Figures & data
Figure 1. Reinforcement learning.[Citation22]
![Figure 1. Reinforcement learning.[Citation22]](/cms/asset/1cd174e2-3e20-4e5f-a366-fa054b4081cb/uopt_a_2222146_f0001_c.jpg)
Table 1. Parameters of gait patterns.
Figure 9. Changes of gait parameters when the robot is walking. (a) Gait parameters without PPO controller and (b) Gait parameters when PPO controller is operating.
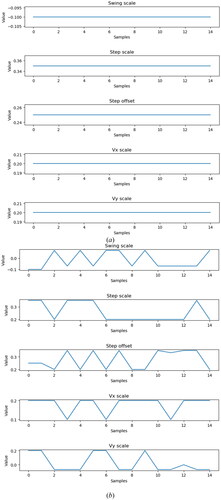
Table 2. Parameters of PPO2.
Figure 17. Acceleration record in the x, y, and z directions processed by the unprocessed sensor data.
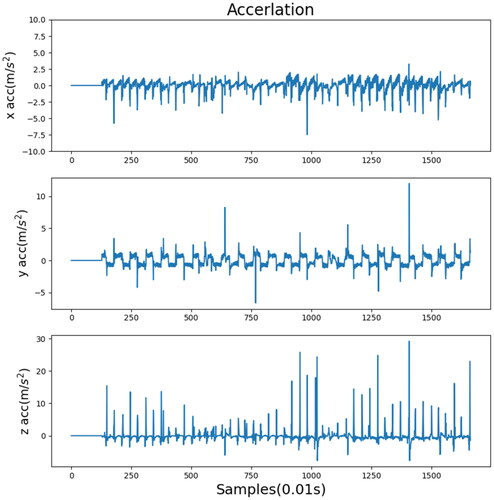
Figure 19. Acceleration record in the x, y, and z directions processed by the type A fuzzy membership function.
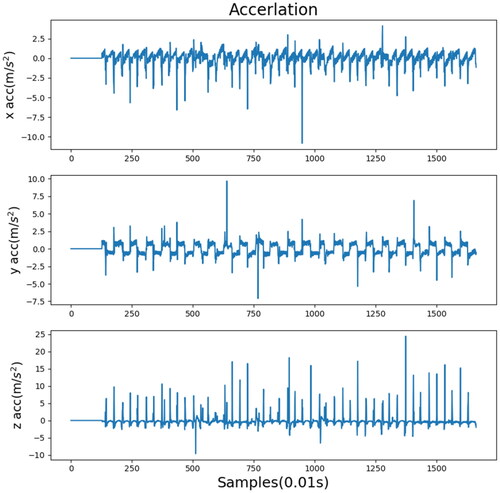
Figure 20. Acceleration record in the x, y, and z directions processed by the type B fuzzy membership function.
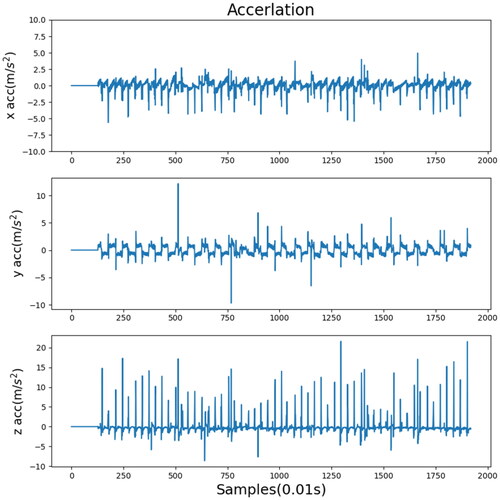
Table 3. Standard deviation of the PPO2 training results.
Table 4. Standard deviation of the TRPO training results.
Table 5. Standard deviation of the A2C training results.