Figures & data
Table 1. The basic information of the 24 forest plots. The three coverage levels of understory vegetation are low (<18%), medium (18–34%), and high (>34%)
Figure 1. The location and distribution of sampling plots in the Bavarian Forest National Park, Germany
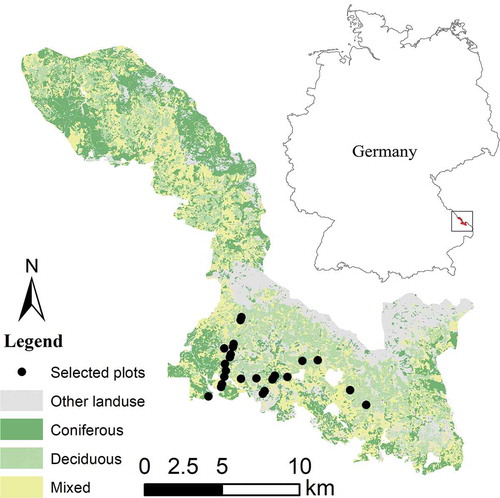
Figure 2. (a) A schematic of the sample plot design with a radius of 20 m, (b) the setup with cover board, reflector, and camera
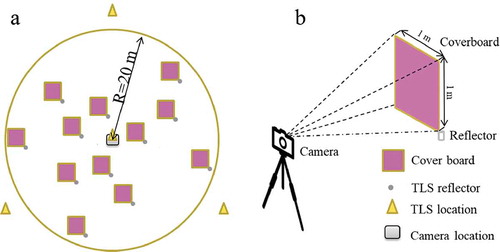
Table 2. The spectral variables used in the stepwise logistic regression to classify board versus non-board on the cover board photos
Figure 3. Illustration of occupancy grid mapping: (a) A 2D example of mapping the occupancy probabilities of the cells affected by one laser beam. The orange arrow represents a laser beam. is the distance from the point
to the TLS sensor origin.
is the i-th voxel which lies on the traveling path of the laser beam that raised the point
. Lower probabilities are encoded in white, higher probabilities in black. The unmapped cells are gray. (b) Inverse sensor model for one laser beam. Occupancy probability values for the distance measure at 5 m. (c) A 2D example of mapping the occupancy probabilities of two cells affected by several laser beams. One cell at 6 m from the TLS sensor contains five points, and another one at 10 m from the TLS sensor contains three points. The brown and green solid lines and dots represent laser beams and points, respectively. (d) The resulting occupancy probability values for the two cells in (c)
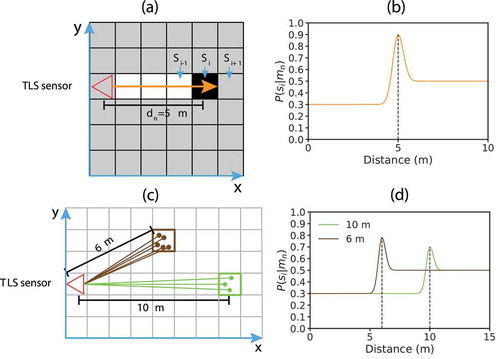
Table 3. The standard occupancy grid mapping algorithm, shown here using log-odds representations
Figure 4. Illustration of the voxelization of a sample point cloud (Plot 12) using five different voxel sizes
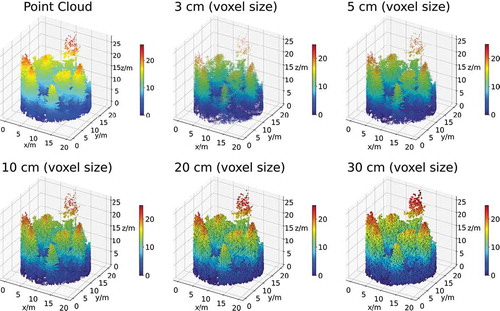
Table 4. The confusion matrix for the classification results of cover board photos
Figure 5. An example of the classification results of the cover board photos. The left, middle, and right panels correspond to the mixed, coniferous, and deciduous forest plots, respectively. In binary images, black areas indicate pixels classified as a board and white areas indicate pixels classified as a non-board
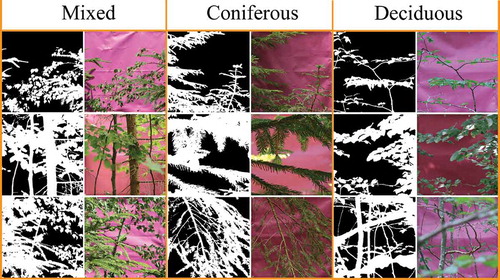
Table 5. Summary of results from a factorial ANOVA showing the effects of forest type, understory cover, voxel size, and their interaction on the agreement between photography-derived and TLS-derived visibility
Figure 6. The mean of and RMSE between photography-derived and TLS-derived visibility (a) for five different voxel sizes: 3 cm, 5 cm, 10 cm, 20 cm, 30 cm, (b) for three forest types: deciduous, coniferous, and mixed, and (c) three levels of understory cover: low, medium, and high. The different letters inside the figures indicate a statistically significant difference between different combinations of these three factors. The same letter indicates no significant difference (Tukey’s HSD test, p < 0.05)
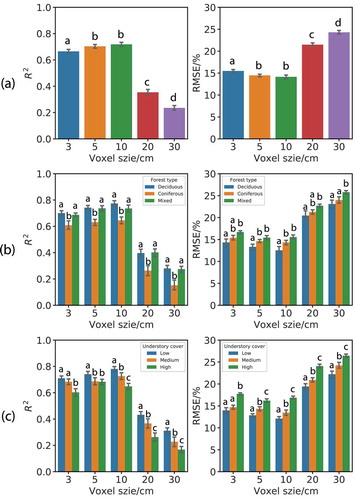
Table 6. The agreement ( and RMSE) between photography-derived and TLS-derived visibility under different forest types and understory cover conditions using a voxel size of 10 cm
Figure 7. (a) The relationship between the average ratio of unmapped voxels of all 24 plots and voxel size, (b) the average ratio of unmapped voxels of all 24 plots for different levels of understory cover given a voxel size of 10 cm
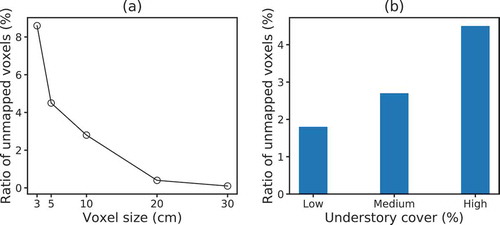
Table 7. Summary of results from a one-way ANOVA showing the effect of the traveling distance of the laser beam on the agreement between photography-derived and TLS-derived visibility