Figures & data
Figure 1. Wind turbine gearbox vibration-based fault diagnosis: (a) Traditional vibration pre-processing framework (b) Autoencoder vibration pre-processing framework.
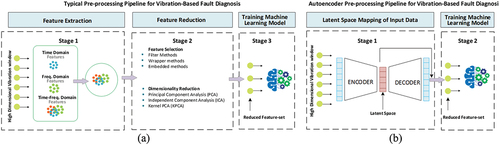
Figure 2. Vibration waveform of the intermediate speed shaft bearing: (a) Damaged signal (b) Normal signal.
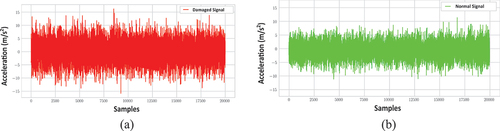
Figure 3. Vibration spectrum of the intermediate speed shaft bearing: (a) Damaged signal (b) Normal signal.
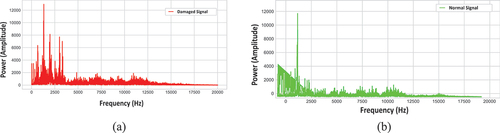
Figure 4. The waveform (a) and spectrum (b) of the intermediate speed shaft bearing’s normal vibration signals superimposed upon the damaged.
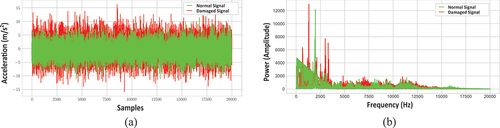
Table 1. The experimented Autoencoder model architectures.
Table 2. The Autoencoder model training hyperparameters.
Table 3. Parameters of the genetic algorithm.
Figure 10. (a) Impact of the autoencoder latent space dimension size on the LDA model’s (a) performance metrics (b) accuracy and computational time.
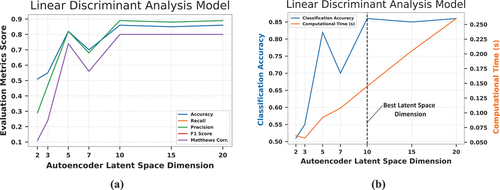
Figure 11. (a) Impact of the autoencoder latent space dimension size on the MLP model’s (a) performance metrics (b) accuracy and computational time.
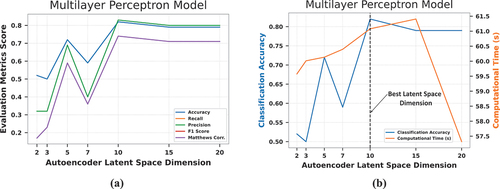
Figure 12. (a) Impact of the autoencoder latent space dimension size on the RF model’s (a) performance metrics (b) accuracy and computational time.
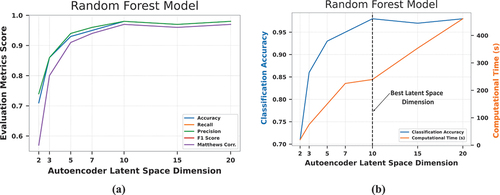
Figure 14. (a) Confusion matrix of the AE-Pre-GO-LDA model (b) confusion matrix of the AE-Pre-GO-MLP model (c) confusion matrix of the AE-Pre-GO-RF model (d) comparison of the computation time of the AE-Pre-GO-LDA, AE-Pre-GO-MLP and AE-Pre-GO-RF.
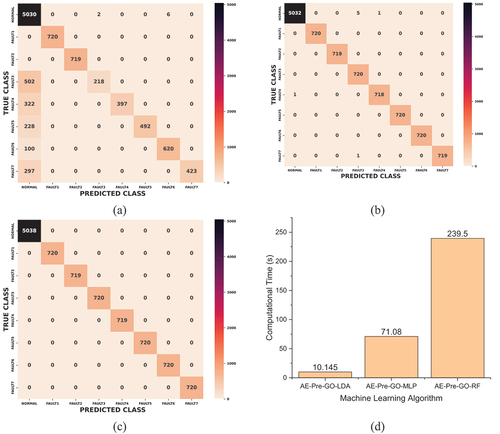
Table 4. Performance of the LDA, MLP and RF models based on the optimized hyperparameters.
Table 5. Comparison of wind turbine studies employing conventional methods for pre-processing gearbox vibration signals.