Figures & data
Figure 1. Computational pipeline for detection of molecular mimicry candidates in human pathogenic bacteria.
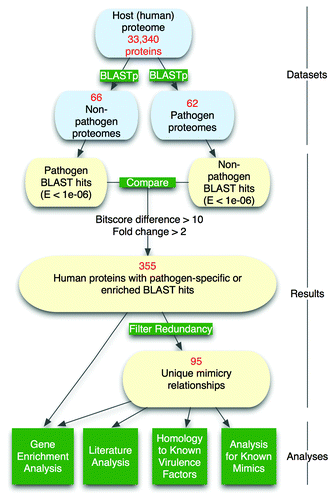
Table 1. Top 25 unique predictions of molecular mimicry relationships.
Figure 2. Top BLAST matches for human proteins in pathogen vs. non-pathogen proteomes. Left: −log10E-values for top BLAST matches to human proteins in 62 human pathogens vs. 66 non-pathogens. Right: −log10E-values for top BLAST matches to human proteins with different host/pathogen definitions (6 plant pathogens vs. 16 non-pathogens). Values above ~60 are not shown. Collagens (top detected mimicry relationship) and ADP-ribosylating factors (positive control mimicry relationship) have pathogen-elevated E-value distributions.
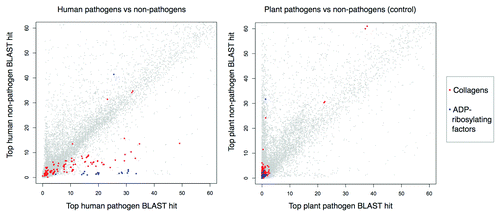
Figure 3. Top pathogen vs. non-pathogen protein similarities to a selected set of predicted human mimicry targets. Predicted human mimicry targets were selected from the top 25 detected relationships (), and the top BLAST matches by bitscore (x-axis) in pathogen vs. non-pathogen proteomes (frequency on y-axis) have been plotted. In each case, it can be seen that a subset of pathogen proteomes encode putative mimics that exhibit much greater similarities to human proteins than similarities found in non-pathogen proteins. A selected portion of the alignment is shown for the top-scoring pathogen mimic in each case. See Data File S1 for additional details regarding pairwise alignments.
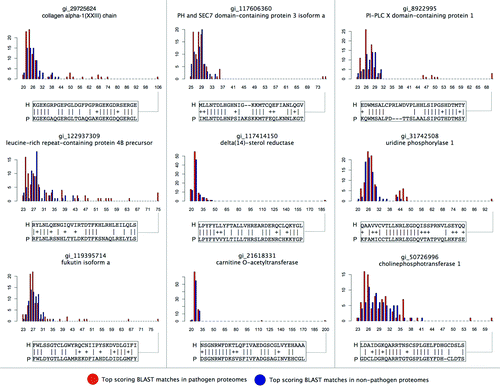
Table 2. Function enrichment analysis of predicted mimicry candidates
Table 3. Predicted mimicry candidates in human pathogenic bacteria and potential roles in virulence
Figure 4. Independent evolution of ECM mimics from separate repeat amplifications. High-scoring collagen-like (A) and leucine-rich repeat (B) protein mimics were selected and divided into their constituent protein repeats, which were aligned and used to generate sequence logos. Differences between the sequence logos of each repetitive protein suggest evolution from separate progenitor peptides and repeat amplifications. (C) An example demonstrating similarity of leucine-rich repeat sequence conservation patterns between a human NOD-like receptor (NLRC3) and a predicted mimicry candidate (lpl1579) from Legionella pneumophila. The detected level of sequence similarity between these two proteins is far above that observed in non-pathogens (blue) and other pathogens (red) as indicated by the BLAST bitscore distribution (left panel).

Figure 5. Phylogenetic trees of bacterial pathogen encoded collagen-like repeats (left) and leucine-rich repeats (right) from . The repeats are colored in the tree according to their parent protein. Top-aligning repeats from human proteins have also been included and are colored light green. Repeats cluster predominantly by protein of origin, suggesting that different pathogen repeat proteins have evolved by independent repeat amplifications. Interestingly, the pathogen repeat classes generally cluster with a specific human repeat, suggesting that ancestral progenitor repeats may be host-derived.
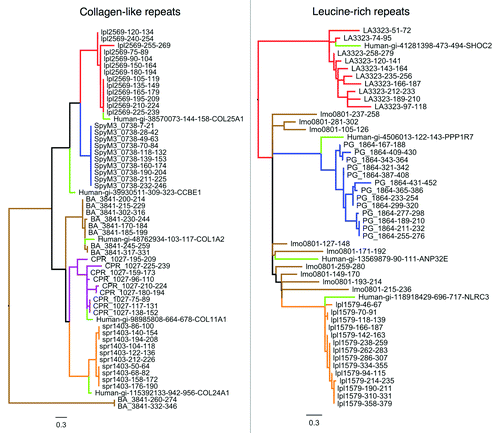