Figures & data
Table 1. Datasets for classification, detection, and segmentation of the vehicle damage.
Figure 4. Samples of annotated images in the VehiDE dataset. The first row presents the images of damaged vehicle; the second row shows the annotated labels; the last row demonstrates the masks of vehicles.
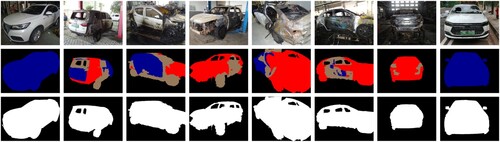
Table 2. SOD method hyper-parameters. denotes the interval between the ith and jth epochs.
Table 3. Results of CSNet, -Net, PoolNet, and SGL-KRN's quantitative salient object detection.
Table 4. Results of SGL-KRN's quantitative salient object detection for each class.