Figures & data
Figure 1. Geographic location of the study area and validation plots with information from the Dominant Leaf Type 2018 forest cover layer (European Environmental Agency Citation2020) and Landsat Path/Row coverage (World Reference System 2). The base layer corresponds to the hill shade derived from the 25 m EU-DEM digital surface model.
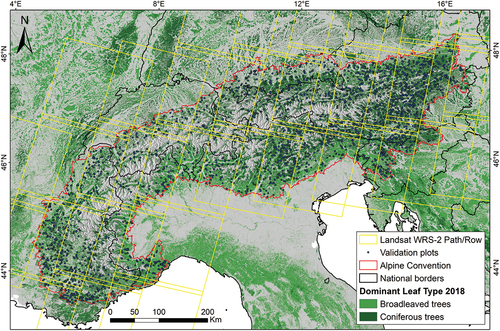
Table 1. List of the spectral indices used in this study.
Figure 2. Flowchart of the main operations performed by HILANDYN. Input data consist of four-dimensional raster cubes where bands and time form the third and fourth dimensions, respectively. A three-dimensional spatial kernel is employed on a pixel basis to build -dimensional time series with a matrix form of the dimension
. The core operations performed by HILANDYN on
-dimensional time series are described in the orange box.
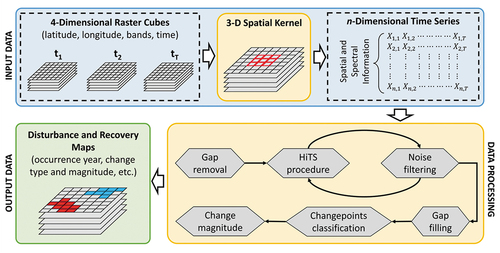
Figure 3. Examples of the spatial arrangement of disturbed pixels (orange) within the spatial kernel used by HILANDYN where the focal pixel is surrounded by non-disturbed pixels (grey).
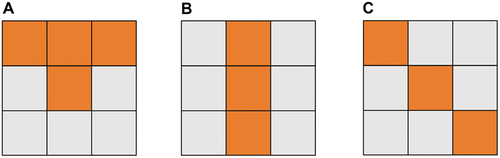
Figure 4. Example of time series of three focal pixels containing sparse noise (a), dense noise (b) or spectral changes caused by a wildfire (c). HILANDYN ignored sparse noise (a) while detected the dense ones in 1986 and 2002 (b). The wildfire occurred in 1991 (c) caused a changepoint characterised by a spike similar to impulsive noise though some bands, i.e. SWIR1, SWIR2 and TCW, did not exhibit a rapid spectral recovery.
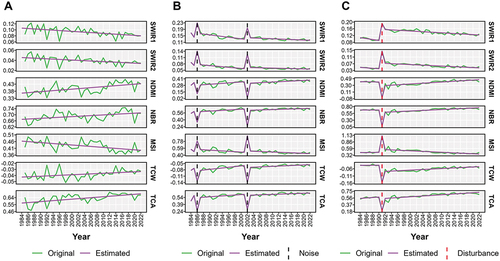
Figure 5. Diagram of the operations performed by the noise filter during the screening stage for each interval. The number of observations per year and the parameter are required for evaluating condition
. Original and estimated spectral values are required for evaluating conditions
and
. Time points at the beginning of the time series are first processed through condition
. Conditions
and
are checked on time points that exhibit the maximal spectral deviation from a reference value. Blue boxes indicate input data, black boxes indicate processing and green boxes indicate outputs.
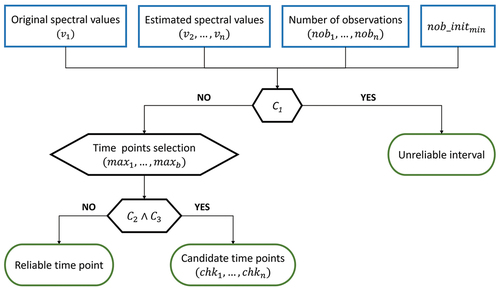
Table 2. Information on the validation dataset based on the validation strata. We discriminated between stand-replacing and non-stand-replacing events using a threshold of 50% of the relative TCW change magnitude.
Table 3. List of the main parameters required by HILANDYN and values tested for the sensitivity analysis.
Figure 6. Maximum values of user’s accuracy (UA), producer’s accuracy (PA) and F1 score based on the validation dataset and computed from all the unique combinations of bands with lengths from one to 14. The value of the constant ranged from 0.6 to 1.2. The noise filter was deactivated.
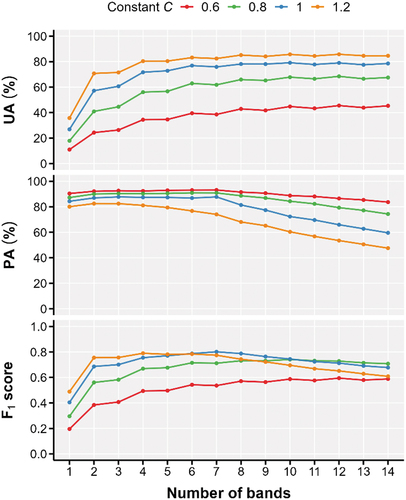
Figure 7. Distribution of values of user’s accuracy (UA), producer’s accuracy (PA) and F1 score based on the validation dataset and computed from all the unique combinations of bands with lengths from one to 14. The value of the constant factor was equal to one. The noise filter was deactivated.
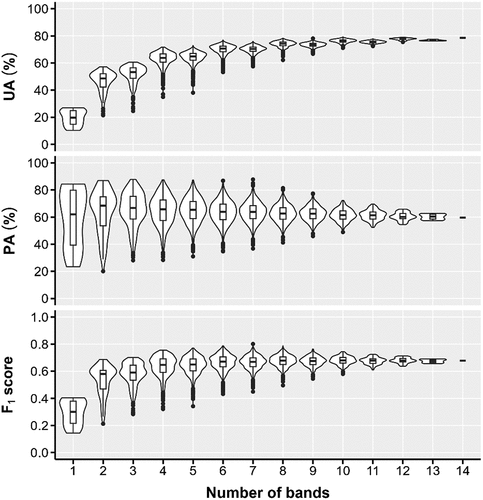
Figure 8. Frequency count relative to the number of times each band was in the best-performing combination in terms of PA, using from one to 14 bands in the high-dimensional time series. Results obtained with values of the constant factor ranging from 0.6 to 1.2 were pooled together. The noise filter was deactivated.
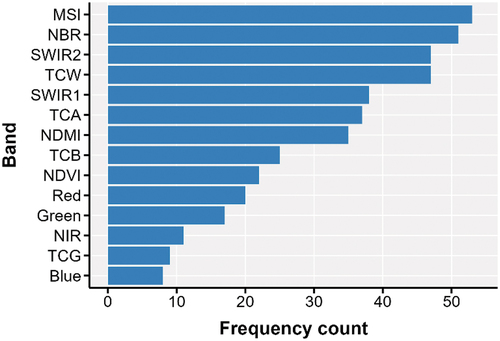
Table 4. User’s accuracy (UA), producer’s accuracy (PA), and F1 score achieved by HILANDYN with the noise filter activated or not. Accuracy metrics were computed using either both strata of the validation dataset or a single one. High-dimensional Landsat time series included data from seven bands (SWIR1, SWIR2, NDMI, NBR, MSI, TCW, and TCA) within a three-by-three spatial kernel. We parametrized the algorithm using = 1,
= 4, and
= 5.
Figure 9. Distribution of the relative TCW change magnitude of commission (100 – UA) errors and omission (100 – PA) errors for HILANDYN and BEAST. High-dimensional Landsat time series included data of seven bands (SWIR1, SWIR2, NDMI, NBR, MSI, TCW and TCA) within a three-by-three spatial kernel. We parametrised each algorithm using the settings that provided the best results in terms of F1 score. We constrained the relative TCW change magnitude between −50% and 250% by assigning these values to those outside that range.
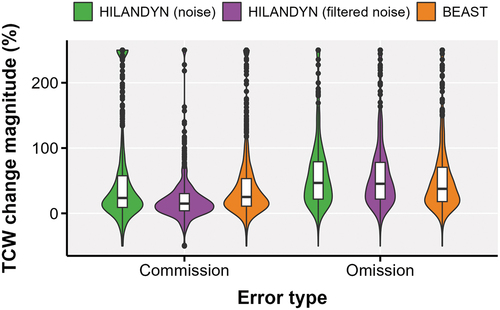
Table 5. User’s accuracy (UA), producer’s accuracy (PA), and F1 score achieved by BEAST with the validation dataset as a function of the maximum number of trend changepoints (trendMaxKnotNum) and the data in the time series. Input time series included either data of one band (NBR), or seven bands (SWIR1, SWIR2, NDMI, NBR, MSI, TCW, and TCA) of one or nine pixels, i.e. within a three-by-three spatial kernel. The highest value of each accuracy metric for every input data type is highlighted in bold.
Figure 10. Execution time required by HILANDYN and BEAST to process the 39-year high-dimensional time series of the validation dataset. The total number of variables in each time series was equal to the product of the number of bands and nine, i.e. the number of pixels within a three-by-three spatial kernel. The bands selected in each combination were those that gave the best results in terms of PA with HILANDYN when the constant was equal to one (Table S1). We configured the noise filter of HILANDYN by setting the
to four and
to five. For BEAST, we used the predefined prior parameters except for trendMaxknotnum and trendMinsepdist that we set to eight and one, respectively. Each process ran on a single core of an AMD Ryzen 9 5950X processor.
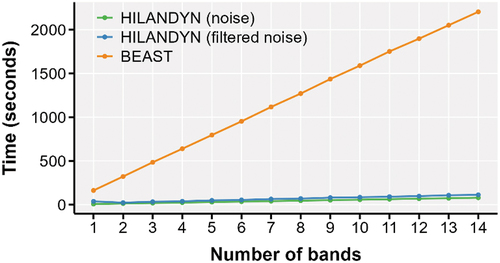
Figure 11. Examples of stand-replacing and non-stand-replacing forest disturbances detected by HILANDYN in the study area between 1985 and 2022. Panels A-F depict the following disturbance events: (a) dieback induced by defoliating insect outbreak (Asian chestnut gall wasp, Dryocosmus kuriphilus) in 2011; (b) dieback caused by a severe drought and heatwave in 2003; (c) wildfires in 1990, 1991, 2003, and 2017; (d) windthrow in 2007; (e) ice storm in 2014; (f) windthrow in 2018. The grey background in the central panel indicates the presence of forest cover either in 1990 or 2018 according to the Corine Land Cover within the European Alps borders (blue line).
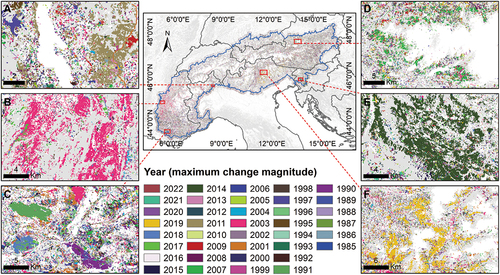
Figure 12. Examples of disturbed forest patches detected by HILANDYN and characterised by different disturbance agents and severities. Please refer to the legend in for the colour corresponding to each year in panels a-e. Clearcuts (a, f; 5°45‘18,102“E; 44°3’9,791“N), debris flows (b, g; 8°1‘54,389“E; 46°46’4,057“N), defoliating insect outbreak (c, h; 7°35‘10,709“E; 45°22’18,178“N), wildfire (d, i; 13°16‘54,707“E; 46°28’25,202“N), windthrow (e, j; 7°21‘17,61“E; 44°39’31,641“N). The grey background indicates the presence of forest cover either in 1990 or 2018 according to the Corine Land Cover.
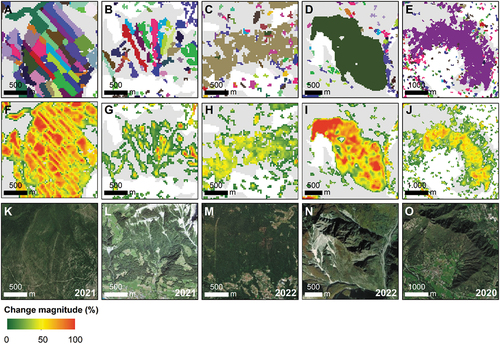
Figure 13. Examples of forest patches, depicted in yellow, that were disturbed multiple times during the analysis period. Each row shows results from HILANDYN, recent high-resolution satellite imagery, and temporal profiles of NBR and TCW. We parametrised the algorithm according to the configuration that achieved the highest F1 score (Section 3.1). Recurrent wildfires (a-d; 7° 9’1.02“E; 45° 9’35.57“N). Wildfire followed by salvage logging (e-h; 9°39’23.26“E; 46°11’59.51“N). Bark beetle attack followed by salvage logging (i-l; 14°42’0.63“E; 46°16’1.43“N). Windthrow followed by salvage logging (m-p; 11°21’13.93“E; 46°17’41.66“N).
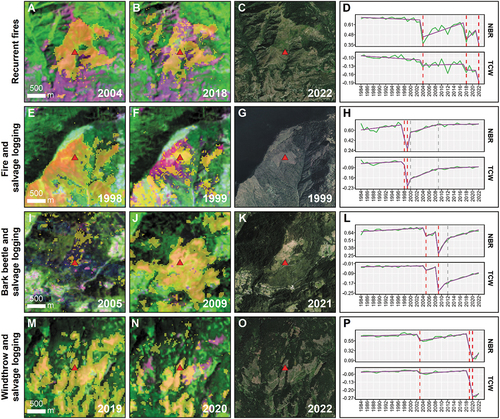
Supplementary material R1.docx
Download MS Word (285.1 KB)Data availability statement
The code of the algorithm is implemented in the R package “hilandyn” publicly available via the GitHub repository of the first author at https://github.com/donatomorresi/hilandyn.