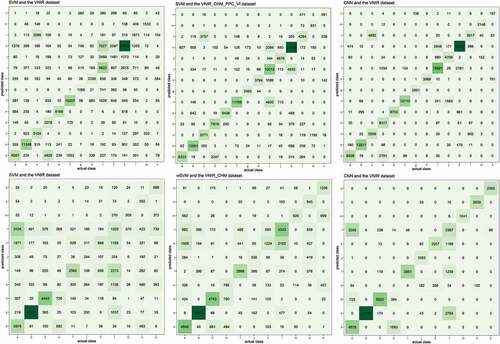
Figure 11. Examples of classification images for Area 1. a) Reference samples; b) the SVM classifier (VNIR_CHM_PPC dataset) after the MV rule – the red circle shows missing ITCs of Podocarpus; c) OBIA associated with the RF classifier and the VNIR_CHM_PPC_VI_MNF dataset – the red circle shows two missing ITCs of Nectandra and one of Podocarpus; d) the CNN classifier with the VNIR dataset – the red circle shows one missing ITC of Nectandra.
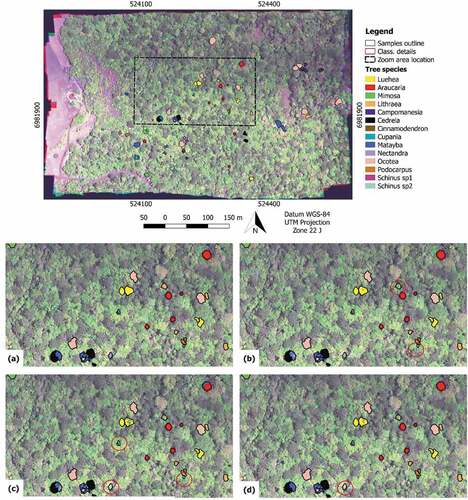