Figures & data
Table 1. Channel depth of each convolution block.
Figure 4. Diagram of HACM. Coverage maps of local receptive field for the three atrous convolutions with different dilation rates are presented from left to right.
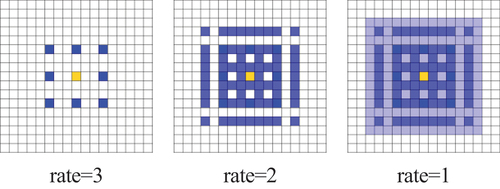
Figure 7. Diagram of overlap clipping inference. Only the first horizontal traversal is presented for better visual effects.
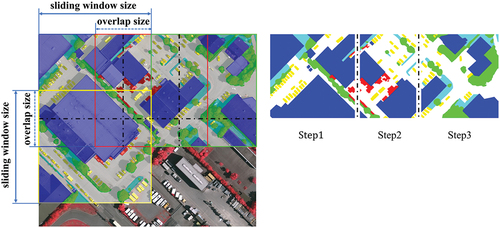
Table 2. Results of different methods on Vaihingen dataset.
Figure 8. Results for complete tiles of the Vaihingen dataset: areas 27 (top) and 31(bottom). (a) original, (b) GT, (c) FCN–8s, (d) UNet, (e) SegNet, (f) PSPNet, (g) DeepLabv3+, (h) ERN, (i) SCAttNet, (j) ResUNet-a, (k) GAMNet, (l) LANet, (m) MDANet, (n) proposed BEDSN.
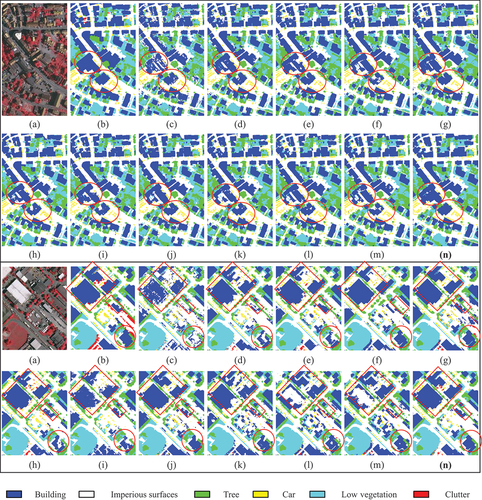
Figure 9. Results for local areas of the Vaihingen dataset. (a) original, (b) GT, (c) FCN–8s, (d) UNet, (e) SegNet, (f) PSPNet, (g) DeepLabv3+, (h) ERN, (i) SCAttNet, (j) ResUNet–a, (k) GAMNet, (l) LANet, (m) MDANet, (n) proposed BEDSN.
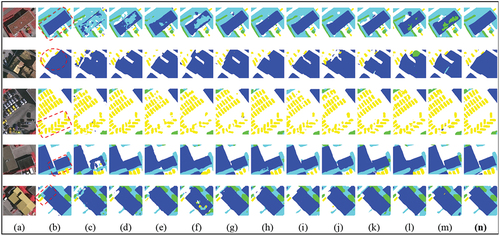
Table 3. Results of different methods on Potsdam dataset.
Figure 10. Results for complete tiles of the Potsdam dataset: areas 5_13 (top) and 5_14 (bottom). (a) original, (b) GT, (c) FCN–8s, (d) UNet, (e) SegNet, (f) PSPNet, (g) DeepLabv3+, (h) ERN, (i) SCAttNet, (j) ResUNet–a, (k) GAMNet, (l) LANet, (m) MDANet, (n) proposed BEDSN.
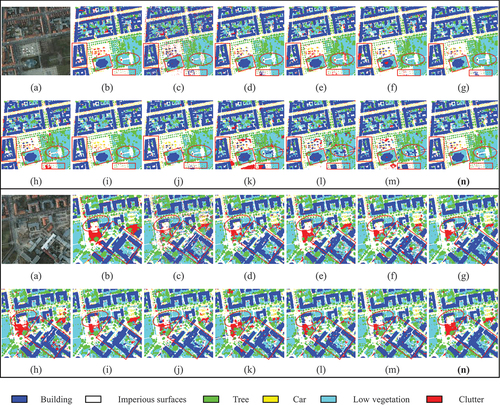
Figure 11. Results of local areas on the Potsdam dataset. (a) original, (b) GT, (c) FCN–8s, (d) UNet, (e) SegNet, (f) PSPNet, (g) DeepLabv3+, (h) ERN, (i) SCAttNet, (j) ResUNet–a, (k) GAMNet, (l) LANet, (m) MDANet, (n) proposed BEDSN.
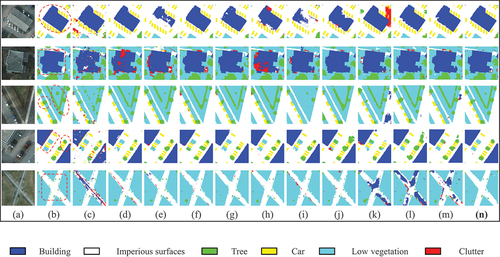
Figure 12. Results of boundary blur on the Potsdam dataset: a part of area 7_13 (a patch identification number of Potsdam dataset). (a) original, (b) GT, (c) edge, (d) dilated edge, (e) FCN–8s, (f) UNet, (g) SegNet, (h) PSPNet, (i) DeepLabv3+, (j) ERN, (k) SCAttNet, (l) ResUNet–a, (m) GAMNet, (n) LANet, (o) MDANet, (p) proposed BEDSN.
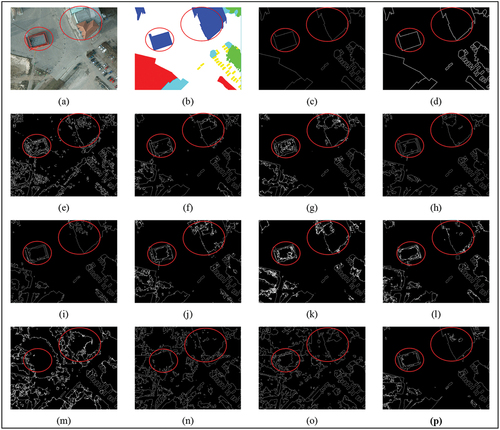
Table 4. Results of boundary metrics on Vaihingen dataset with dilated edge labels.
Table 5. Results of boundary metrics on Potsdam dataset with dilated edge labels.
Table 6. Results of ablation experiments on Vaihingen dataset.
Figure 13. Results for the improved effects of SSBS and EDBS on Vaihingen (top) and Potsdam (below) datasets. (a) Image, (b) GT, (c) UNet, (d) SSBS, (e) BEDSN-P, (f) BEDSN, (g) Boundary results of BEDSN.
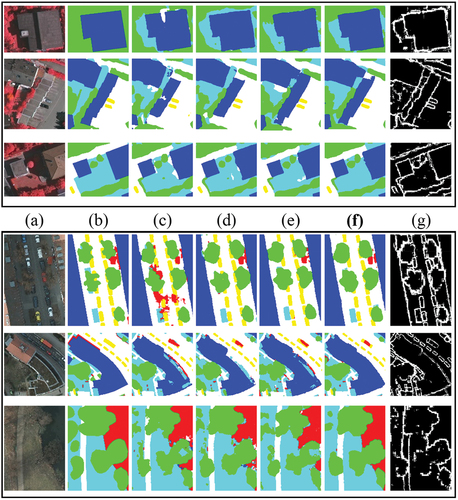
Table 7. Experiments on arrangement of SSBS, EDBS and efficiency.
Figure 14. Semantic segmentation results of different λ and loss functions. Optimal indicators are marked with red triangles.
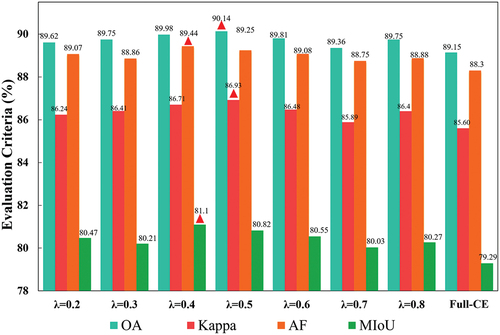
Figure 15. Results of the joint loss function and Full–CE. (a) Image, (b) GT, (c)–(d) Segmentation and boundary predictions of the joint loss function (λ = 0.5), (e)–(f) Segmentation and boundary predictions of Full–CE.
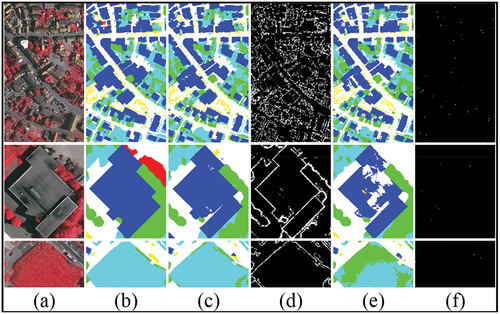
Table 8. Experiments on different batchsizes with learning rate of 3 × 10−4.
Table 9. Experiments on different learning rates with the batchsize of 8.
Data availability statement
The data that support the findings of this study are openly available in ISPRS at https://www.isprs.org/education/benchmarks/UrbanSemLab/Default.aspx. Other data that support the findings of this study including codes and results are available from the first author, [Xinghua Li, [email protected]], upon reasonable request.