Figures & data
Figure 1. Study sites: in the top, location of the study sites in Europe and in the respective countries; in the bottom, the overviews of the two study sites (post-fire Sentinel-2 images, SWIR-NIR-Green false-color composite) where the burned areas are clearly visible (the dark-purple area in PO; the darker area in IT)
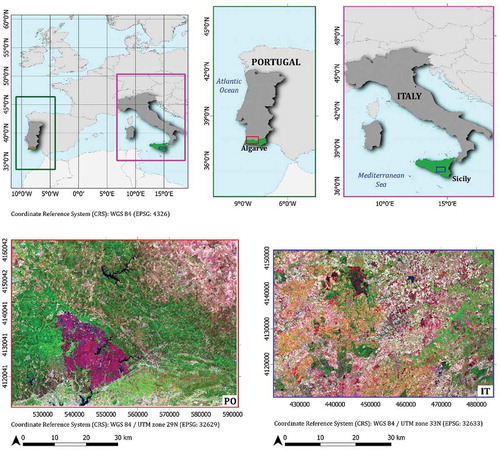
Table 1. Sentinel-1 dataset characteristics. The red line separates the images acquired before and after the fire occurrence
Table 2. Name, group, and equation of used GLCM (Grey Level Co-occurrence Matrix) texture measures. Pi,j is the probability of values i and j occurring in adjacent pixels in the original image within the window defining the neighborhood. i and j are the labels of the columns and rows (respectively) of the GLCM: i refers to the digital number value of a target pixel; j is the digital number value of its immediate neighbor. µ is mean and σ the standard deviation
Figure 3. The S-1 indices (RBDVH, LogRBRVH, ΔRVI, RBDVV, LogRBRVV, and ΔDPSVI) were obtained in the PO dataset pre-processing steps. For each of these indices, the GLCM (Grey Level Co-occurrence Matrix) texture features were calculated
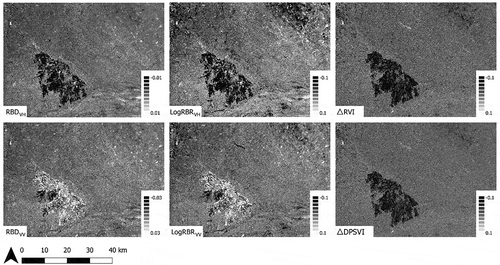
Figure 4. The S-1 indices (RBDVH, LogRBRVH, ΔRVI, RBDVV, LogRBRVV and ΔDPSVI) were obtained in the IT dataset pre-processing steps. For each of these indices, the GLCM (Grey Level Co-occurrence Matrix) texture features were calculated
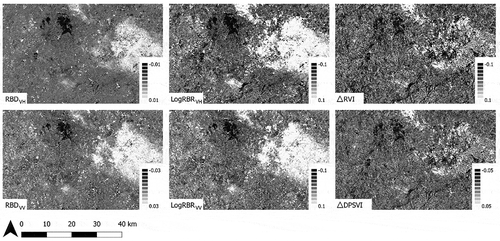
Figure 5. The cumulative variance explained by the principal components (PCs) for the PO study site. The red line identifies the first PCs that reached a cumulative variance of 0.99
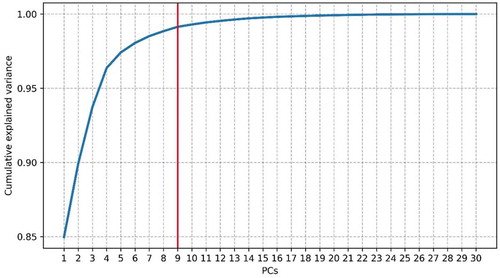
Figure 6. The cumulative variance explained by the principal components (PCs) for the IT study site. The red line identifies the first PCs that reached a cumulative variance of 0.99
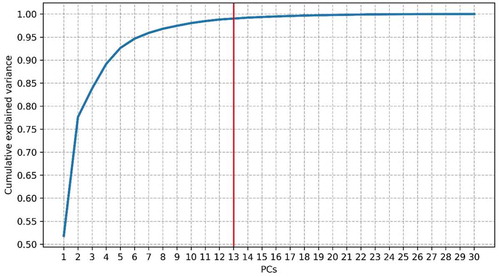
Figure 7. Silhouette score values, for the PO dataset, for a k-space range (k values) between 2 and 20
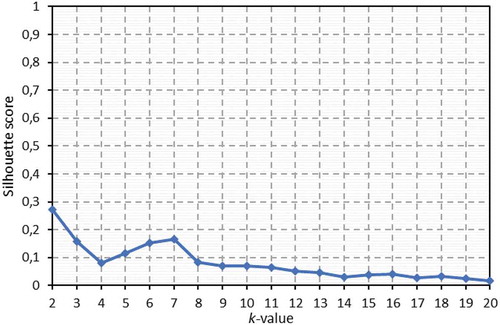
Figure 8. Silhouette score values, for the IT dataset, for a k-space range (k values) between 2 and 20
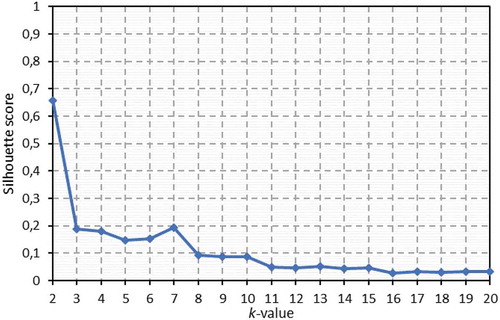
Figure 9. Classification results, showing the seven classes for both study areas. The blue clusters (classes 3–4 in the PO, and 6 in IT) represent the burned areas’ classes
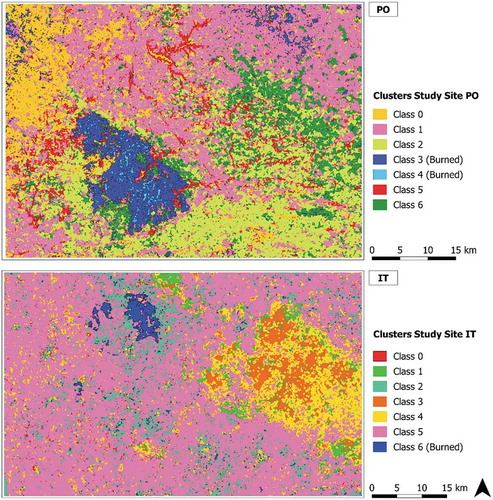
Figure 10. The figure shows the distribution of the mean value of each radar index across all six classes for both study sites (PO and IT) (at the top). At the bottom, boxplots of indices values for each class are reported (the white rhombus marker indicates the mean values)
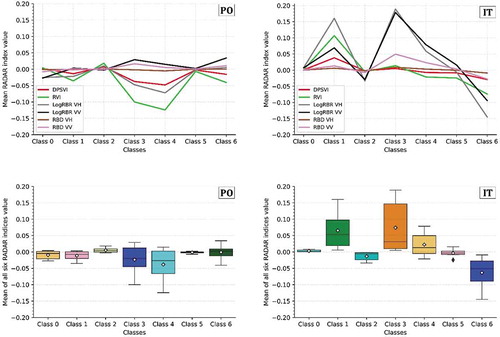
Table 3. Distribution of each dataset’s pixels and the three accuracy categories (true positive, TP; false negative, FN; false positive, FP) for both study sites (PO and IT)
Supplemental Material
Download Zip (12 MB)Data availability statement
The implemented process code is available online at https://doi.org/10.5281/zenodo.4556927.