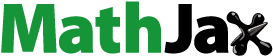
Abstract
A central objective of empirical research on treatment response is to inform treatment choice. Unfortunately, researchers commonly use concepts of statistical inference whose foundations are distant from the problem of treatment choice. It has been particularly common to use hypothesis tests to compare treatments. Wald’s development of statistical decision theory provides a coherent frequentist framework for use of sample data on treatment response to make treatment decisions. A body of recent research applies statistical decision theory to characterize uniformly satisfactory treatment choices, in the sense of maximum loss relative to optimal decisions (also known as maximum regret). This article describes the basic ideas and findings, which provide an appealing practical alternative to use of hypothesis tests. For simplicity, the article focuses on medical treatment with evidence from classical randomized clinical trials. The ideas apply generally, encompassing use of observational data and treatment choice in nonmedical contexts.
1 Introduction
A central objective of empirical research on treatment response is to inform treatment choice. Identification problems combine with the necessity of inference from sample data to limit the informativeness of studies. Unfortunately, researchers commonly use concepts of statistical inference whose foundations are distant from the problem of treatment choice. It has been particularly common to use hypothesis tests to compare treatments.
The Wald (Citation1950) development of statistical decision theory provides a coherent frequentist framework for use of sample data on treatment response to make treatment decisions. A body of recent research applies statistical decision theory to characterize uniformly satisfactory treatment choices, in the sense of maximum loss relative to optimal decisions (also known as maximum regret). This article describes the basic ideas and findings, which provide an appealing practical alternative to use of hypothesis tests.
To keep the exposition simple and framed in a familiar setting, I focus on medical treatment with evidence from classical randomized clinical trials. Trials have long enjoyed a favored status within evidence-based medicine, often being called the “gold standard” for collection of data on treatment response. The influential Cochrane system for grading the quality of evidence ordinarily reserves its highest rating for evidence from randomized trials (Higgins and Green 2011, Sec. 12.2.1). The drug approval process of the U.S. Food and Drug Administration (FDA) ordinarily considers only experimental evidence when making decisions on drug approval. While I focus on the use of trial data in medical decision making, the broad ideas discussed here are general, encompassing use of observational data and treatment choice in nonmedical contexts.
Section 2 reviews the use of hypothesis tests to compare medical treatments and the basic principles of statistical decision theory. Section 3 describes the recent research on uniformly satisfactory treatment choice using trial data. Section 4 concludes.
Readers may be aware that it has become increasingly common to express concern that evaluation of empirical research by the outcome of hypothesis tests generates publication bias and diminishes the reproducibility of findings. See, for example, Ioannidis (Citation2005) and Wasserstein and Lazar (Citation2016). This concern is important, but it is distinct from the theme of the present article.
The article relates directly to other important issues covered in the ASA Statement on Statistical Significance and p-Values (Wasserstein and Lazar Citation2016). Two of the six Principles of the Statement are
“3. Scientific conclusions and business or policy decisions should not be based only on whether a p-value passes a specific threshold.”
“5. A p-value, or statistical significance, does not measure the size of an effect or the importance of a result.”
Treatment choice using statistical decision theory is not based at all on whether a p-value passes a threshold. Statistical decision theory clearly distinguishes between the statistical and clinical significance of empirical estimates of treatment effects.
2 Background
2.1 Using Hypothesis Tests to Compare Treatments
A long-standing practice in medicine has been to use trial data to test a specified null hypothesis against an alternative and to use the outcome of the test to compare treatments. A common procedure when comparing two treatments in a trial is to view one as the status quo and the other as an innovation. The usual null hypothesis is that the innovation is no better than the status quo and the alternative is that the innovation is better. If the null hypothesis is not rejected, it is recommended that the status quo treatment continue to be used in clinical practice. If the null is rejected, it is recommended that the innovation become the treatment of choice. This type of test is institutionalized in the FDA drug approval process, which calls for comparison of a new drug with a placebo or a previously approved treatment. Approval of the new drug normally requires rejection of the null hypothesis of zero average treatment effect in two independent trials (Fisher and Moyé Citation1999).
The convention has been to perform a test that fixes the probability of rejecting the null hypothesis when it is correct, the probability of a Type I error. Then sample size determines the probability of rejecting the alternative hypothesis when it is correct, the probability of a Type II error. The power of a test is defined as one minus the probability of a Type II error. The convention has been to choose a sample size that yields specified power at some value of the effect size deemed clinically important. For example, International Conference on Harmonisation (1999) has provided guidance for the design and conduct of trials evaluating pharmaceuticals, stating (p. 1923)
Conventionally the probability of type I error is set at 5% or less or as dictated by any adjustments made necessary for multiplicity considerations; the precise choice may be influenced by the prior plausibility of the hypothesis under test and the desired impact of the results. The probability of type II error is conventionally set at 10% to 20%.
Manski and Tetenov (Citation2016) observed that there are several reasons why hypothesis testing may yield unsatisfactory results for medical decisions and other forms of treatment choice. These include
Use of Conventional Asymmetric Error Probabilities: It has been standard to fix the probability of Type I error at 5% and the probability of Type II error at 10–20%. The theory of hypothesis testing gives no rationale for selection of these conventional error probabilities. It gives no reason why a clinician concerned with patient welfare should find it reasonable to make treatment choices that have a substantially greater probability of Type II than Type I error.
Inattention to Magnitudes of Losses to Welfare When Errors Occur: A clinician should care about more than the probabilities of Type I and II error. He should care as well about the magnitudes of the losses to patient welfare that arise when errors occur. A given error probability should be less acceptable when the welfare difference between treatments is larger, but the theory of hypothesis testing does not take this into account.
Limitation to Settings with Two Treatments: A clinician often chooses among several treatments and many clinical trials compare more than two treatments. Yet the standard theory of hypothesis testing only contemplates choice between two treatments. Statisticians have struggled to extend it to deal sensibly with comparisons of multiple treatments.
The third issue is well-appreciated, but the first two are often overlooked. A simple example shows why they may matter for patient care.
2.1.1 Example
Suppose that a typically terminal form of cancer may be treated by a status quo treatment or an innovation. It is known from experience that mean patient life span with the status quo treatment is 1 year. Prior to use of the innovation, medical researchers see two possibilities for its effectiveness. It may be less effective than the status quo, yielding a mean life span of only 1/3 of a year, or it may be much more effective, yielding a mean life span of 5 years.
Suppose that a classical randomized trial is performed to learn the effectiveness of the innovation. Let the trial data be used to perform a conventional hypothesis test comparing the innovation and the status quo. The null hypothesis is that the innovation is no more effective than the status quo and the alternative is that the innovation is more effective. The probability of a Type I error is set at 0.05 and that of a Type II error is 0.20. The test result is used to choose between the treatments.
A Type I error occurs with frequentist probability 0.05 and reduces mean patient life span by 2/3 of a year (1 year minus 1/3 year). A Type II error occurs with frequentist probability 0.20 and reduces mean patient life span by 4 years (5 years minus 1 year). Thus, use of the test to choose between the status quo and the innovation implies that society is willing to tolerate a large (0.20) chance of a large welfare loss (4 years) when making a Type II error, but only a small (0.05) chance of a small welfare loss (2/3 of a year) when making a Type I error. The theory of hypothesis testing does not motivate this asymmetry.
2.2 Principles of Statistical Decision Theory
2.2.1 Basic Ideas
The standard formalization of decision making under uncertainty supposes that a decision maker must choose among a set of feasible actions. The welfare achieved by an action depends on an unknown feature of the environment, called the state of nature. The decision maker wants to choose an action that maximizes welfare, but he cannot do this with certainty because the state of nature is unknown. The decision maker lists all states of nature that he believes could possibly occur. This list, the state space, expresses partial knowledge. The larger the state space, the less the decision maker knows about the outcomes of actions.
For example, the decision maker may be a clinician and the actions may be treatments for a patient. A state of nature may characterize how a patient would respond to alternative treatments, which may be incompletely known. Welfare may be a health outcome of interest, perhaps patient life span or quality of life, that would occur when a specified treatment is administered to a patient. The clinician might ideally want to choose a treatment that optimizes the patient’s health outcome but, having incomplete knowledge of treatment response, he cannot do this with certainty.
Suppose that a sampling process generates observable sample data that are informative about the true state; for example, data on how each member of a sample of patients has responded to the assigned treatment. Wald (Citation1950) considered the general problem of using such sample data to make decisions. He posed the task as choice of a statistical decision function, which maps potentially available data into a choice among the feasible actions. Wald’s seminal book is abstract, making it a difficult read. Ferguson (Citation1967), Berger (Citation1985), and Parmigiani and Inoue (Citation2009) provide comprehensive expositions.
Wald recommended ex ante evaluation of statistical decision functions as procedures applied as the sampling process is engaged repeatedly to draw independent data samples. The idea of a procedure transforms the original statistical problem of induction from a single sample into the deductive problem of assessing the probabilistic performance of a statistical decision function across realizations of the sampling process. Thus, the theory is frequentist.
Wald proposed that the decision maker evaluate a statistical decision function by the mean welfare it yields across realizations of the sampling process. His presentation differed semantically from the one that I use to describe treatment choice in that he defined loss to be the negative of welfare, took the objective to be minimization of loss rather than maximization of welfare, and used the term risk to denote mean loss across realizations of the sampling process. With these semantic distinctions, he prescribed a three-step decision process
Specify the set of feasible actions, the loss (or welfare) function, and the state space. These basic concepts of decision theory are context specific. The set of feasible actions is commonly considered to be predetermined. The loss function and the state space are subjective. The former formalizes what the decision maker wants to achieve and the latter expresses what states of nature he believes could possibly occur.
Eliminate inadmissible statistical decision functions. A decision function is inadmissible if there exists another that yields at least as good mean sampling performance in every state of nature and strictly better mean performance in some state.
Use some criterion to choose an admissible statistical decision function. Wald focused on the minimax criterion and on minimization of a subjective mean of the risk function (called Bayes risk). Savage Savage (Citation1951) proposed minimax regret.
2.2.2 Decision Criteria
What are reasonable ways to choose an admissible decision function? The Bayesian approach is particularly well known. It has been common to think of the Bayesian process of transforming a prior into a posterior distribution as antithetical to frequentist statistics, but Wald provided a clear frequentist perspective on Bayes decisions. He showed that minimization of Bayes risk, a frequentist decision criterion, yields the same decisions as would occur if one performs Bayesian inference, combining the prior distribution with the data to form a posterior subjective distribution, and then chooses an action to minimize the posterior mean of expected loss. Berger (Citation1985, Sec. 4.4.1) gives an accessible proof.
Bayesian decision making is compelling when one feels able to place a credible subjective prior distribution on the state space. There exists a considerable body of work ranging across multiple disciplines that develops methods to help persons conceptualize uncertainty and express themselves in subjective probabilistic terms. See, for example, Savage (Citation1971), Koriat, Lichtenstein, and Fischhoff (Citation1980), Morgan and Henrion (Citation1990), Manski (Citation2004b), and Garthwaite, Kadane, and O’Hagan (Citation2005).
Nevertheless, Bayesians have long struggled to provide guidance on specification of priors to be used in evidence-based medicine and the matter continues to be controversial. See, for example, the spectrum of views regarding Bayesian analysis of randomized trials expressed by the authors and discussants of Spiegelhalter, Freedman, and Parmar (Citation1994). The controversy suggests that inability to express a credible prior is common in actual decision settings.
When one finds it difficult to assert a credible subjective distribution, Bayesian statisticians who believe it essential to use a probability distribution to express uncertainty may suggest use of some default distribution that is variously called a “reference” or “conventional” or “objective” prior; see, for example, Berger (Citation2006). However, there is no consensus on what prior should play this role. The choice made matters for decision making.
An alternative is to abandon the notion that one must use a probability distribution to express uncertainty. In the absence of a prior, Wald argued that a reasonable way to act is to use a decision criterion that achieves uniformly satisfactory results, whatever the true state of nature may be. There are multiple ways to formalize the idea of uniformly satisfactory results. The two most commonly studied are embodied in the minimax and minimax-regret (MR) criteria.
The minimax criterion chooses an action that minimizes the maximum risk that might possibly occur, across all feasible states of nature. The minimax-regret criterion considers each state of nature and computes the incremental risk that occurs if one chooses a specified action rather than the one that minimizes risk in this state. This quantity, called regret, measures the nearness to optimality of the specified action in the state of nature. The decision maker must choose without knowing the true state. To achieve a uniformly satisfactory result, he computes the maximum regret of each action; that is, the maximum distance from optimality that the action would yield across all possible states of nature. The MR criterion chooses an action that minimizes maximum regret.
The minimax and MR criteria are sometimes confused with one another, but they yield the same choice only in certain special cases. Although the minimax criterion considers only the worst outcome that an action may yield, MR considers the worst outcome relative to what is achievable in a given state of nature. (Savage Citation1951) distinguished the minimax criterion sharply from MR, writing that the former criterion is “ultrapessimistic” while the latter is not. Maximum regret quantifies how uncertainty—lack of knowledge of the true state of nature—potentially diminishes the quality of decisions.
It is important to understand that use of the minimax or the MR criteria does not eliminate all subjectivity in decision making. As discussed earlier, decision theory begins with specification of a welfare function and a state space, both of which are subjective. Bayes decision theory goes a step further by placing a subjective distribution on the state space. The minimax and MR criteria do not embrace this further element of subjectivity, but they still require the decision maker to specify a welfare function and state space.
The reader may have noticed that, to introduce this discussion, I asked what are “reasonable” decision criteria rather than what is an “optimal” criterion. Statistical decision theorists recognized from the outset that there is no singularly optimal way to choose an admissible decision function. There at most are reasonable ways. Wald (Citation1950), who was particularly concerned with decision making in the absence of a prior distribution on the state space, motivated his focus on the minimax criterion in part by stating (p. 18)a minimax solution seems, in general, to be a reasonable solution of the decision problem when an a priori distribution …does not exist or is unknown to the experimenter.
Ferguson (Citation1967) wrote (p. 28)
It is a natural reaction to search for a ‘best’ decision rule, a rule that has the smallest risk no matter what the true state of nature. Unfortunately, situations in which a best decision rule exists are rare and uninteresting. For each fixed state of nature there may be a best action for the statistician to take. However, this best action will differ, in general, for different states of nature, so that no one action can be presumed best overall.
He went on to write (p. 29): “A reasonable rule is one that is better than just guessing.”
2.2.3 Some History, Post Wald
The Wald framework has breathtaking generality. In principle, it enables comparison of all statistical decision functions whose risk functions exist. It enables comparison of alternative sampling processes as well as decision rules. It uses no asymptotic approximations. It applies whatever information the decision maker may have. The state space may be finite dimensional or larger; that is, nonparametric. The true state of nature may be point or partially identified. Settings with partial identification are ones where the sampling process generating the data incompletely reveals the true state asymptotically; see Manski (Citation2003), (2007a).
Given the appeal of statistical decision theory, one might anticipate that it would play a central role in modern statistics. However, this has not occurred. After publication of Wald (Citation1950), a surge of important extensions and applications followed in the 1950s. Much research focused on best point prediction under square loss with sample data, analysis of which began with Hodges and Lehmann (Citation1950). In this important case, regret is mean square error and the MR criterion yields a predictor that minimizes maximum mean square error across the state space.
However, this period of rapid development closed by the 1960s, with the exception of Bayesian statistical decision theory. Bayesian analysis has continued to develop, but as a self-contained field of study disconnected from the Wald frequentist framework. Recent research in Bayesian statistics has focused more on the computational problem of transformation of priors into posteriors than on use of posteriors in decision making.
Why did statistical decision theory lose momentum long ago? One reason may have been the technical difficulty of the subject. Wald’s ideas are easy to describe abstractly, but applying them can be analytically and computationally demanding. Determination of admissible decision functions and minimax/minimax-regret rules is often difficult. Another reason may have been diminishing interest in decision making as the motivation for analysis of sample data. Modern statisticians tend to view their objectives as estimation and hypothesis testing rather than decision making.
I cannot be sure what role these or other reasons played in the vanishing of statistical decision theory from statistics in the latter part of the twentieth century. However, the near absence of the subject in mainstream journals and textbooks of the period is indisputable. I think this is unfortunate. The recent research described in the next sections aims to reinvigorate statistical decision theory, focusing on the important applied problem of treatment choice. Other recent research, not described here, provides new analysis of best point prediction under square loss when the sampling process is afflicted with missing data or other problems of imperfect data quality; see Dominitz and Manski (Citation2017).
3 Recent Work on Statistical Decision Theory for Treatment Choice
Bayesian statistical decision theory has long been available to design trials and to choose treatments with trial data. DeGroot (Citation1970) provides a classical treatise on the subject. Canner (Citation1970), Spiegelhalter, Freedman, and Parmar (Citation1994), Cheng, Su, and Berry (Citation2003), and Spiegelhalter (2004) study various aspects. However, as mentioned above, Bayesians have struggled to provide guidance on specification of priors and the matter continues to be controversial. Perhaps as a result, Bayesian analysis is well known but seldom used in evidence-based medicine. A limited exception is that the FDA has provided guidance permitting the use of Bayesian statistics in the design and analysis of clinical trials evaluating new medical devices; see U. S. Food and Drug Administration ((2010)).
I describe here a recent body of research that avoids specification of priors and instead studies uniformly satisfactory treatment choice, using maximum regret to measure performance across states of nature. Contributions to this emerging literature include Manski (Citation2004a, Citation2005, Citation2007a,Citationb), Schlag (Citation2006), Hirano and Porter (Citation2009), Stoye (Citation2009, Citation2012), Tetenov (Citation2012), Manski and Tetenov (Citation2016), and Kitagawa and Tetenov (Citation2018).
The recent research supposes that the objective of treatment choice is to maximize a social welfare function that sums treatment outcomes across a population of patients that may have heterogeneous treatment response. For example, the objective may be to maximize the five-year survival rate in a population of cancer patients or mean life span in a population with a chronic disease. In this setting, a statistical decision function uses the data to choose an allocation of patients to treatments. Using terminology introduced in Manski (Citation2004a), such a function has been called a statistical treatment rule (STR).
The mean sampling performance of an STR across repeated samples is its expected welfare. This term means the negative of Wald’s risk and is used because the objective is to maximize welfare rather than minimize loss. Given that the objective is to maximize rather than minimize a function, the minimax decision criterion becomes maximin instead. The MR criterion remains as earlier except that regret in a state of nature now is the maximum welfare achievable in that state minus the expected welfare of a specified STR.
In what follows, Section 3.1 explains why the recent work has measured the performance of STRs by maximum regret. Section 3.2. discusses treatment choice with existing trial data. Section 3.3 considers the design of trials.
3.1 Measuring Performance by Maximum Regret
In the absence of a prior distribution on the state space, practical and conceptual reasons motivate measurement of the performance of STRs by maximum regret across the state space, rather than by minimum expected welfare. I explain here.
3.1.1 Practical Appeal
From a practical perspective, it has been found that MR decisions behave more reasonably than do maximin ones in the context of treatment choice. In common settings of treatment choice with trial data on outcomes that take a bounded range of values, it has been found that the MR rule is well approximated by the empirical success (ES) rule, which chooses the treatment with the highest observed average outcome in the trial. The ES rule provides a simple and plausible way to use the results of a trial. The performance of the ES rule from the perspective of maximum regret was initiated by Manski (Citation2004a). Subsequently, Schlag (Citation2006) and Stoye (2009) showed that this rule either exactly or approximately minimizes maximum regret in common settings with two treatments when sample size is moderate. Hirano and Porter (Citation2009) showed that the ES rule is asymptotically optimal.
In contrast, the maximin rule commonly ignores the trial data, whatever they may be. When Savage (Citation1951) stated that the minimax criterion is “ultrapessimistic,” he went on to write (p. 63): “it can lead to the absurd conclusion in some cases that no amount of relevant experimentation should deter the actor from behaving as though he were in complete ignorance.” Savage did not flesh out this statement but it is easy to show that this occurs with trial data. Manski (Citation2004a) provides a simple example that will be discussed in Section 3.2.
3.1.2 Conceptual Appeal
The conceptual appeal of using maximum regret to measure performance is that maximum regret quantifies how lack of knowledge of the true state of nature diminishes the quality of decisions. While the term “maximum regret” has become standard in the literature, it is important to keep in mind that this term is a shorthand for the maximum suboptimality of a decision criterion across the feasible states of nature. An STR with small maximum regret is uniformly near-optimal across all states.
Maximum regret is well-defined in general settings with multiple treatments and when patients have heterogeneous observable covariates that may be used to differentiate treatment. However, the concept is especially transparent when there are two treatments and the members of the patient population are observationally identical, all having the same observable covariates.
Suppose there are two feasible treatments, say A and B. In a state of nature where A is better, the regret of an STR is the product of the probability across repeated samples that the rule commits a Type I error (choosing B) and the magnitude of the loss in expected welfare that occurs when choosing B. Similarly, in a state where B is better, regret is the probability of a Type II error (choosing A) times the magnitude of the loss in expected welfare when choosing A.
Recall the critique in Section 2.1 of the conventional use of hypothesis testing to choose a treatment. I called attention to the asymmetric treatment of Type I and Type II error probabilities and the inattention to magnitudes of losses when errors occur. Evaluating treatment rules by regret overcomes both problems. Regret considers Type I and II error probabilities symmetrically and it measures the magnitudes of the losses that errors produce.
3.1.3 Example
To illustrate, consider again the example of Section 2.1.1, in which a conventional hypothesis test is used as an STR to choose between a status quo treatment for cancer and an innovation. There are two feasible states of nature in the example, with the innovation yielding mean life span of 1/3 year in one state and 5 years in the other. In the first state, the regret of this conventional “test rule” equals 1/30 of a year; that is, a 0.05 chance of a Type I error times a 2/3 of a year reduction in mean life span with improper choice of the innovation. In the second state, the regret of the test rule equals 4/5 of a year; that is, a 0.20 chance of a Type II error times a 4-year reduction in mean life span with improper choice of the status quo. Thus, the maximum regret of the test rule is 4/5 of a year.
Rather than use the conventional test rule to choose between the status quo and the innovation, one could seek an STR that has smaller maximum regret. Given the available trial data, a simple option would be to reverse the conventional probabilities of Type I and Type II; thus, one might use a test with a 0.20 chance of a Type I error and a 0.05 chance of a Type II error. In the first state, the regret of this unconventional test rule STR equals 2/15 of a year; that is, a 0.20 chance of a Type I error times a 2/3 of a year reduction in mean life span with improper choice of the innovation. In the second state, the regret of the unconventional test rule equals 1/5 of a year; that is, a 0.05 chance of a Type II error times a 4-year reduction in mean life span with improper choice of the status quo. Thus, the maximum regret of the unconventional test rule is 1/5 of a year.
In this example, the unconventional test rule delivers much smaller maximum regret than does the conventional test rule. There may exist other STRs that perform even better.
3.2 Treatment Choice With Existing Trial Data
To move beyond verbal discussion and examples, I now formalize treatment choice as a statistical decision problem in the relatively simple setting of a classical trial with two treatments and a population of observationally identical patients. The presentation in this section draws substantially on Manski (2007, Chap. 12). The research literature cited earlier also studies more general and complex settings with multiple treatments, patients who have heterogeneous observable covariates, and imperfect trials that only partially identify treatment response.
3.2.1 General Analysis
Suppose that a health planner must assign treatment A or B to each member of patient population J. Each patient has response function yj(⋅):
mapping treatments
into individual outcomes
. Let P denote the distribution of treatment response in the population.
The members of the population may respond heterogeneously to treatment, but they are observationally identical to the planner. For any , the planner can allocate a fraction δ of patients to treatment B and
to A. The planner wants to choose δ to maximize an additive welfare function
(1)
(1) where
] and
] are the mean outcomes if everyone were to receive treatment A or B, respectively. The quantity
is the average treatment effect (ATE) in the population. It is optimal to set δ = 1 if the ATE is positive and δ = 0 if the ATE is negative. The problem of interest is treatment choice when incomplete knowledge of P makes it impossible to determine the sign of the ATE.
Suppose that sample data are available. Let Q be the sampling distribution and be the sample space. For example, the data may be treatment response observed in a randomized trial. A STR is a function
that maps sample data into a treatment allocation. The welfare realized with δ and data ψ is the random variable
(2)
(2)
The state space is the set of (P,
pairs that the planner deems possible. Expected welfare in state s, the mean sampling performance of rule δ in this state, is
(3)
(3)
Here Es[]
d
is the expected allocation of patients to treatment B, across repeated samples.
Rule δ is admissible if there exists no rule such that
, Ps,
, Ps,
for all
and
, Ps,
>
, Ps,
for some s. The Bayes, maximin, and MR rules are as follows:
(4)
(4) where π is a subjective distribution on the state space.
(5)
(5)
(6)
(6)
3.2.2 Illustration: Choice Between a Status Quo Treatment and an Innovation When Outcomes Are Binary
To illustrate in perhaps the simplest nontrivial setting, let the outcomes y be binary, taking the value zero if treatment fails and one if it succeeds. Let A be a status quo treatment and B be an innovation. Suppose that the planner knows the success probability of the status quo treatment but not the success probability
of the innovation. The planner wants to choose treatments to maximize the success probability.
A randomized trial is performed to learn about outcomes under the innovation, with N subjects randomly drawn from the population and assigned to treatment B. The observed trial outcomes are that n subjects realize outcome y = 1 and N – n realize y = 0. In this setting, N indexes the sampling process and the number n of experimental successes is a sufficient statistic for the data.
The feasible STRs are functions δ(⋅): that map the number of experimental successes into a treatment allocation. The expected welfare of rule δ is
(7)
(7) n is distributed binomial B[β, N], so
(8)
(8)
where
is the probability of i successes. The only unknown determinant of expected welfare is β, so the state space S indexes the feasible values of β. Specifically,
[
1].
It is reasonable in this setting to conjecture that admissible treatment rules should be ones in which the fraction of the population allocated to treatment B increases with n. It turns out that the admissible treatment rules are a simple subclass of these rules. A theorem of Karlin and Rubin (Citation1956) shows that the admissible rules are the monotone treatment rules. Monotone rules assign all persons to the status quo if the experimental success rate is below some threshold and all to the innovation if the success rate is above the threshold. Thus, is admissible if and only if
(9a)
(9a)
(9b)
(9b)
(9c)
(9c) for some 0
and 0
1.
The collection of monotone treatment rules is a mathematically “small” subset of the space of all feasible treatment rules. Nevertheless, it still contains a broad range of rules. These include the following:
Data-Invariant Rules: These are the rules and
, which assign all persons to treatment A or B, respectively, whatever n may be.
Empirical Success Rule: An optimal treatment rule allocates all persons to treatment A if β <α and all to B if β >α. The empirical success rule emulates the optimal rule by replacing β with its sample analog, the empirical success rate n/N.
Bayes Rules: The form of the Bayes rule depends on the prior subjective distribution placed on β. Consider the class of Beta priors, which form the conjugate family for a Binomial likelihood. Let and let the prior be Beta with parameters (c, d). Then, the posterior mean for β is
. The resulting Bayes rule is
(10a)
(10a)
(10b)
(10b)
(10c)
(10c)
Maximin Rule: Minimum expected welfare for rule δ is(11)
(11)
Es[] > 0 for all βs >0 and for all monotone rules except
(⋅) = 0. When S contains states with βs <α, the maximin rule is δ(⋅) = 0. Thus, the maximin rule ignores the trial data on treatment response, whatever they may turn out to be. This illustrates the Savage (Citation1951) statement that using the maximin rule can induce one to entirely ignore available sample data.
Minimax-Regret Rule: The regret of rule δ in state s is(12)
(12)
Thus, regret is the mean welfare loss when a member of the population is assigned the inferior treatment, multiplied by the expected fraction of the population assigned this treatment. The minimax-regret rule does not have an analytical solution, but it can be determined numerically. When all values of β are feasible, the minimax-regret rule is well approximated by the empirical success rule.
3.2.3 Numerical Computation of the Minimax-Regret Rule for Small Samples
Manski (2007, Table 12.1) reports numerical computations of the minimax-regret rule for specified values of α and N when all values of β are feasible; that is, when . I reproduce the findings here in The top two panels display the value of (n0,
for this rule. The third panel displays the value of minimax regret.
Table 1 Minimax-regret rules for small sample sizes
The top panel of the table shows that the threshold n0 of experimental successes for allocation of persons to treatment B increases with the sample size and with the success probability of treatment A. The inequality |n0 – αN| 1 holds everywhere in the table. Thus, the minimax-regret rule is well approximated by an empirical success rule.
The third panel shows that the value of minimax regret decreases by roughly an order of magnitude as the sample size increases from to 10. For example, when 0.50, it falls from 0.25 to 0.027. Thus, even a sample size as small as 10 suffices to make maximum regret quite small.
3.3 Designing Trials to Enable Near-Optimal Treatment Choice
From the perspective of treatment choice, an ideal objective for the design of trials would be to collect data that enable subsequent implementation of an optimal treatment rule in the patient population of interest; that is, a rule for use of trial data that always selects the best treatment, with no chance of error. Optimality is too strong a property to be achievable with finite sample size. However, near-optimal rules—ones with small maximum regret—exist when classical trials are large enough.
Manski and Tetenov (Citation2016) investigated trial design that enables near-optimal treatment choices. It is shown that, given any ε > 0, ε -optimal rules exist when trials have large enough sample size. An ε-optimal rule has expected welfare, across repeated samples, within ε of the welfare of the best treatment in every state of nature. Equivalently, it has maximum regret no larger than ε.
The article considers trials that draw predetermined numbers of subjects at random within groups stratified by covariates and treatments. It reports exact results for cases of two treatments and binary outcomes; see Section 3.3.1 below for a summary. It gives sufficient conditions on sample sizes that ensure existence of ε-optimal treatment rules when there are multiple treatments and outcomes are bounded. These conditions are obtained by application of large deviations inequalities to evaluate the performance of empirical success rules.
Choosing sample size to enable existence of ε-optimal treatment rules requires specification of a value for ε. The selected ε determines how much deviation from optimality a decision maker is willing to tolerate when making treatment choices. The value of ε should be specified by clinical researchers concerned with patient care rather than by some universal convention. Clinical researchers may, perhaps, find it congenial to let ε equal the minimum clinically important difference (MCID) in the average treatment effect comparing alternative treatments.
Medical research has long distinguished between the statistical and clinical significance of treatment effects. While the idea of clinical significance has been interpreted in various ways, many writers call an average treatment effect clinically significant if its magnitude is greater than a specified value deemed minimally consequential in clinical practice. International Conference on Harmonisation (1999) put it this way (p. 1923): “The treatment difference to be detected may be based on a judgment concerning the minimal effect which has clinical relevance in the management of patients.”
3.3.1 Findings with Binary Outcomes, Two Treatments, and Balanced Designs
Determination of sample sizes that enable near-optimal treatment is simple in settings with binary outcomes (coded 0 and 1 for simplicity), two treatments, and a balanced design which assigns the same number of subjects to each treatment group. Manski and Tetenov (Citation2016, ), reproduced here as , provides exact computations of the minimum sample size that enables ε-optimality when a clinician uses one of three different treatment rules, for various values of ε.
Table 2 Minimum sample sizes per treatment enabling -optimal treatment choice: binary outcomes, two treatments, balanced designs
The first column shows the minimum sample size (per treatment arm) that yields ε-optimality when a clinician uses the empirical success (ES) rule to make a treatment decision. The ES rule chooses the treatment with the better average outcome in the trial. The rule assigns half the population to each treatment if there is a tie. It is known that the ES rule minimizes maximum regret in settings with binary outcomes, two treatments, and balanced designs (Stoye Citation2009).
The second and third columns display the minimum sample sizes that yield ε-optimality of rules based on one-sided 5 and 1% hypothesis tests. Decisions made with these tests take the two treatments to be a status quo and an innovation, choosing the innovation if the estimated treatment effect is positive and statistically significant. There is no consensus on what hypothesis test should be used to compare two proportions. Results are reported based on the widely used one-sided two-sample z-test, which is based on an asymptotic normal approximation (Fleiss Citation1973).
The findings are remarkable. A sample as small as two observations per treatment arm makes the ES rule ε-optimal when ε = 0.1 and a sample of size 145 suffices when ε = 0.01. The minimum sample sizes required for ε-optimality of the test rules are orders of magnitude larger. If the z-test of size 0.05 is used, a sample of size 33 is required when and 3488 when
. The sample sizes must be more than double these values if the z-test of size 0.01 is used. See Manski and Tetenov (Citation2016) for discussion of the factors that underlie these findings.
3.3.2 Implications for Practice
Based on their exact calculations and analytical findings using large-deviations inequalities, Manski and Tetenov conclude that sample sizes determined by clinically relevant near-optimality criteria tend to be much smaller than ones set by conventional statistical power criteria. Reduction of sample size relative to prevailing norms can be beneficial in multiple ways. Reduction of total sample size can lower the cost of executing trials, the time necessary to recruit adequate numbers of subjects, and the complexity of managing trials across multiple centers. Reduction of sample size per treatment arm can make it feasible to perform trials that increase the number of treatment arms and, hence, yield information about a wider variety of treatment options.
4 Conclusion
Science does not always progress monotonically. There are times when important, even fundamental, ideas are discovered and receive attention but then are neglected. This has occurred with statistical decision theory, which received considerable attention in the middle of the twentieth century but was largely forgotten by the century’s end. A revival in the context of treatment choice began in the early 2000s and has been gathering force. I hope that the growing dissatisfaction of statisticians with ritual applications of hypothesis testing, exemplified by the ASA Statement in Wasserstein and Lazar (Citation2016), will encourage statisticians to relearn statistical decision theory and use it when studying not only treatment choice with trial data but decision making with sample data more generally.
Acknowledgment
The author is grateful to the Editors and reviewers for their comments.
References
- Berger, J. (1985), Statistical Decision Theory and Bayesian Analysis (2nd ed.), New York: Springer.
- Berger, J. (2006), “The Case for Objective Bayesian Analysis,” Bayesian Analysis, 1, 385–402. DOI: 10.1214/06-BA115.
- Canner, P. (1970), “Selecting One of Two Treatments When the Responses Are Dichotomous,” Journal of the American Statistical Association, 65, 293–306. DOI: 10.1080/01621459.1970.10481080.
- Cheng, Y., Su, F., and Berry, D. (2003), “Choosing Sample Size for a Clinical Trial Using Decision Analysis,” Biometrika, 90, 923–936. DOI: 10.1093/biomet/90.4.923.
- DeGroot, M. (1970), Optimal Statistical Decisions, New York: McGraw-Hill.
- Dominitz, J., and Manski, C. (2017), “More Data or Better Data? A Statistical Decision Problem,” Review of Economic Studies, 84, 1583–1605.
- Ferguson, T. (1967), Mathematical Statistics: A Decision Theoretic Approach, San Diego, CA: Academic Press.
- Fisher, L., and Moyé, L. (1999), “Carvedilol and the Food and Drug Administration Approval Process: An Introduction,” Controlled Clinical Trials, 20, 1–15. DOI: 10.1016/S0197-2456(98)00052-X.
- Fleiss, J. (1973), Statistical Methods for Rates and Proportions, New York: Wiley.
- Garthwaite, P., Kadane, J., and O’Hagan, A. (2005), “Statistical Methods for Eliciting Probability Distributions,” Journal of the American Statistical Association, 100, 680–701. DOI: 10.1198/016214505000000105.
- Higgings, J., and Green, S. (eds.) (2011), Cochrane Handbook for Systematic Reviews of Interventions, Version 5.1.0, The Cochrane Collaboration, available at http://handbook-5-1.cochrane.org.
- Hirano, K., and Porter, J. (2009), “Asymptotics for Statistical Treatment Rules,” Econometrica, 77, 1683–1701.
- Hodges, E., and Lehmann, E. (1950), “Some Problems in Minimax Point Estimation,” Annals of Mathematical Statistics, 21, 182–197. DOI: 10.1214/aoms/1177729838.
- International Conference on Harmonisation, ICH E9 Expert Working Group (1999), “Statistical Principles for Clinical Trials: ICH Harmonized Tripartite Guideline,” Statistics in Medicine, 18, 1905–1942.
- Ioannidis, J. (2005), “Why Most Published Research Findings Are False,” PLoS Medicine, 2, 696–701.
- Karlin, S., and Rubin, H. (1956), “The Theory of Decision Procedures for Distributions with Monotone Likelihood Ratio,” Annals of Mathematical Statistics, 27, 272–299. DOI: 10.1214/aoms/1177728259.
- Kitagawa, T. and Tetenov, A. (2018), “Who Should be Treated? Empirical Welfare Maximization Methods for Treatment Choice,” Econometrica, 86, 591–616. DOI: 10.3982/ECTA13288.
- Koriat, A., Lichtenstein, S., and Fischhoff, B. (1980), “Reasons for Confidence,” Journal of Experimental Psychology: Human Learning and Memory, 6, 107–118. DOI: 10.1037//0278-7393.6.2.107.
- Manski, C. (2003), Partial Identification of Probability Distributions, New York: Springer.
- Manski, C. (2004a), “Statistical Treatment Rules for Heterogeneous Populations,” Econometrica, 72, 221–246.
- Manski, C. (2004b), “Measuring Expectations,” Econometrica, 72, 1329–1376.
- Manski, C. (2005), Social Choice With Partial Knowledge of Treatment Response, Princeton, NJ: Princeton University Press.
- Manski, C. (2007a), Identification for Prediction and Decision, Cambridge, MA: Harvard University Press.
- Manski, C. (2007b), “Minimax-Regret Treatment Choice With Missing Outcome Data,” Journal of Econometrics, 139, 105–115.
- Manski, C., and Tetenov, A. (2016), “Sufficient Trial Size to Inform Clinical Practice,” Proceedings of the National Academy of Sciences, 113, 10518–10523. DOI: 10.1073/pnas.1612174113.
- Morgan, G. and Henrion, M. (1990), Uncertainty: A Guide to Dealing With Uncertainty in Quantitative Risk and Policy Analysis, New York: Cambridge University Press.
- Parmigiani, G. and Inoue, L. (2009), Decision Theory: Principles and Approaches, New York: Wiley.
- Savage, L. (1951), “The Theory of Statistical Decision,” Journal of the American Statistical Association, 46, 55–67. DOI: 10.1080/01621459.1951.10500768.
- Savage, L. (1971), “Elicitation of Personal Probabilities and Expectations,” Journal of the American Statistical Association, 66, 783–801. DOI: 10.1080/01621459.1971.10482346.
- Schlag, K. (2006), “Eleven Tests Needed for a Recommendation,” European University Institute Working Paper ECO No. 2006/2.
- Spiegelhalter, D., Freedman, L., and Parmar, M. (1994), “Bayesian Approaches to Randomized Trials” (with discussion), Journal of the Royal Statistics Society, Series A, 157, 357–416. DOI: 10.2307/2983527.
- Spiegelhalter, D. (2004), “Incorporating Bayesian Ideas Into Health-Care Evaluation,” Statistical Science, 19, 156–174. DOI: 10.1214/088342304000000080.
- Stoye, J. (2009), “Minimax Regret Treatment Choice With Finite Samples,” Journal of Econometrics, 151, 70–81. DOI: 10.1016/j.jeconom.2009.02.013.
- Stoye, J. (2012), “Minimax Regret Treatment Choice With Covariates or With Limited Validity of Experiments,” Journal of Econometrics, 166, 138–156.
- Tetenov, A. (2012), “Statistical Treatment Choice Based on Asymmetric Minimax Regret Criteria,” Journal of Econometrics, 166, 157–165. DOI: 10.1016/j.jeconom.2011.06.013.
- U.S. Food and Drug Administration (2010), Guidance for the Use of Bayesian Statistics in Medical Device Clinical Trials. Available at https://www.fda.gov/MedicalDevices/ucm071072.htm.
- Wald, A. (1950), Statistical Decision Functions, New York: Wiley.
- Wasserstein, R., and Lazar, N. (2016), “The ASA’s Statement on p-Values: Context, Process, and Purpose,” The American Statistician, 70, 129–133. DOI: 10.1080/00031305.2016.1154108.