Figures & data
Figure 1. Overview of the proposed local-aware coupling network (LCNet). The proposed framework includes a simulated data generation module for input data and SRF calculation, an LR HSI reconstruction network designed for learning spectral feature recovery, and an HR MSI reconstruction network with spatial local-aware block for capturing key region features. The output target image is generated by the HR MSI reconstruction network.
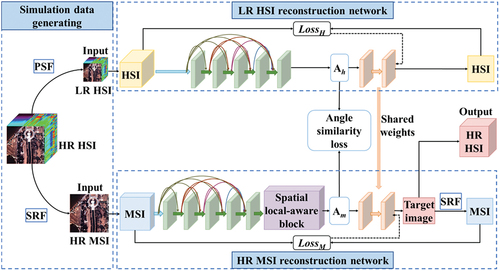
Figure 2. The spatial feature loss challenge of fusion-based HSI SR. The number of bands L in HSI is much larger than the number of basis vectors p, as well as the number of bands l in MSI. When mapping spatial features to more bands during image fusion, it is easy to cause loss of information.
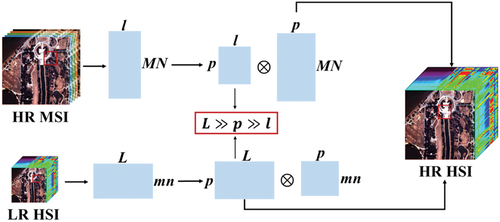
Figure 3. Spatial local-aware block. After feature extraction through a densely connected structure, the MSI is fed into the module. After pooling, convolution, and activation, a spatial attention map is obtained, which aims to focus on the texture structures of key regions.
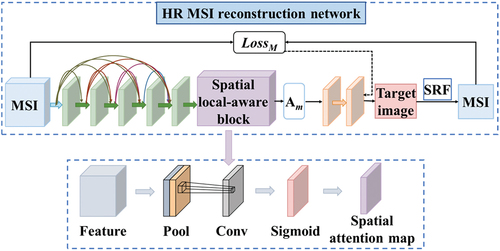
Table
Figure 4. The color-composite of three public hyperspectral datasets. (a) Indian Pines, (b) Pavia University and (c) Washington DC.
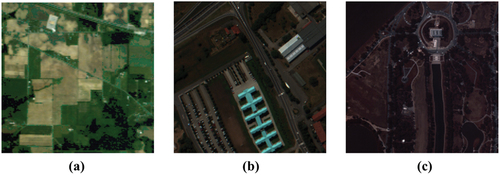
Table 1. The ability of each method to learn unknown SRF and PSF.
Figure 5. Grayscale display comparison of the 5th band of the reconstruction results on the Indian Pines data. Methods: (a) SFIM, (b) GLPHS, (c) GSA, (d) CNMF, (e) FUSE, (f) HySure, (g) CSTF, (h) uSDN, (i) LCNet, (j) Reference image.
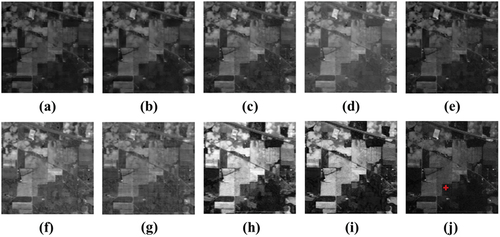
Figure 6. Comparison of the error maps of the 5th band of the reconstruction results on the Indian Pines data. Methods: (a) SFIM, (b) GLPHS, (c) GSA, (d) CNMF, (e) FUSE, (f) HySure, (g) CSTF, (h) uSDN, (i) LCNet.
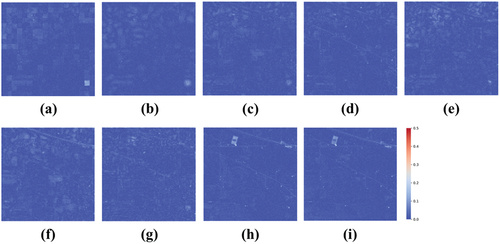
Table 2. Quantitative performance comparison of each method on the Indian Pines dataset. The best results are highlighted in bold.
Figure 8. Grayscale display comparison of the 30th band of the reconstruction results on the Pavia University data. Methods: (a) SFIM, (b) GLPHS, (c) GSA, (d) CNMF, (e) FUSE, (f) HySure, (g) CSTF, (h) uSDN, (i) LCNet, (j) Reference image.
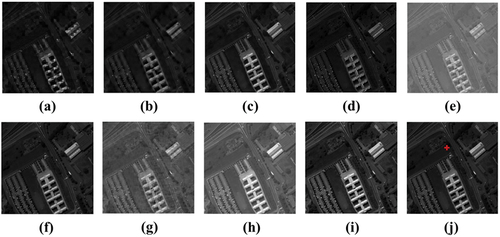
Figure 9. Comparison of the error maps of the 30th band of the reconstruction results on the Pavia University data. Methods: (a) SFIM, (b) GLPHS, (c) GSA, (d) CNMF, (e) FUSE, (f) HySure, (g) CSTF, (h) uSDN, (i) LCNet.
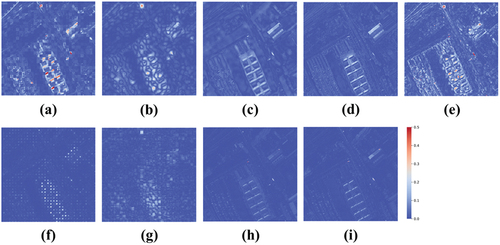
Table 3. Quantitative performance comparison of each method on the Pavia University data. The best results are highlighted in bold.
Figure 11. Grayscale display comparison of the 40th band of the reconstruction results on the Washington DC data. Methods: (a) SFIM, (b) GLPHS, (c) GSA, (d) CNMF, (e) FUSE, (f) HySure, (g) CSTF, (h) uSDN, (i) LCNet, (j) Reference image.
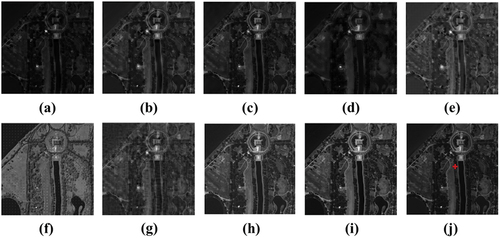
Figure 12. Comparison of the error maps of the 40th band of the reconstruction results on the Washington DC data. Methods: (a) SFIM, (b) GLPHS, (c) GSA, (d) CNMF, (e) FUSE, (f) HySure, (g) CSTF, (h) uSDN, (i) LCNet.
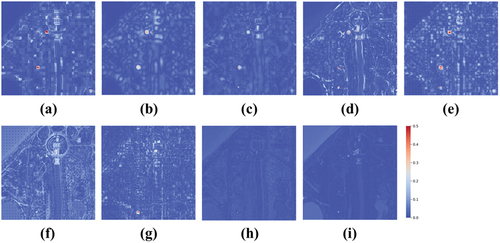
Table 4. Quantitative performance comparison of each method on the Washington DC data. The best results are highlighted in bold.
Figure 14. Grayscale display comparison of the 14th band of the reconstruction results on the real dataset. Methods: (a) LR HSI, (b) HR MSI (bands 3, 2, 1), (c) SFIM, (d) GLPHS, (e) GSA, (f) CNMF, (g) FUSE, (h) HySure, (i) uSDN, (j) LCNet.
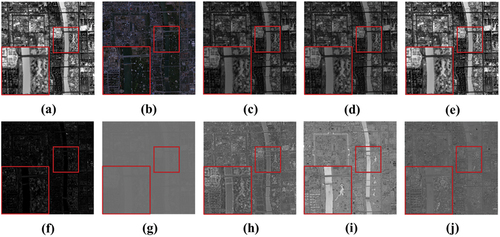
Table 5. Ablation and substitution experiment results on the Washington DC dataset. The best results are highlighted in bold.
Table 6. Parameters and FLOPs of LCNet and uSDN on the Washington DC dataset.
Data availability statement
The Indian Pines, Pavia University, and Washington DC datasets are publicly available hyperspectral image datasets. The datasets can be downloaded from the following link: https://rslab.ut.ac.ir/data. The OHS-1 hyperspectral data can be obtained from the following link can be downloaded from the following link: https://www.orbitalinsight.com/data/. The GF-2 multispectral data can be downloaded from the following link: https://data.cresda.cn/.