Figures & data
Table 1. Description of the collected dataset.
Figure 2. Molecular images in the dataset categorized as sweet and non-sweet (bitter, tasteless) compounds.
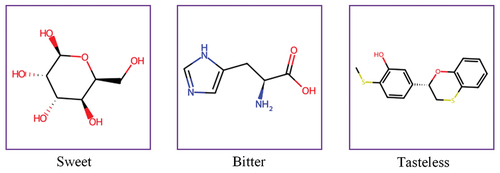
Table 2. The architecture of ResNet-50.
Table 3. Parameter setting.
Figure 7. Comparing the AUROC values of the transfer learning model ResNet-50 with different attention mechanisms.
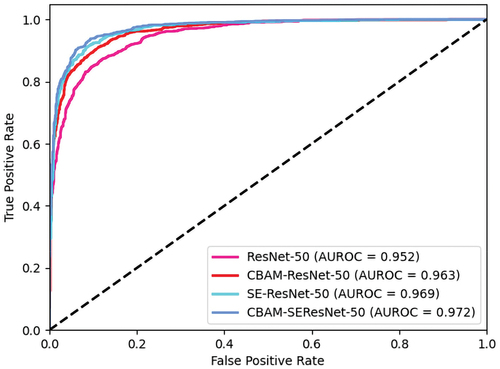
Table 4. Comparison of the transfer learning model ResNet-50 with different attention mechanisms.
Figure 9. Comparison of AUROC values for different transfer learning driven sweetener identification algorithms.
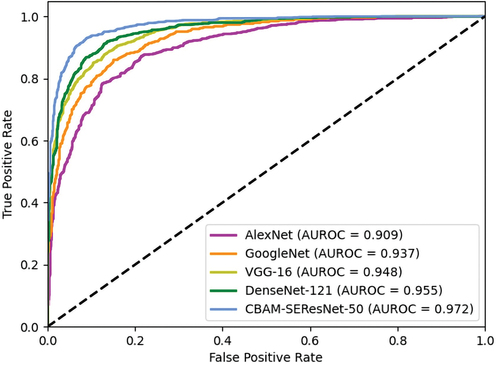
Table 5. Comparison of different transfer learning driven sweetener identification models.
Table 6. Comparison with previous sweetener identification methods.
Figure 10. Visualization of the convolutional layers, maximum-pooling layers, and residual layers in the CBAM-SEResNet-50 model.
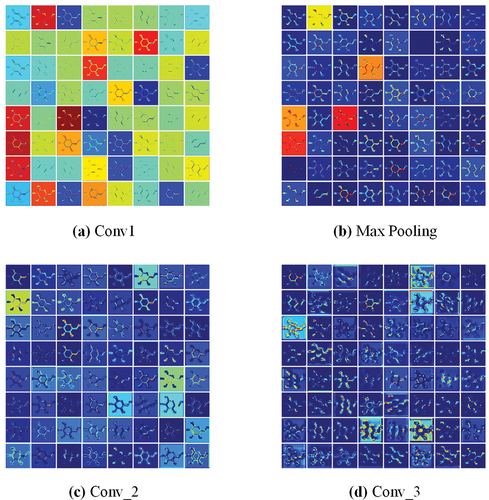
Table 7. The similarity threshold, the number of compounds outside AD for each threshold, and the corresponding AUROC value.
Table 8. Sweetener identification applications of CBAM-SEResNet-50 on different datasets.
Table 9. The identification results of the CBAM-SEResNet-50 model on a multi-taste molecule dataset.