Figures & data
Figure 1. XRD pattern of ErB2 (top) and LuB2 (bottom) samples. Asterisks in the figure denote Er2O3 or Lu2O3 impurity. Dots correspond to the experimental data, while lines correspond to the calculated pattern by Rietveld analysis. Inset: Crystal structure of REB2, drawn by VESTA software [Citation16].
![Figure 1. XRD pattern of ErB2 (top) and LuB2 (bottom) samples. Asterisks in the figure denote Er2O3 or Lu2O3 impurity. Dots correspond to the experimental data, while lines correspond to the calculated pattern by Rietveld analysis. Inset: Crystal structure of REB2, drawn by VESTA software [Citation16].](/cms/asset/0945d2d4-969b-45b0-a80a-bf04ec6418a7/tstm_a_2217474_f0001_oc.jpg)
Figure 2. (a) Temperature dependence of magnetic susceptibility in ErB2 (top) and its temperature-derivative (bottom), taken at = 0.01 T. Inset shows isothermal magnetization curve of ErB2, taken at
= 5 K. (b) Inverse magnetic susceptibility of ErB2 at 1 T. The dashed line in the figure is the fit to Curie–Weiss law in the temperature range of 200–300 K.
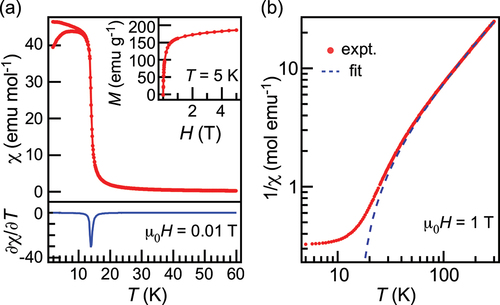
Figure 3. (a) Specific heat of ErB2 under various magnetic fields. (b) Entropy curves of ErB2 under magnetic fields deduced from data in (a). (c) of ErB2, obtained from data in (b). Gray open squares denote
estimated from magnetization curves (supplemental information S1). (d)
of ErB2, obtained from data in (b).
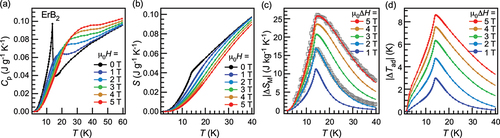
Figure 4. (a) Specific heat of ErB2 and LuB2 (left axis, solid lines) and estimated (right axis, dashed line). (b) Magnetic entropy curve of ErB2 as a function of temperature.
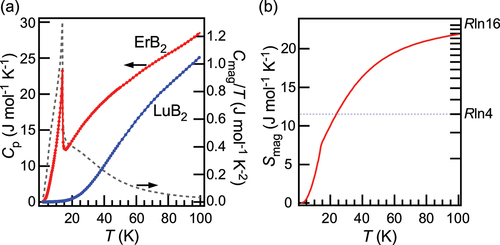
Figure 5. (a) Comparison of values in REB2 (RE = Tb, Dy, Ho, Er, Tm) at
= 5 T between experiment (left axis, black filled circles [Citation4,Citation10–12], and this work) and prediction by the machine-learned model (right axis, red open squares). (b)
values predicted by machine-learned model plotted against the experimental values for REB2 (red open squares) and those in the test dataset for the model (gray circles) [Citation4].
![Figure 5. (a) Comparison of |ΔSM|peak values in REB2 (RE = Tb, Dy, Ho, Er, Tm) at μ0ΔH = 5 T between experiment (left axis, black filled circles [Citation4,Citation10–12], and this work) and prediction by the machine-learned model (right axis, red open squares). (b) |ΔSM|peak values predicted by machine-learned model plotted against the experimental values for REB2 (red open squares) and those in the test dataset for the model (gray circles) [Citation4].](/cms/asset/356fd91a-9eee-4170-a2e9-ec5e31b0bcb9/tstm_a_2217474_f0005_oc.jpg)
Figure 6. Summary plots of SHAP values up to the top 15 compositional descriptors, sorted by (a) magnitude of the absolute mean (i.e. significance), and (b) magnitude of RE-dependence in REB2 prediction. Dots in the figure represents each data point of the training dataset.
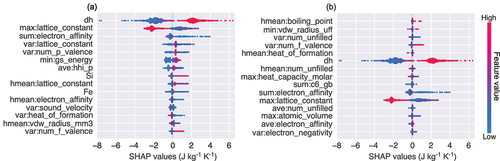
Figure 7. Top panel: Target values in training data (gray circles) plotted against focused compositional descriptors of (a) harmonic mean of boiling point, (b) minimum of van der Waals radius, and (c) maximum of molar heat capacity. Horizontal dashed lines correspond to the mean target value of training data (7.48). Predicted values for REB2 are also shown as colored open squares. Middle panel: The same for SHAP values for corresponding compositional descriptors. Bottom panel: RE-dependence of SHAP values for corresponding compositional descriptors in the prediction for REB2.
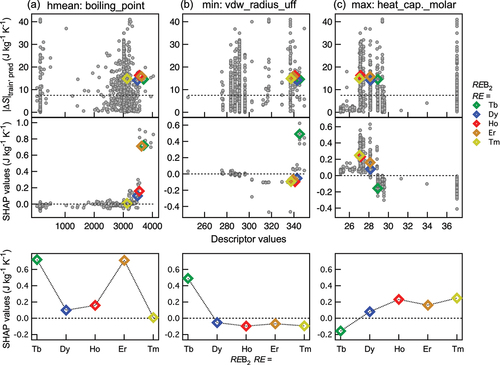
Figure 8. RE-dependence of SHAP values. (a) Total sum of SHAP values that is identical to predicted target values. (b) Partial sum of SHAP values. (c) Sum of SHAP values for the rest of descriptors.
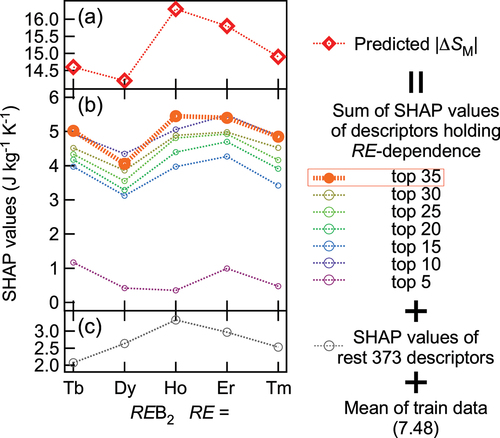
Figure 9. Schematic workflow of materials search using compositional descriptor-based machine-learning model (blue arrows) and proposed possible materials design assisted by SHAP analysis of the model. (a)(b) Focused physical property for atomic elements (X). (c) Target plotted against compositional descriptor
. (d) Constructed model by regression of data in (c). (e) SHAP value plotted against compositional descriptor
. (f) Sorted physical property for atomic elements (X).
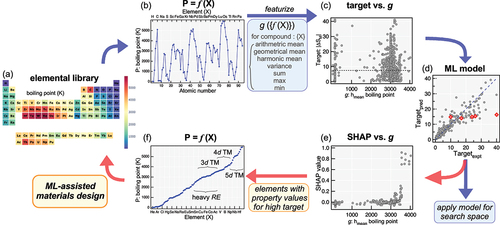
Figure 10. Left panel: Training data distribution of target plotted against focused compositional descriptors. Middle panel: The same as the left panel for SHAP values. Right panel: Heatmap of properties of atomic elements stored in XenonPy [Citation6]. Focused compositional descriptors are the ones that exhibited the top five largest absolute mean SHAP values in the model (shown in Figure 6(a)), namely (a) maximum of lattice constant, (b) sum of electron affinity, (c) variance of lattice constant, (d) variance of the number of valence -electrons, and (e) minimum of ground state energy in first principles software VASP [Citation19]. Datapoints for several representative magnetocaloric materials are indicated explicitly, Gd5Si1.5Ge2.5 [Citation20], EuTiO3 [Citation21], EuS [Citation22], ErCo2 [Citation23], La0.8Ce0.2Fe11.7Si1.3 [Citation24] and abbreviated as GSG, ETO, ES, EC, LFS, respectively.
![Figure 10. Left panel: Training data distribution of target plotted against focused compositional descriptors. Middle panel: The same as the left panel for SHAP values. Right panel: Heatmap of properties of atomic elements stored in XenonPy [Citation6]. Focused compositional descriptors are the ones that exhibited the top five largest absolute mean SHAP values in the model (shown in Figure 6(a)), namely (a) maximum of lattice constant, (b) sum of electron affinity, (c) variance of lattice constant, (d) variance of the number of valence p-electrons, and (e) minimum of ground state energy in first principles software VASP [Citation19]. Datapoints for several representative magnetocaloric materials are indicated explicitly, Gd5Si1.5Ge2.5 [Citation20], EuTiO3 [Citation21], EuS [Citation22], ErCo2 [Citation23], La0.8Ce0.2Fe11.7Si1.3 [Citation24] and abbreviated as GSG, ETO, ES, EC, LFS, respectively.](/cms/asset/95906ecf-f833-4f05-853e-92bf86ed3134/tstm_a_2217474_f0010_oc.jpg)