Figures & data
Fig. 1. (a) Map of the 56 regions whose monthly emission budgets are controlled by the inversion; (b) zoom on the 17 control regions in Europe; (c) zoom on the 11 control regions in the United States; (d) zoom on the 10 control regions in China.
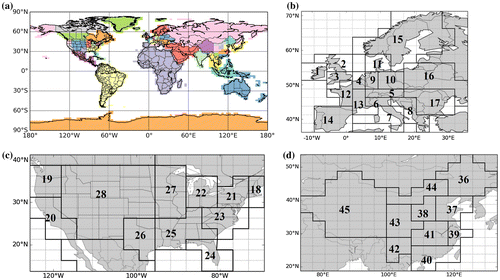
Fig. 3. Distribution of urban pixels (defined by population density, section 2.1.2) over Europe at 0.5° resolution (a) and maps of the RMS of the 1-year long time series of the representation errors εr (at 0.5° resolution) (b) εa (at 3.75°× 2.5° resolution) (c) and the prior FFCO2 errors Hεb (at 3.75°× 2.5° resolution) (d) for 2-week mean afternoon FFCO2 gradients (from 100 magl sites to the JFJ reference site) (unit: ppm). In (a), the triangles give the location of the sites of a typical continental observation network similar to ICOS (ICOS, 2008; 2013); blue triangles means that the stations are in ‘rural’ pixels, while yellow triangles means the stations fall in ‘urban’ pixels.
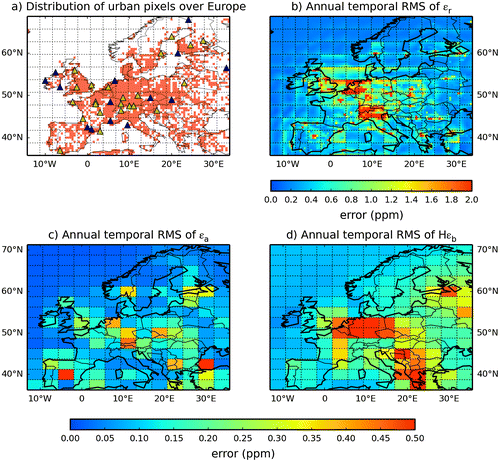
Fig. 4. Time series of the spatial RMS of the urban and rural representation errors (a), of the aggregation errors (b) and of the prior FFCO2 errors (c) for 2-week mean afternoon FFCO2 gradients (from 100 magl sites to the JFJ reference site) (unit: ppm).
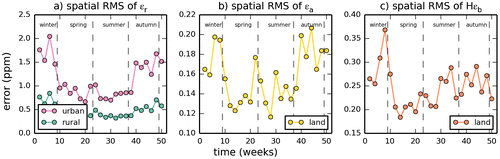
Fig. 5. Probability density functions (PDFs) of the representation, aggregation and prior FFCO2 errors for 2-week mean afternoon gradients (from 100 magl sites to the JFJ reference site) for nearly all of the categories defined by sections 4.1 and 4.2 (only PDFs in spring and fall for urban and rural representation errors and for the prior FFCO2 errors are not shown). The theoretical fit of these PDFs with Gaussian distributions (yellow dash lines) in terms of mean (μ) and standard deviations (σ), and the theoretical fit of these PDFs with Cauchy distributions (red dash lines) in terms of location parameter (x0) and scale factor (γ) are also reported on the graphs.
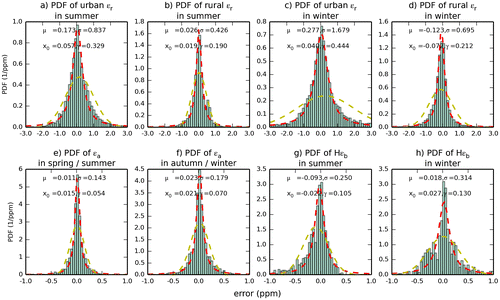
Table 1. Standard deviations (in ppm) of the different categories of representation, aggregation and prior FFCO2 errors for the 2-week mean afternoon FFCO2 gradients and seasonal RMS (in ppm) of the FFCO2 gradients between all potential rural or urban locations of 100 magl continental sites and JFJ and over all time periods during each season as simulated at 0.5° resolution when using CHIMERE and the EDG-IER inventory (i.e. our practical representation of the true gradients
).
Table 2. The parameters optimized by the regressions of the temporal auto-correlations of the representation, aggregation and prior FFCO2 errors for 2-week and 1-day mean afternoon FFCO2 gradients (from all the potential 100 magl sites to JFJ), using e-folding functions r(Δt) = a × e−Δt/b + (1 − a) × e−Δt/c and ignoring the different temporal categories.
Fig. 6. Estimates of the correlations for the different spatial categories of representation errors (urban εr in purple and rural εr in green), aggregation errors (in yellow) and prior FFCO2 errors (in red) for 2-week mean afternoon FFCO2 gradients (from all the potential 100 magl sites to JFJ), ignoring the different temporal categories (i.e. mixing errors from all seasons and thus computing temporal auto-correlations between errors across different seasons or using a temporal sampling across different seasons to compute spatial correlations). (a) Temporal auto-correlations. Dots correspond to the estimates of the temporal auto-correlations. Lines correspond to regression curves with e-folding functions r(Δt) = a × e−Δt/b + (1 − a) × e−Δt/c, where Δt is the timelag (in days), and where a, b and c are the parameters optimized by the regression. (b) Spatial correlations. The spatial correlations between urban and rural representation errors (in brown) are given along with the correlations within the different categories of errors.
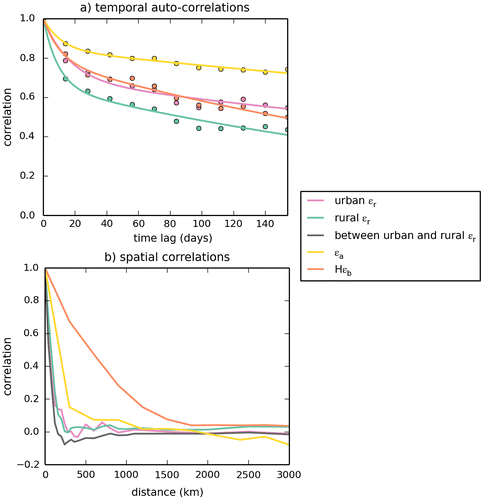
Fig. 7. Standard deviations (SDs) of all the occurrences of the representation, aggregation and prior FFCO2 errors for specific categories of 2-week mean afternoon FFCO2 gradients, as a function of the sampling height above ground (unit: ppm).
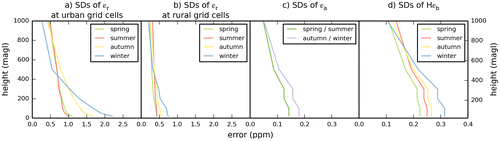
Fig. 8. Standard deviations (SDs) of all the occurrences of the representation, aggregation and prior FFCO2 errors for 1-day to 1-month mean afternoon FFCO2 gradients between 100 magl sites and the JFJ reference site (unit: ppm), and RMS of the simulated gradients at 0.5° resolution when using CHIMERE and the EDG-IER inventory (i.e. our practical representation of HHR →HRt). The sampling durations are expressed in days. Dots correspond to the estimates of the standard deviations of the errors and of the quadratic mean of the simulated gradients. Lines correspond to regression curves with e-folding functions ε(l) = ε(1) × [a × e−(l−1)/b + (1 − a) × e−(l−1)/c], where l is the duration (in days) of the mean afternoon sampling, ε(1) is the standard deviations of the errors (or simulated gradients) for 1-day sampling, and where a, b and c are the parameters optimized by the regressions. Results for (a) urban εr; (b) rural εr; (c) εa; (d) Hεb; (e) simulated gradients for urban grid cells; (f) simulated gradients for rural grid cells.
![Fig. 8. Standard deviations (SDs) of all the occurrences of the representation, aggregation and prior FFCO2 errors for 1-day to 1-month mean afternoon FFCO2 gradients between 100 magl sites and the JFJ reference site (unit: ppm), and RMS of the simulated gradients at 0.5° resolution when using CHIMERE and the EDG-IER inventory (i.e. our practical representation of HHR →HRt). The sampling durations are expressed in days. Dots correspond to the estimates of the standard deviations of the errors and of the quadratic mean of the simulated gradients. Lines correspond to regression curves with e-folding functions ε(l) = ε(1) × [a × e−(l−1)/b + (1 − a) × e−(l−1)/c], where l is the duration (in days) of the mean afternoon sampling, ε(1) is the standard deviations of the errors (or simulated gradients) for 1-day sampling, and where a, b and c are the parameters optimized by the regressions. Results for (a) urban εr; (b) rural εr; (c) εa; (d) Hεb; (e) simulated gradients for urban grid cells; (f) simulated gradients for rural grid cells.](/cms/asset/42944513-5eac-40e1-9788-2bdc50b5b9da/zelb_a_1325723_f0008_oc.gif)
Table 3. The parameters optimized by the regressions of the standard deviations of the representation, aggregation and prior FFCO2 errors as functions of the temporal sampling of the observations, for 1-day to 1-month mean afternoon FFCO2 gradients between 100 magl sites and the JFJ reference site (unit: ppm), and RMS of the simulated gradients at 0.5° resolution when using CHIMERE and the EDG-IER inventory (i.e. our practical representation of
).
Fig. 9. Temporal auto-correlations of the representation (urban εr in purple and rural εr in green), aggregation (in yellow) and prior FFCO2 errors (in red) for 1-day mean afternoon FFCO2 gradients (from all the potential 100 magl sites to JFJ), ignoring the different temporal categories (i.e. mixing errors from all seasons and thus computing temporal auto-correlations between errors across different seasons).
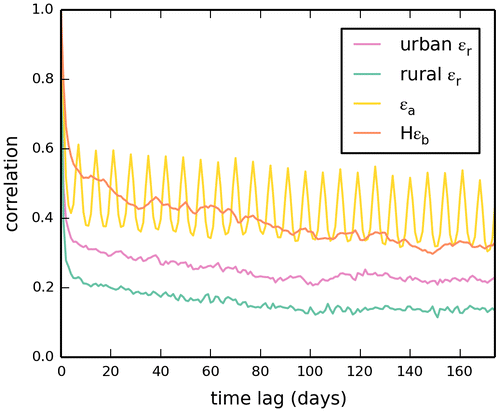