Figures & data
Figure 1. Possible cells of origin of glioma. Studies in mouse models have shown that various cell types can give rise to glioma. Neural stem cells (NSCs) give rise to other Neural Stem Cells, Astrocytes, Astrocyte-like cells, and neurons. Liu et al. 2011 demonstrated that NSCs give rise to OPCs, which can give rise to glioma. Friedmann-Morvinski et al. 2012 demonstrated that astrocytes and neurons can give rise to glioma. Hambardzumyan et al. 2011 demonstrated that astrocytes can give rise to glioma after PDGF overexpression and Ink4a, and ARF deletion. Koso et al. 2012 demonstrated that overexpression of a mutagenic Sleeping Beauty (SB) transposon (T2/Onc2) along with a dominant negative p53 in astrocyte like cells can give rise to glioma. Chen et al., 2012 demonstrated that NSCs could give rise to glioma after Nf1, p53, and Pten deletion. Neural Stem Cells can give to proneural, mesenchymal, and neural cell lineages.
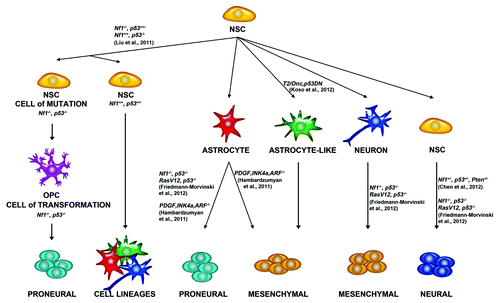
Figure 2. Competing endogenous RNA levels modulate expression of oncogenes and tumor suppressors. (A) The levels of oncogenes can be modulated by an increase in levels in competing endogenous RNAs. microRNAs are titrated away from mRNAs encoding oncogenes when the levels of competing endogenous RNAs are increased. This leads to increased expression of oncogenes. (B) The levels of tumor suppressor proteins are modulated by decreases in the levels of competing endogenous RNAs. microRNAs bind to RNAs of tumor suppressors, thereby reducing tumor suppressor protein expression after transformation.
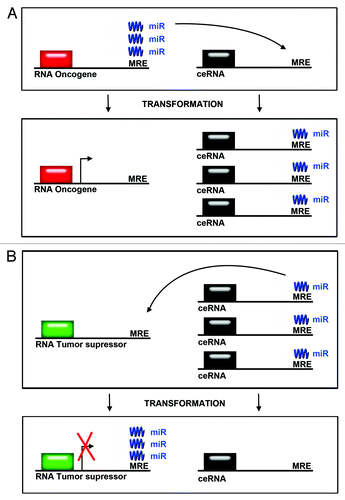
Figure 3. A bioinformatics and statistical pipeline for identifying epigenetic targets for GBM from transcriptome data. Hypothetical pipeline for identifying epigenetic targets in GBM based on differentially expressed pathways in both differentiating neural stem cell and GBM. Left Panel: Differentiating neural stem cells are analyzed for changes in RNA transcript levels by performing RNA-sequencing analysis of differentiating cells. RNA sequencing yields transcripts expressed over time. Mapping/alignment of transcripts using human genome is performed using Tophat and quantification of aligned transcripts is then performed using Cufflinks, or similar bioinformatics pipeline. Statistical filtering by t-tests or analysis of variance after quantification yields differentially expressed genes. Clustering of genes by patterns is then performed to identifying RNAs that are associated with differentiation pathways. Right Panel: RNA-sequencing of GBM and control tissue is performed to identify differentially expressed genes using the same bioinformatics pipeline utilized in analyzing differentiating neural stem cells. The degree of overlap of those transcripts, which are differentially expressed during differentiation, and in GBM is then calculated to identify epigenetic targets in GBM.
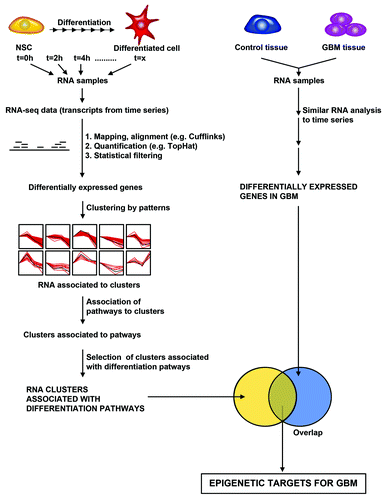
Figure 4. Examples of statistical models for neural temporal data. (A) Time series. Each transcript is modeled separately (univariate) or as part of a group (multivariate). The model uses information from previous time points in modeling future time points, and can capture contemporaneous and lagged dependencies among transcripts. (B) Discrete Markov chain model. Each cellular stage is considered a “state” and the chain models the probabilities of moving from one “state” to another in a given time step. Depending on the type of Markov model it may or may not be possible to move both backward and forward in time, and hence for cells to differentiate as well as dedifferentiate. (C) Bayesian network model. If we consider a directed acyclic graph (DAG), then we define a joint probability distribution over cellular states. For each node or state we define a probability distribution for transcription in each state, conditional on transcription in previous states. If we consider a dynamic graphical model (DGM), then we can model each state with a graphical model, and separately model the movement from state to state across time. In this way transcripts can have contemporaneous as well as time-dependent relationships. NSC, neural stem cell; OPC1, oligodendrocyte precursor cell 1; OPC2, oligodendrocyte precursor cell 2; Olig, oligodendrocyte; GBM, glioblastoma cell. Pa1 is the probability that a neural stem cell remains a stem cell from one time point to the next. Pa2 is the probability that a neural stem cell transforms from the current time point to the next time point. Pb3 is the probability that a GBM cell de-differentiates from the current time point to the prior time point. Pb1 is the probability that a GBM cell remains a GBM cell from the current time point to the next. Pc2 is the probability that an oligodendrocyte precursor cell (OPC) transforms from the current time point to the next. Pc1 is the probability that an OPC remains an OPC from the current time point to the next. Pb2 is the probability that a GBM cell de-differentiates into an OPC from the current time point to the next time point. Pc4 is the probability that an OPC differentiates into an oligodendrocyte from the current time point to the next time point. Pc3 is the probability that an OPC dedifferentiates into a neural stem cell from the current time point to the next. Pa3 is the probability that a NSC differentiates into an OPC from the current time point to the next. Pd1 is the probability that an oligodendrocyte will remain an oligodendrocyte from the current time point to the next. Pd2 is the probability that an oligodendrocyte dedifferentiates into an OPC form the current time point to the next. Pa1+Pa2+Pa3 = 1; Pb1+Pb2+Pb3 = 1; Pc1+Pc2+Pc3 = 1.
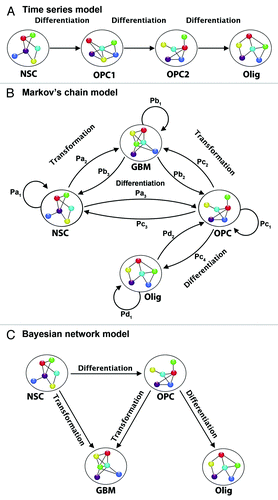