Figures & data
Table 1. Comparative analysis among various methods with the ML models
Table 2. Prediction properties using different approaches (Ahmad, Ostrowski, et al., Citation2021)
Table 3. Experimental dataset
Table 4. Parameters specification to develop model
Table 5. Descriptive analysis of the input and output variables
Table 6. Outlier analysis of variables
Figure 7. (a). The density curves of variables at X and Y axis: Cement, water, CNFs, and superplasticizers.
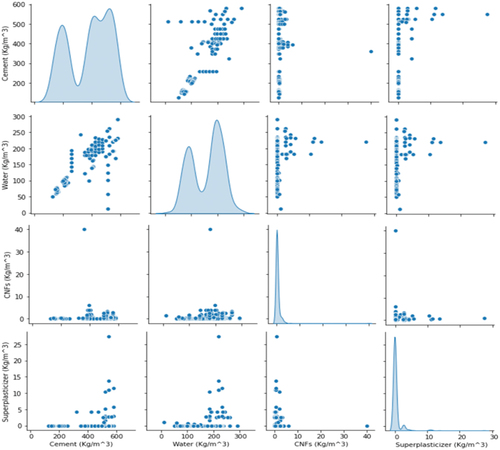
Figure 7. (b). The density curves of variables at X axis: Cement, Water, CNFs, and Superplasticizers; and Y axis: Fine aggregate, coarse aggregate, age and compressive strength fc.
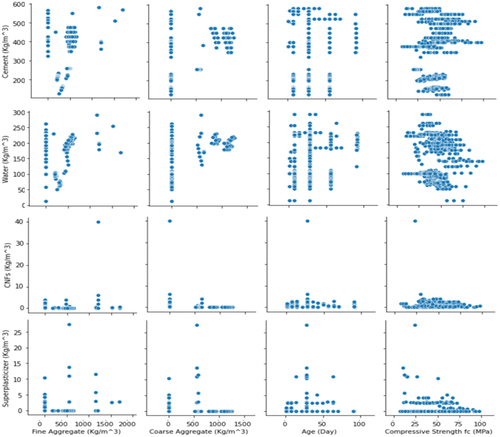
Figure 7. (c). The density curves of variables at X axis: Fine aggregate, coarse aggregate, age and compressive strength fc; and Y axis: Cement, water, CNFs, and superplasticizers.
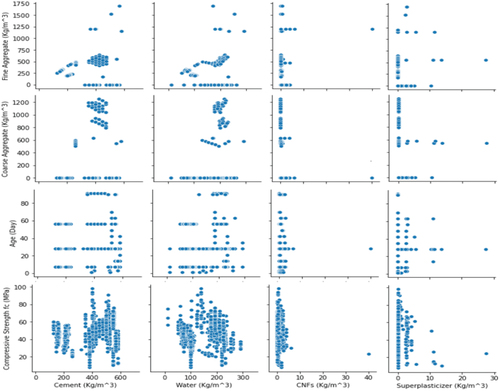
Figure 7. (d). The density curves of variables at X and Y axis: Fine aggregate, coarse aggregate, age and compressive strength fc.
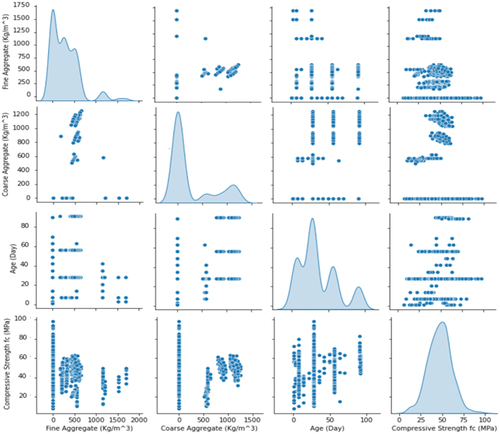
Table 7. Capability metrics of the supervised regression ML models
Figure 9. Scatter plot of R2 between the actual fc and predicted fc by (a) RF, (b) BR, (c) XGBR, and (d) DT models..
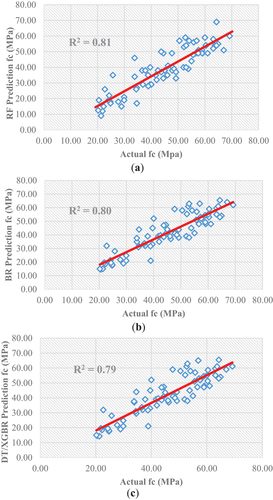
Figure 10. Scatter plot of R2 between the actual fc and predicted fc by (a) GBR and (b) KNN models..
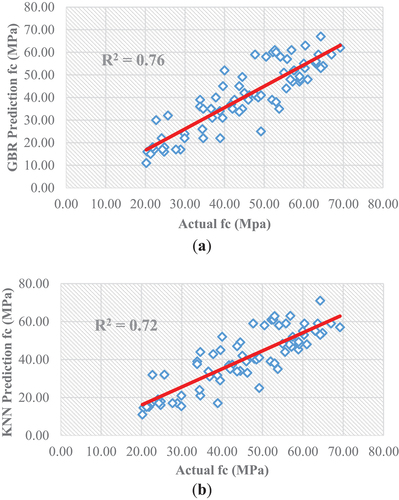
Data availability statement
The data used to support the findings of this study are included in this published article.