Figures & data
Figure 1 The data analysis and machine learning schema.
Abbreviations: ER, estrogen receptor; SVM, support vector machine; ROC, receiver operating characteristic; PCA, principal component analysis.
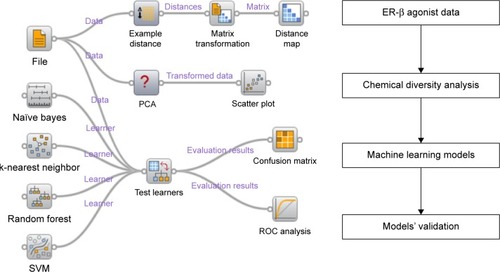
Figure 2 Principal component analysis (PCA) of the dataset.
Abbreviations: Ext, extended; AP2D, 2D atom pairs; FP, fingerprints.
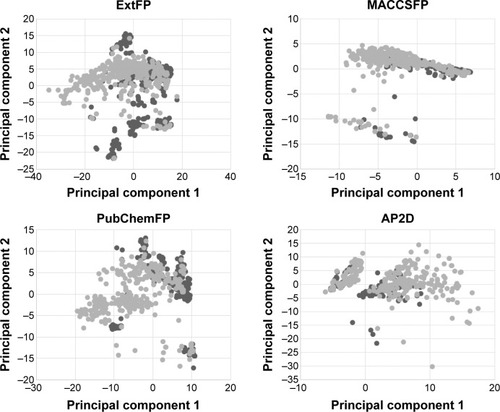
Figure 3 The heat map of distance matrix for the compounds in the collected dataset.
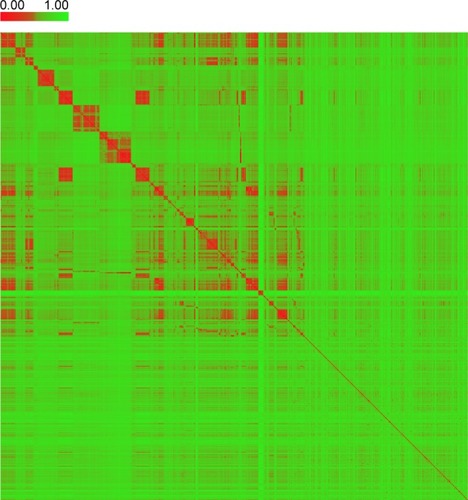
Table 1 Model performances of 5-fold cross validation
Figure 4 The ROC curves of the 5-fold cross validation models based on four types of fingerprints (FP) and four machine learning approaches.
Abbreviations: ROC, receiver operating characteristic; NB, Naïve Bayesian; KNN, k-nearest neighbor; RF, random forest; SVM, support vector machine; Ext, extended; AP2D, 2D atom pairs; TP, true positives, FPos, false positives.
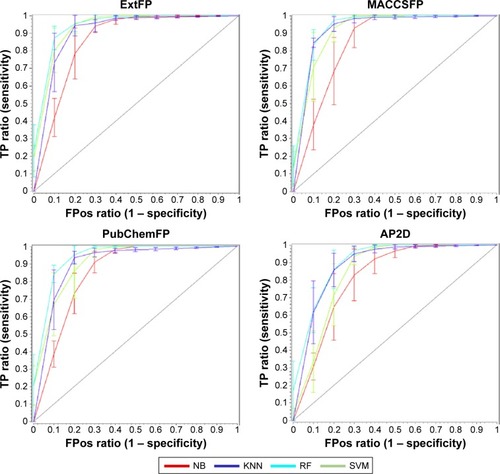
Figure 5 Performance ranking of machine learning methods with various fingerprints (FP).
Abbreviations: NB, Naïve Bayesian; KNN, k-nearest neighbor; RF, random forest; SVM, support vector machine; Ext, extended; AP2D, 2D atom pairs.
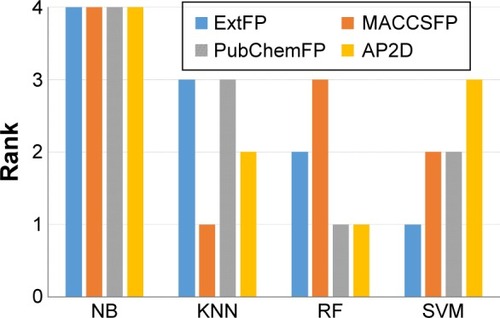
Figure 6 Performance ranking of fingerprints (FP) in various machine learning methods.
Abbreviations: NB, Naïve Bayesian; KNN, k-nearest neighbor; RF, random forest; SVM, support vector machine; Ext, extended; AP2D, 2D atom pairs.
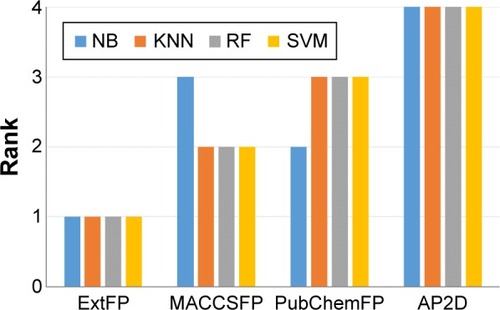
Table 2 Model performances of test set
Table 3 Model performances of external test set
Table S1 Ten-fold cross validation model performance
Table S2 Five-fold cross validation model performance using experimental inactive agonists