Figures & data
Figure 2. Locations of five national wetland reserves in China. (a) Site 1 (Guangxi Beilun Estuary National Nature Reserve), (b) Site 2 (Yancheng National Nature Reserve), (c) Site 3 (Mai Po Marshes and Inner Deep Bay), (d) Site 4 (Zhalong), (e) Site 5 (Lalu National Nature Reserve). Google Earth imagery is shown in the background.
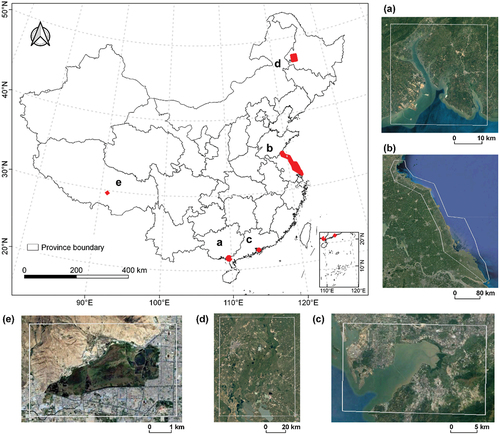
Table 1. The wetland classification system used in this study.
Table 2. The detailed information of data utilized in this study.
Figure 3. Geographical distribution of the wetland sample set over China. The pie charts show the detailed distribution of wetland and non-wetland samples among categories.
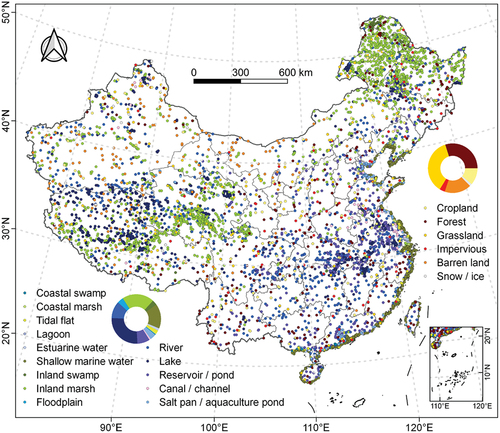
Table 3. Pixel-based features adopted for wetland classification.
Table 4. Object-based features adopted for wetland classification.
Figure 5. The architecture of ensemble learning with multi-layer stacking strategy, shown here with n types of base classifiers and 2 stack layers.
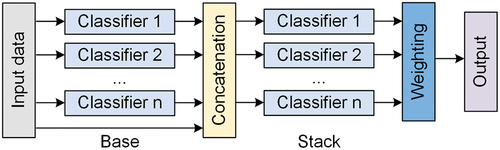
Table 5. Comparison results of different classifiers according to first-stage pixel-based classification accuracy and running time×.
Table 6. Comparison results of different classifiers according to second-stage object-based classification accuracy and running time×.
Table 7. Overall wetland classification accuracy of the proposed multi-stage method.
Figure 6. The relative importance of features for (a) pixel-based classification and (b) object-based classification. Note: explanations of feature names can be found in . Suffixes in the feature names such as p50 and p75 denote quantile values. Suffixes including diss, contrast, var, and savg, refer to dissimilarity, contrast, variation, and sum average features. The suffix ‘_4’ stands for the wettest composites. Features including latsin, latcos, lonsin, and loncos represent sine and cosine values of latitude and longitude.
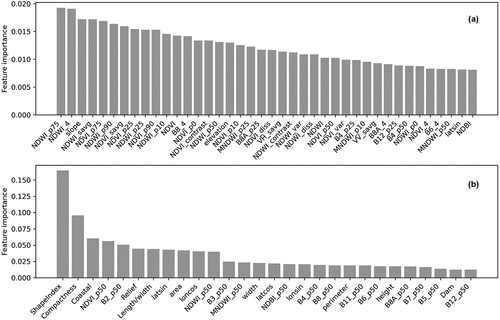
Table 8. Comparison of pixel-based classification accuracy with different combinations of features×.
Table 9. Comparison of object-based classification accuracy with different combinations of features×.
Figure 7. The wetland mapping results of five national wetland reserves in 2021. (a) Site 1, (b) Site 2, (c) Site 3, (d) Site 4, (e) Site 5. Figures in the first and second columns are Sentinel-2 and Sentinel-1 composite images in 2021, respectively.
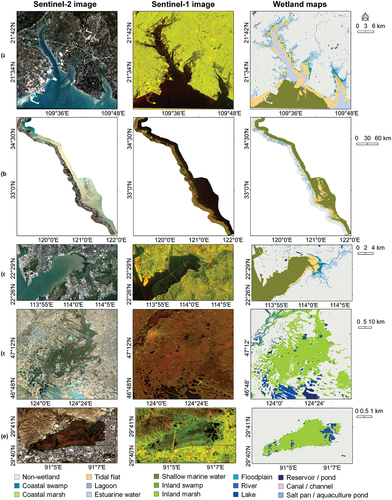
Figure 8. Comparison of global and local classifiers across five national wetland reserves using overall accuracy.
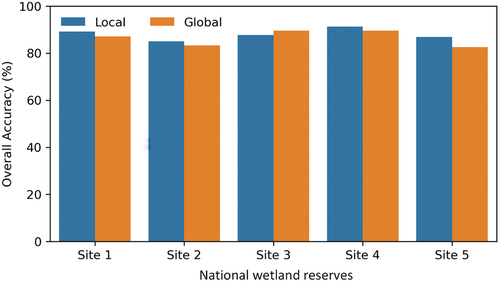
Figure 9. Comparison of our wetland mapping results, CAS_wetlands, and FROM-GLC10 in selected locations. (a) centered at 109.5°E, 21.5°N; (b) centered at 114.0°E, 22.5°N; (c) centered at 109.7°E, 21.6°N; (d) centered at 121.0°E, 33.0°N. Figures in the first column are Google Earth images.
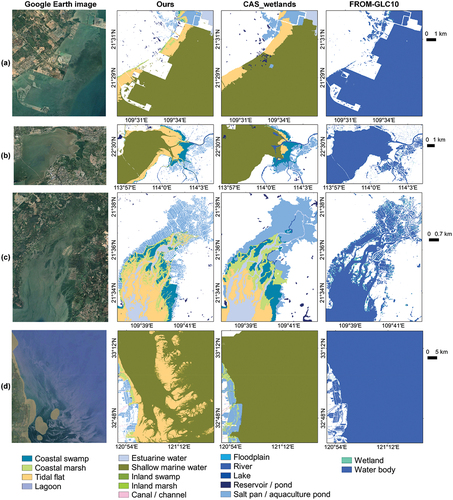
wetland_sm_20231106_highlightCleanVersion.docx
Download MS Word (709 KB)Data availability statement
The data that support the findings of this study are available upon request by contact with corresponding authors.