Figures & data
Table 1. Results of farm map calibration according to levee width.
Table 2. Boundary intersection-over-union (BIOU) measured for recurrent U-Net (RU-Net) applications.
Figure 9. Correlation between PLR and error/cultivation area ratio:(a) RU-Net C at city level, (b) RU-Net C at patch level for time steps after June, (c) RU-Net D at city level and (d) patch level on October 20.
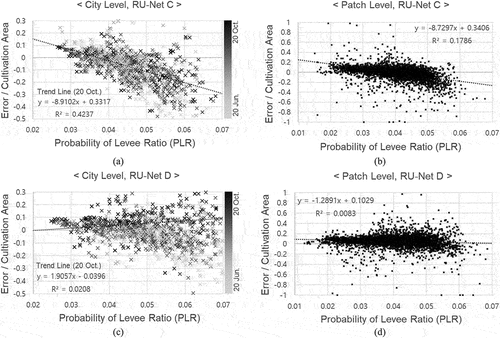
Figure 10. Correlation between national datasets and predictions: (a) Farm map to statistics, (b) Farm map to RU-Net C at city level, (c) Statistics to RU-Net C at city level, (d) Farm map to RU-Net D at city level, (e) Statistics to RU-Net D at city level, (f) Farm map to calibrated RU-Net C at city level, (g) Statistics to calibrated RU-Net C at city level, (h) Farm map to RU-Net C at patch level, and (i) Statistics to calibrated RU-Net C at patch level where paddy rice exists.
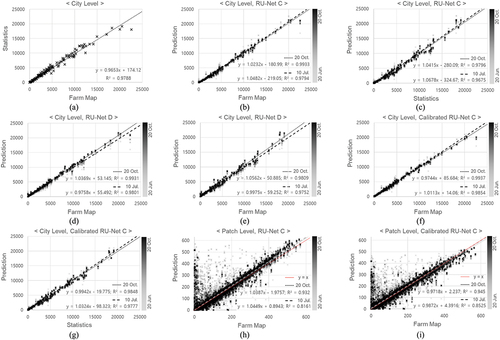
Table 3. Monthly rice prices according to harvest cycle
Table 4. Paddy rice area and expected rice yield based on different datasets.
Table A1. Confusion matrix for paddy rice mapping.
Data availability statement
Paddy rice prediction maps from 2017 to 2021 and the labeled time-series Sentinel-1 patch dataset are available in a publicly accessible repository. If these datasets are used, please cite this publication. The “Paddy Rice Maps South Korea (2017 ~ 2021)” data are available at https://github.com/Agri-Hub/Callisto-Dataset-Collection.