Figures & data
Figure 1. Comparison of automated TSS annotation from dRNA-seq data with TTSpredator and TSSAR. The upper plot pair shows the mapped read coverage in the treated (L+) and untreated (L-) library for an exemplary region from H. pylori dRNA-seq data.Citation53 Blue dashed lines indicate TSS annotated by TTS predator (using default parameter). The middle plot pair shows essentially the same data, but only the read start coverage is plotted. This is how TSSAR looks at the data. Dashed red lines indicate TSS annotated by TSSAR (P value cutoff of 10−4). The bottom part shows the positions of the annotated genes in the considered region. The read coverage plots indicate that the data produced by dRNA-seq is more complex than it might appear from the method description; therefore, statistical data analysis is required.
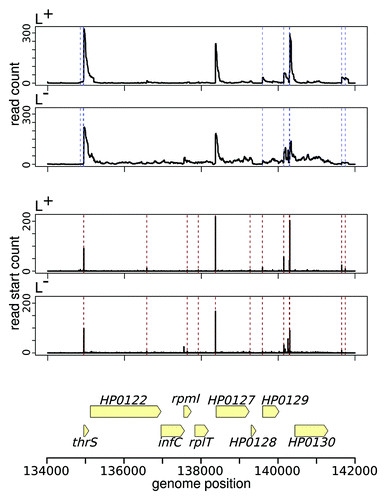
Figure 2. Evolutionary signals are used to classify multiple sequence alignments into non- or protein-coding. RNAz combines structural and thermodynamic descriptors and measures of sequence conservation to detect excess conservation of secondary structure, while RNAcode identifies increased conservation of putative ORFs compared with the observed sequence conservation of the nucleic acid sequences. Well-conserved structured RNAs, such as Xanthomonas sX13, which is involved in virulence-specific gene expression and hfq mRNA regulation, can easily be identifiedCitation71 with RNAz. The E. coli transcript C0343, originally annotated as a small RNA, does not exhibit typical features of a structured RNA. Instead, RNAcode reveals a well-conserved short coding sequence.Citation72 Dual transcripts such as B. subtilis sR1Citation73 are detectable by both RNAz and RNAcode.
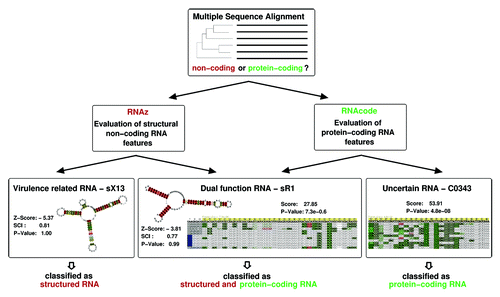
Figure 3. Features describing a secondary structure graph. Each graph is described by the set of all neighborhood subgraphs (indicated by shaded areas) up to a maximal radius r around a reference nucleotide (marked by a circle).
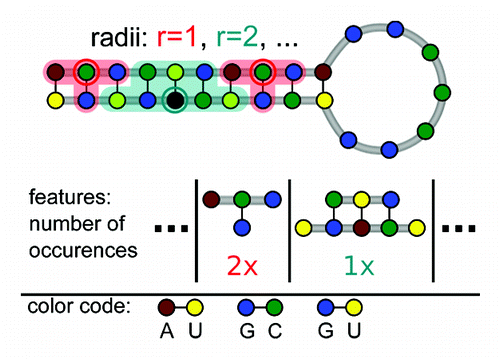
Table 1. Web server for genome-scale prediction of sRNA target genes
Figure 4. Comparative prediction of sRNA targets as implemented in the CopraRNA pipeline. For a given pair of sRNA and mRNA sequences, the associated homologs are selected. In the next step, the best interaction in each species is determined and scored by its P value. Finally, all species-specific P values are combined into a single joint P value while taking the evolutionary distances into account.
