Figures & data
Figure 1 Analysis of single-cell sequencing data. (A) Reduced dimensional cluster analysis, t-distributed stochastic neighbor embedding (t-SNE) distribution of different cell clusters. (B) A violin diagram showing the marker genes for each cell type. (C) Distribution of t-SNE in different cell types. The red arrow indicates the reference gene of epithelial cells.
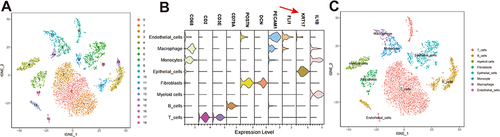
Figure 2 Analysis of epithelial cell signaling pathways. (A–C) Network diagram depicting the interaction of the Wnt, transformation growth factor (TGF), and epidermal growth factor (EGF) signaling pathways. (D–F) Heatmap of the interaction of the Wnt, TGF, and EGF signaling pathways. (G–I) AUCell analysis based on the Reactome pathway database.
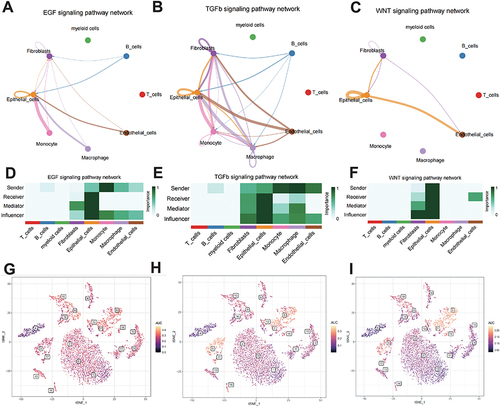
Figure 3 Acquisition of differentially expressed genes. (A) Principal component analysis plot of the GSE161533 and GSE20347 dataset concatenation. (B) Volcano map of merged differentially expressed genes. (C) Uniform Manifold Approximation and Projection plot showing differentially expressed genes of different cell types. (D) Wayne diagram of differentially expressed genes and epithelial cells in ESCC.
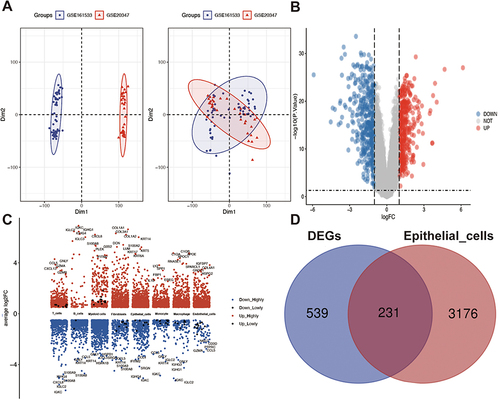
Figure 4 Immuno-infiltration analysis. (A) ESTIMAT analysis of the high- and low-risk group. (B) CIBERSORTx analysis of the abundance of immune cells. (C) Heatmap of correlation between risk score and the presence of immune cell types. (D) The single-sample Gene Set Enrichment Analysis scores of 28 immune cells in the high- and low-risk groups. *p < 0.05, **p < 0.01, ***p < 0.001, ****p < 0.0001.
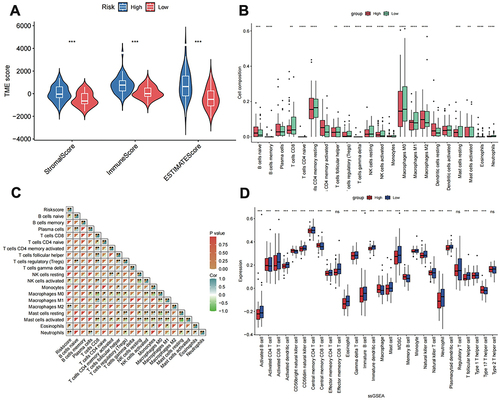
Table 1 Detailed Information on LAPTM4B, CTSL, MYO10, NCF2, and PDLIM2
Figure 5 Screening and validation of core prognostic genes. (A) Prognostic forest map. (B) LASSO analysis to construct a fitted model. (C) Determining the best lambda value for the fitted model: the x-axis represents the logarithmized lambda value, the y-axis represents the error of the model, and the dotted line on the left represents the lambda value that minimizes the error and the number of features screened. (D–H) Dataset GSE45670 validation. (I–M) Validation of the GEPIA dataset. *p < 0.05.
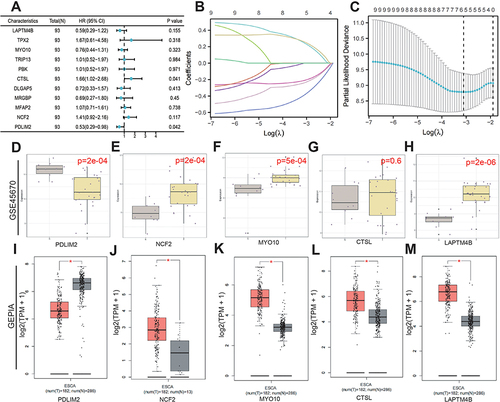
Figure 6 Nomogram construction of related genes. (A) Nomogram construction. (B) ROC curves and calibration curves for the nomograms in the training group. (C) ROC curves and calibration curves for the nomograms in the validation group.
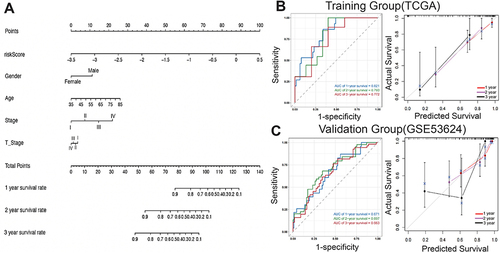
Figure 7 Epithelial cell analysis. (A) The t-distributed stochastic neighbor embedding (t-SNE) of 10 cell clusters. (B) Dot plot of different marker genes. (C) Identification of tumor and paratumor marker genes; (D) Copy number variation profile showing relative differences between the tumor and paratumor groups. (E) Violin plot of essential genes.
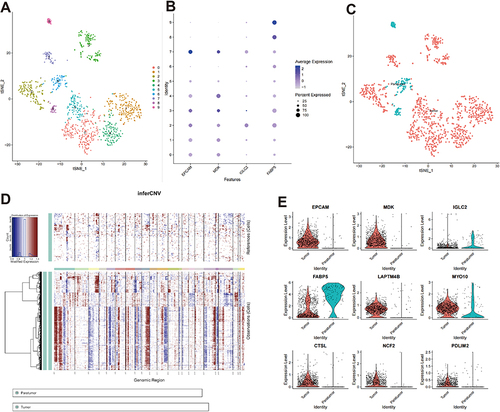
Table 2 Detailed Information on the Top 8 Sensitive Drugs
Figure 8 Drug sensitivity analysis. (A–H) Top 8 drugs showing the most sensitivity with Risk score. High/Low indicates the grouping of samples into high and low categories. p represents the result of statistical analysis, with p<0.05 indicating significance.
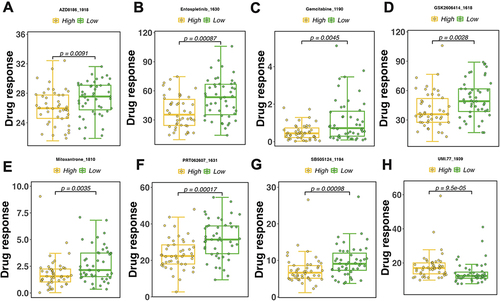
Figure 9 Molecular docking of β-sitosterol with five core genes and the mRNA levels were measured by real-time fluorescence quantitative PCR. (A-E) Molecular docking of β-sitosterol to five core genes. (F) The docking results were presented in a heatmap. (G) K-M survival analysis of the five core genes in the TCGA database. (H) Further validation of the core genes in the external dataset GSE53624.
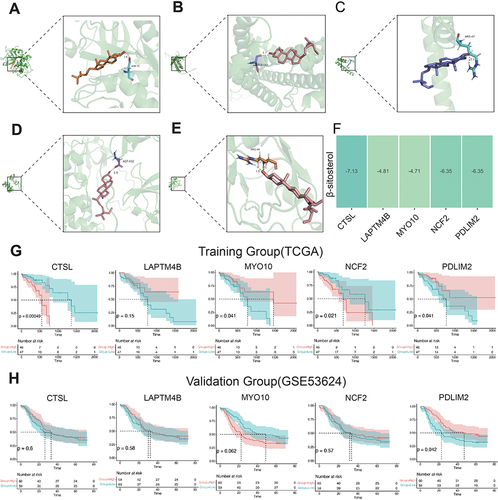
Figure 10 Overexpression of PDLIM2 significantly inhibits the proliferation and migration of KYSE150 and Eca9706 cells. (A) q-PCR detection of PDLIM2 expression in esophageal squamous cell carcinoma cell lines and normal esophageal epithelial cell lines. (B and C) q-PCR and WB were used to detect the overexpression efficiency of PDLIM2 in KYSE150 and Eca9706 cells. (D) The effect of PDLIM2 on the migration of KYSE150 and Eca9706 cells was explored in wound healing assays. (E) Compared with the control group, overexpressed PDLIM2 significantly inhibits the migration and invasion of esophageal squamous carcinoma cells. (F) The effect of PDLIM2 on the proliferation of KYSE150 and Eca9706 cells was investigated in colony formation assays. **p < 0.01, ***p < 0.001. Scale bars: 100 µm.

Data Sharing Statement
Data will be made available on request. For further information, please contact the corresponding author.