Figures & data
Figure 1 Information of the IMU: (A) 3-axis of IMU and (B) the position of the IMU.
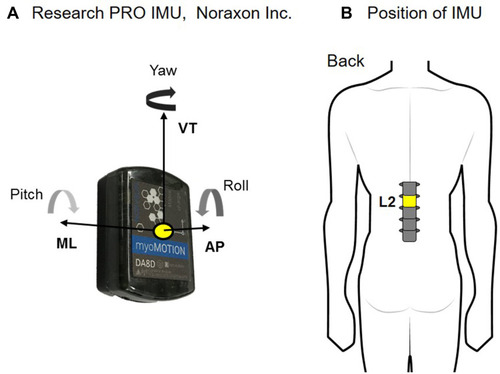
Figure 2 Experimental set-up for collecting physical data of the elderly.
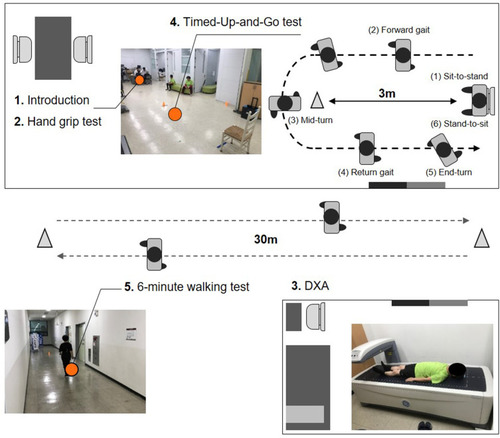
Table 1 Sarcopenia Stage Grouping, According to AWGS Criterion for Female
Figure 3 The framework of identifying the optimal classification model for predicting sarcopenia-stage.
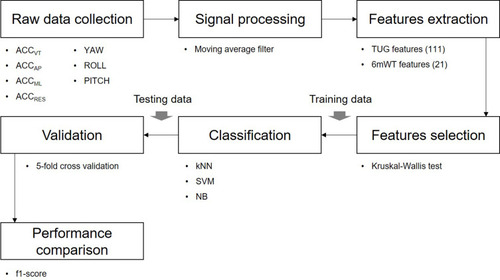
Table 2 Features of TUG
Figure 4 The example of identification the TUG sub-tasks using angular velocity.
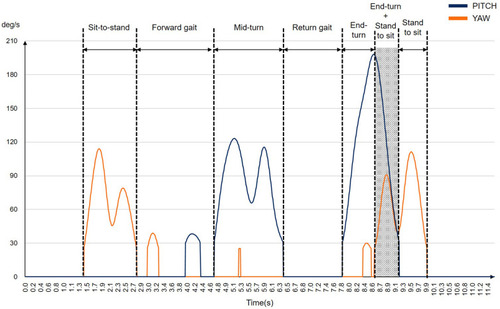
Table 3 Features of 6mWT
Table 4 Feature Selection Datasets
Figure 5 Classification models performance depending on feature selection datasets: (A) Results of FALL, (B) Results of FTUG, (C) Results of F6mWT, and (D) Final results.
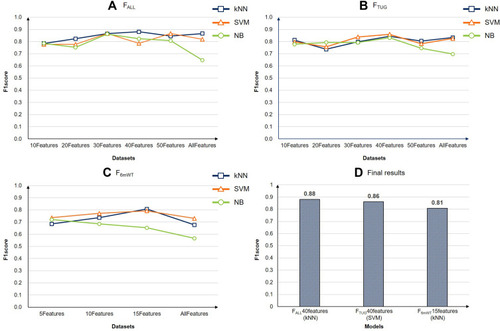