Figures & data
Table 1. Recommendations from delegates: priority actions to support adaptive pathways for DRI.
Figure 4. (a) Realization of the system response; (b) comparison of expected NPV between traditional and adaptation pathway simulation designs.
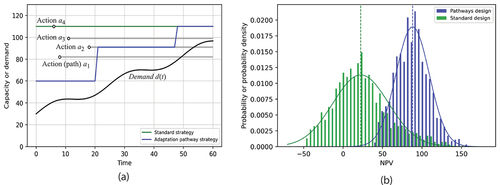
Figure 5. (a) Realizations of the system response to an extreme event; (b) comparison of expected system recovery time.
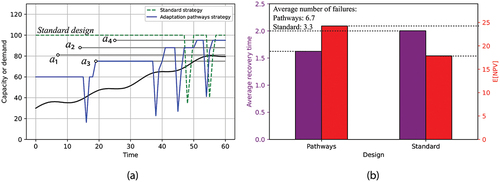
Table 1. Summary of relevant data sources and details. More information is provided in Hickford et al. (Citation2023).
Table 2. Description of adaptation options considered with costs, relevant asset types and impacts on damage curves and return period cut-offs.
Figure 1. Graphical representation of transport system-of-systems risk and adaptation assessment framework.
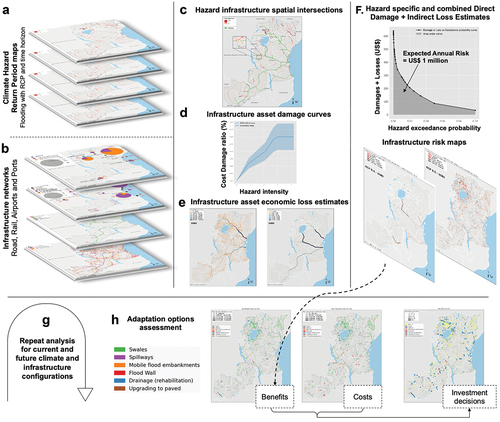
Figure 2. Results of maximum (a) PV of benefits PVs; (b) PV of adaptation investments; and (c) BCR for adaptation options for roads at risk due to river and coastal flooding under climate change.
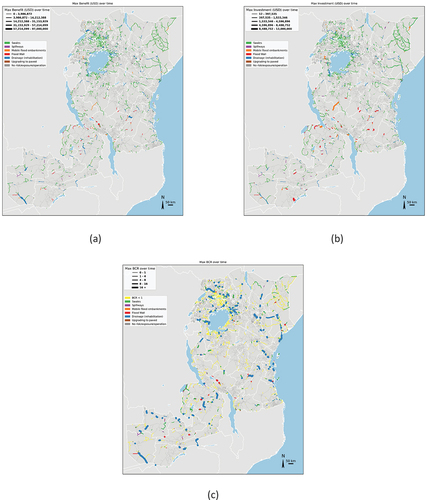
Figure 3. Results of maximum (a) PV of benefits; (b) PV of adaptation investments; and (c) BCR for adaptation options for railways.
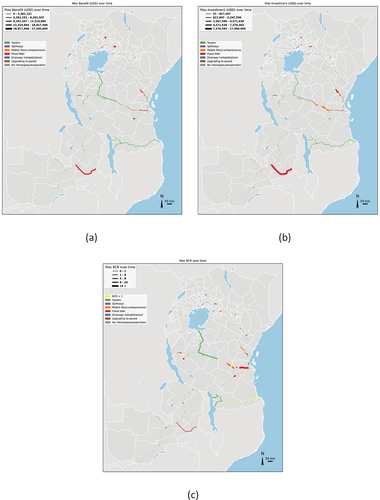
Figure 2. Downscaling principle to adapt climatic models’ outputs to the land-use (Adapted from Willems [2011] in Siwila et al. (Citation2013).
![Figure 2. Downscaling principle to adapt climatic models’ outputs to the land-use (Adapted from Willems [2011] in Siwila et al. (Citation2013).](/cms/asset/605ae8de-64ba-438f-a33e-c220fc801904/tsri_a_2181552_f0011_oc.jpg)
Figure 3. Conceptual mapping of vulnerability (Sharma & Ravindranath, Citation2019).
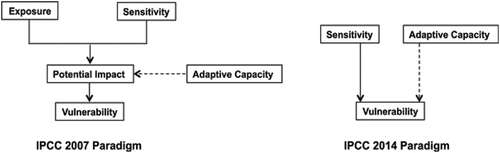
Figure 5. The resilience performance assessment analytical table allowing the evaluation of climate change impacts.
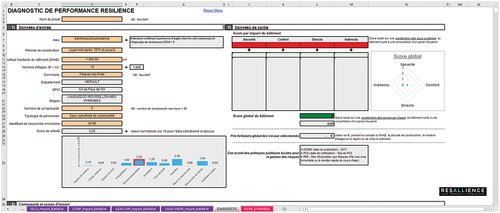
Figure 6. Exposure mapping of multiple hazards to identify most exposed parts of the island to complex risks such as hurricanes.

Figure 7. Mapping of vulnerability from multiple hazards to identify the most vulnerable sectors of the island.
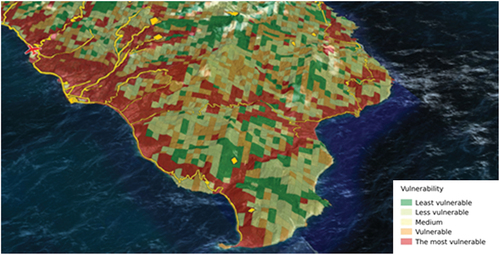
Figure 8. Localization of current and future investment projects facing island territorial vulnerability.
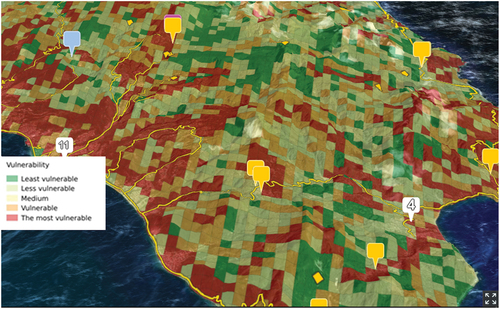
Table 1. Valuation example comparing NPV and DNPV estimations (US$).
Figure 1. Hourly storm hyetograph from 26 July 2005 at 2.30pm to 27 July 2005 at 2.30am with a period of high intensity between 2.30pm and 9.30pm (IST).
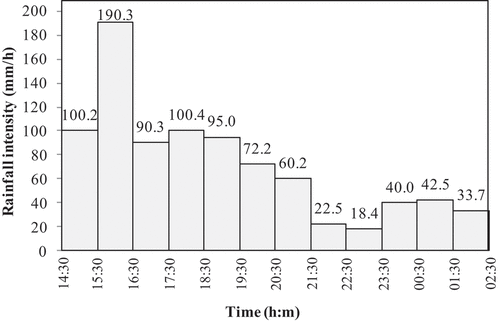
Figure 1. Conceptualization of the research methodology, including the data sources (Landsat and Sentinel imagery), processing steps within GEE and the outputs to be used by stakeholders.
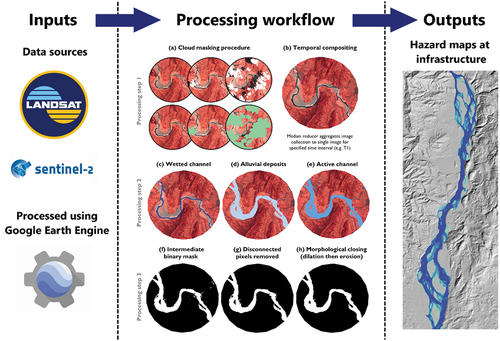
Table 1. Characteristics of the satellite imagery data used in InfraRivChange.
Figure 6. InfraRivChange web application applied outside of the Philippines, to the Chahlari Ghat Bridge (India).
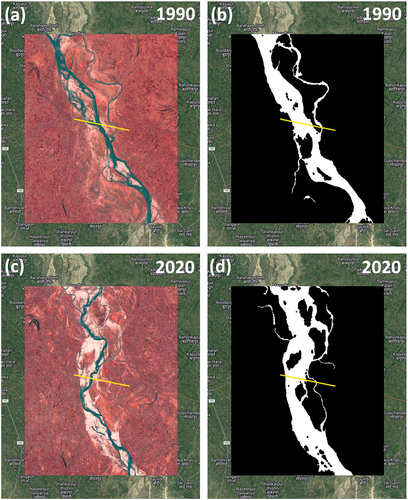
Table 1. Terrain derivatives and definitions used in this study. Modified after (Safanelli et al., Citation2020).
Table 2. Rainfall derivatives and definitions used in this study (Talchabhadel et al., Citation2022).
Figure 2. Annual landslide susceptibility map for years 2016 to 2020 produced using a random forest model in Google Earth Engine.
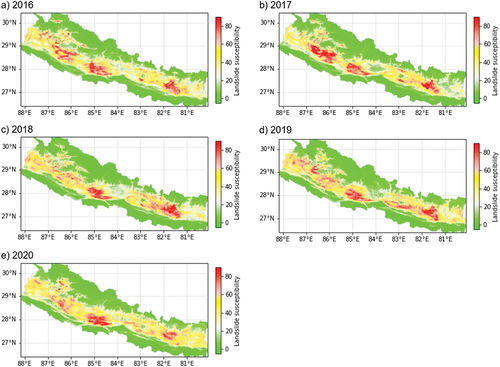
Table 3. Validation score of landslide susceptibility maps: (i) area under the curve value (higher is better); and (ii) out of bag error in random forest model training (lower is better).
Figure 3. (a) LISI map classes; (b) CISI map classes; and (c) LISI overlaid on CISI classes (LISI > 5 and CISI > 5).
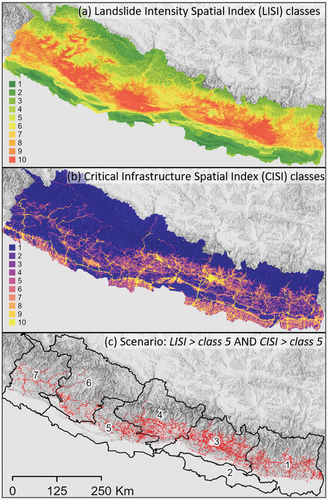
Figure 4. Snapshot of a web application for dissemination of LISI, CISI and other relevant datasets. The snapshot shows LISI of Nepal.
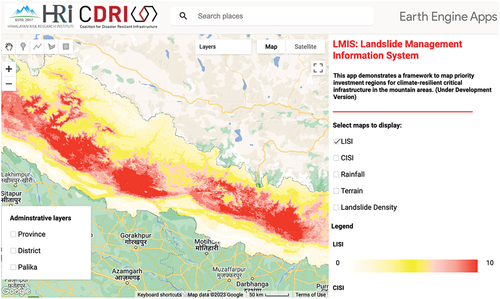
Table 1. Result of Delphi survey phase II: consensus indicators.
Table 2. Result of Delphi survey phase II: non-consensus indicators.
Table 3. Result of Delphi survey phase III.
Table 1. Result of Delphi survey phase II: consensus indicators.
Table 2. Result of Delphi survey phase II: non-consensus indicators.
Table 3. Result of Delphi survey phase III.
Figure 5. Extreme negative solar impacts in relation to high air temperature scenarios in Montreal across different months based on 1953–2005 data.
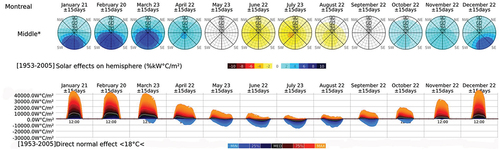
Figure 6. Distribution, probabilities and passive solar impacts in Montreal across different months using 1953–2005 data.
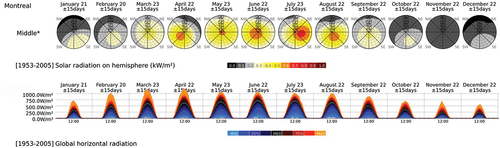
Figure 7. Distributions, probabilities and active solar potentials in Montreal across different months using 1953–2005 data.
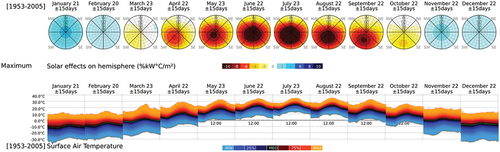
Figure 1. Space weather disturbances from the Sun (adopted from Eastwood et al., Citation2017).
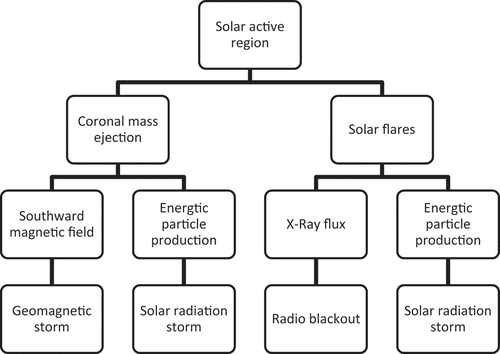
Table 1. Classifications of sunspots or active regions.
Table 2. Potential influence of solar flares.
Figure 4. Variation in TEC values registered on 12 May 2015 (X axis = Hour of the day; Y axis = TEC value).
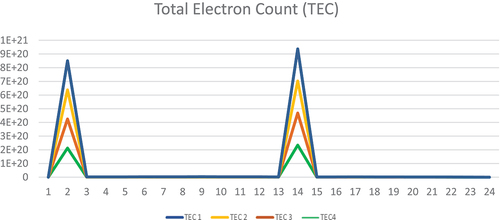
Figure 3. Water stability responses of various specimens of unstabilized and stabilized soil/alternate soils.
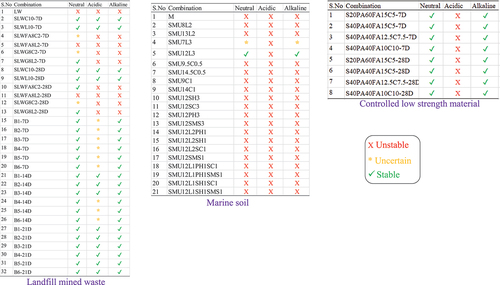
Figure 4. Typical crumb test images for marine soil, landfill mined waste and controlled low strength material specimens.
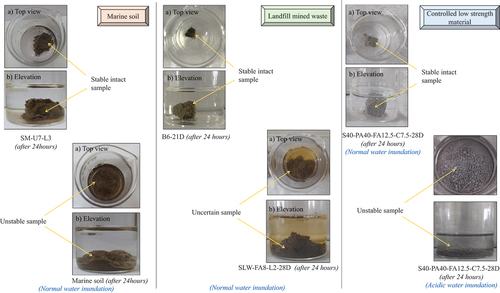
Figure 2. THM for representing the force-deformation behaviour of UFREIs (Banerjee & Matsagar, Citationn.d.).
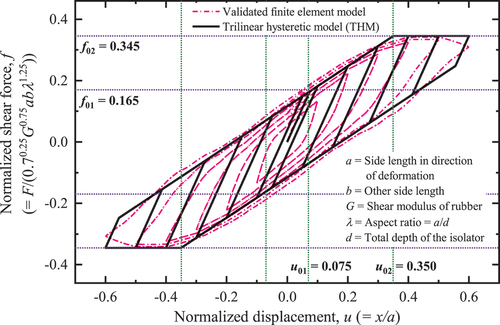
Table 1. Details of site-specific earthquake excitations considered in this study.
Figure 5. Time history of top-floor absolute acceleration responses (truncated) of hospital building with and without base isolation.
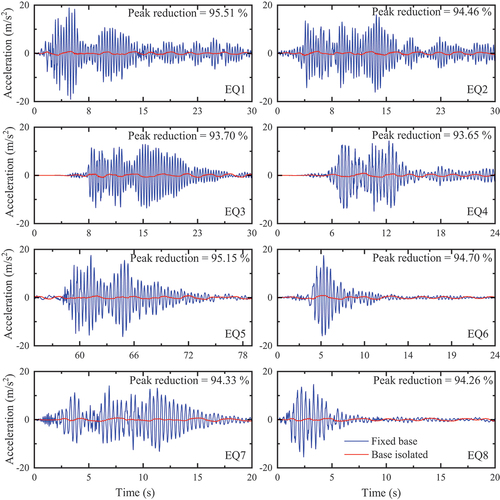
Table 1. Scenario losses, repair time and recovery time assessment results.
Figure 1. Probability of (a) occupancy loss and (b) functionality loss at DBE and MCE over time for various pathways.
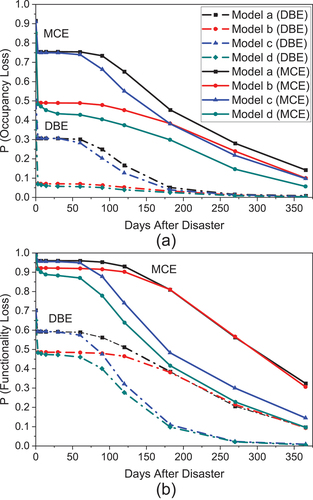
Table 1. Datasets and their description.
Table 2. Description of parameters used for built-up area prediction.
Table 1. Datasets and their description.
Table 2. Description of parameters used for built-up area prediction.
Figure 3. Damage stage analysis of predicted built-up area (2050) in 100-yr flood: return period for (a) 24 hrs and (b) 48 hrs.
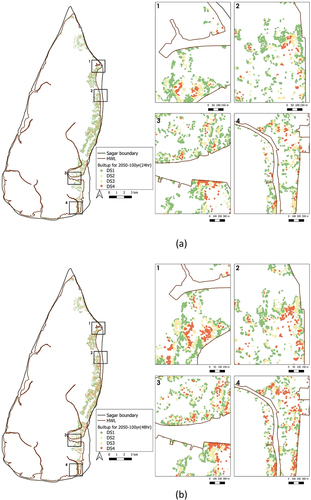
Figure 4. Building damage stage analysis against 100-year flood-return period (a) pre-mitigation, (b) post-mitigation using NBS.
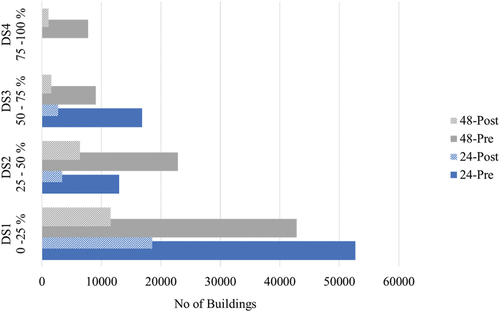
Table 3. Description of parameters used for estimating cost-benefit of NBS.
Table 1. Risk assessment indicators and variables.
Table 2. Multi-hazard index.
Table 3. Risk index of case study districts.
Figure 1. Water Square/Plaza in Benthemplein.
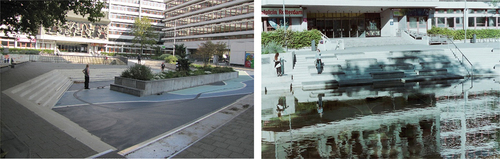
Figure 2. Sønder Boulevard.
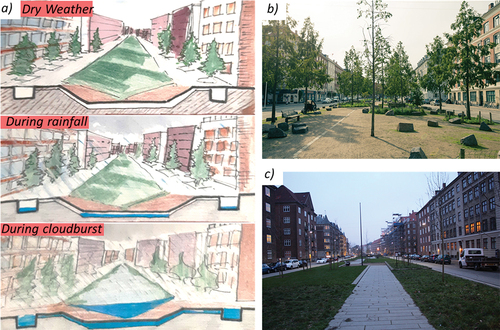
Figure 1. Location and elevation maps of the transboundary Himalayan mountain region in Asia includes the world’s 10 highest peaks.
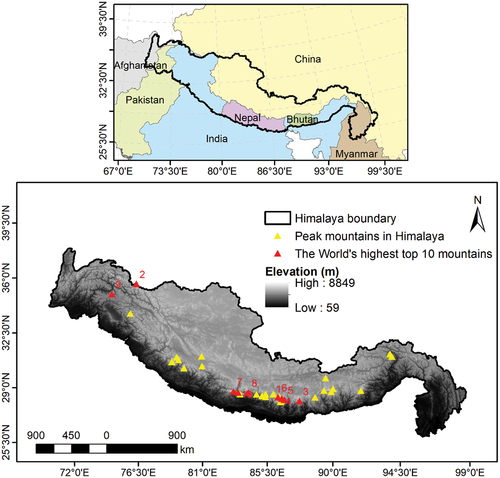
Table 1. List of data and their source websites.
Figure 2. Distribution of structural losses corresponding to a 100-year return period for a cyclonic wind hazard.
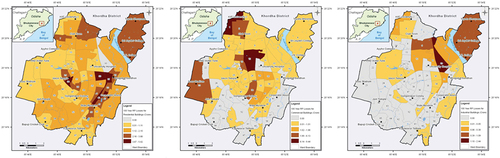
Figure 3. Snippets of the mobile application to be used in the post-disaster scenario for rescue, relief and recovery.
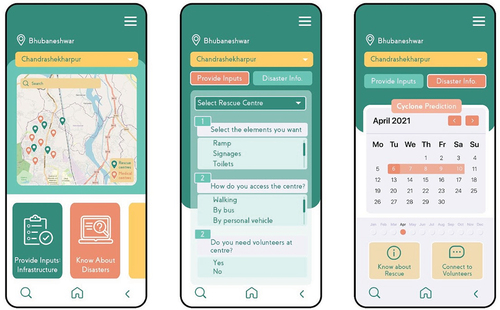
Figure 4. Snippets of the mobile application to be used in the post-disaster scenario for rescue, relief and recovery.
