Figures & data
Figure 1. Overview of approach for training and testing a decision tree model for predicting the level of antibody viscosity for IgG1s and identifying mutations that reduce viscosity.
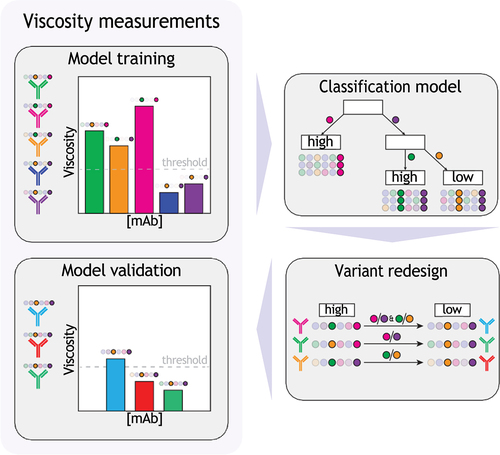
Figure 2. Physicochemical properties and germlines for the panels of 79 IgG1s in this study and 94 clinical-stage IgG1s. (a-d) Distributions of Fv (a) net charge (pH 5.2), (b) isoelectric point, (c) charge symmetry (pH 5.2) and (d) hydrophobicity for IgGs in this study (blue) and clinical-stage antibodies (yellow). (e) Distribution of germline families for IgGs in this study (blue) and clinical-stage antibodies (yellow).
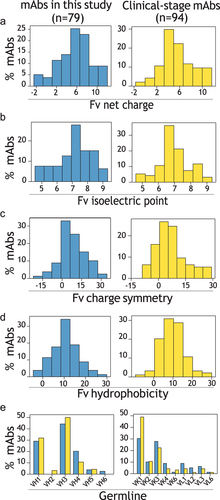
Figure 3. Analysis of the antibody Fv molecular features linked to high antibody viscosity. (a) Spearman’s values for the correlations between Fv and CDR features and antibody viscosity. (b-d) Specific examples of correlations between antibody Fv properties and antibody viscosity, including (b) Fv isoelectric point, (c) largest hydrophobic patch area in Fv, and (d) # of negative patches in Fv.
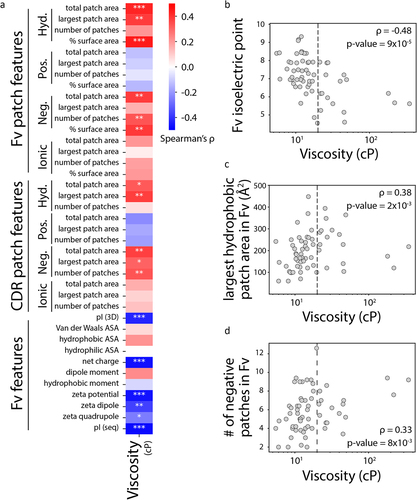
Figure 4. Decision tree model for predicting antibodies with low (<20 cP) or high (>20 cP) viscosity at pH 5.2. (a) The model was trained using a set of 62 mAbs, which includes 45 mAbs with high viscosity and 17 mAbs with low viscosity. mAbs with Fv isoelectric points <6.3 were predicted to display high viscosity (Type III antibodies). Moreover, for those with isoelectric points >6.3, mAbs with the largest hydrophobic patch in Fv <261 ÅCitation2 were predicted to display low viscosity (Type I antibodies). Finally, for those with the largest hydrophobic patch in Fv >261 Å,Citation2 mAbs with <5.8 negative patches in Fv were predicted to display high viscosity (Type IV antibodies) and low viscosity for those with >5.8 negative patches in Fv (Type II antibodies). (b-c) The four types of antibodies are separated by their three properties (and the corresponding cutoffs) from the decision tree in (a), which are shown for pairs of Fv properties, namely (b) isoelectric point versus largest hydrophobic patch and (c) isoelectric point versus number of negative patches.
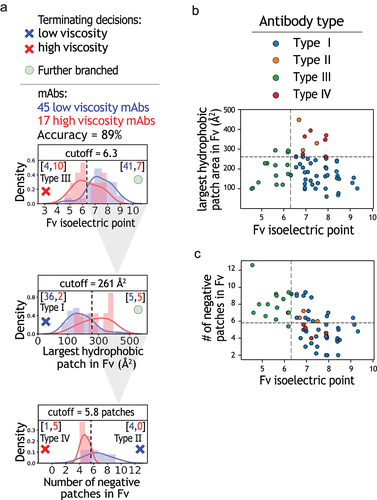
Figure 5. Evaluation of decision tree model for predicting antibodies with low or high viscosity using a hold-out set of antibodies. The model in was tested with a hold-out set of 17 mAbs, which includes 11 mAbs with low viscosity and 6 mAbs with high viscosity. The hold-out set of antibodies was not used during model training.
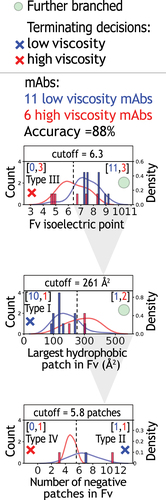
Figure 6. Evaluation of decision tree model performance relative to the performance of single features and previously reported models. (a-d) The performance of the (a) model in this work for the 79 mAbs was compared to single feature models, including (b) Fv pI, (c) largest Fv hydrophobic patch, and (d) # of negative patches in Fv. In addition, the performance of two additional previously reported models is shown, including (e) model #2 (Sharma, Patapoff et al., PNAS, 2014) and (f) model #3 (Lai, Swan et al., mAbs, 2021).
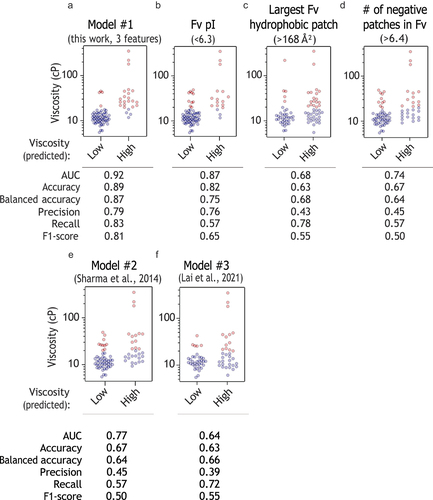
Figure 7. Design of mutations that reduce antibody viscosity. (a-b) Mutations were designed using the decision tree model for two antibodies (A24 and A54), and (c-d) tested experimentally at 150 mg/mL. In (a), the feature values are shown for the wild-type antibodies as orange (A24) and green (A54) solid lines.

Figure 8. Evaluation of viscosity model predictions and antibody properties for clinical-stage mAbs relative to the mAbs in this study. (a) The percentage of mAbs with each type of predicted viscosity behavior, as defined in Fig. 4. (b-c) Distribution of (b) Fv pI and (c) Fv net charge at pH 5.2 for different levels of predicted viscosity. In (a), the number of mAbs is shown on top of each bar.
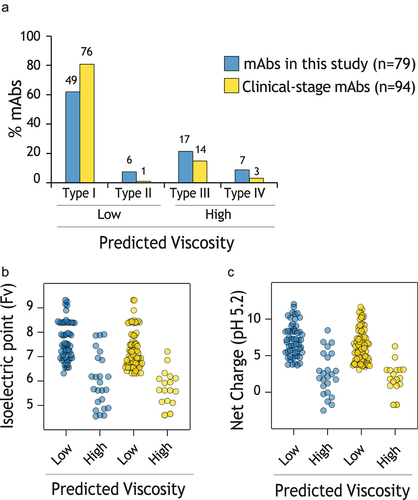