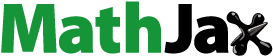
Abstract
A crisis of validity has emerged from three related crises of science, that is, the crises of statistical significance and complete randomization, of replication, and of reproducibility. Guinnessometrics takes commonplace assumptions and methods of statistical science and stands them on their head, from little p-values to unstructured Big Data. Guinnessometrics focuses instead on the substantive significance which emerges from a small series of independent and economical yet balanced and repeated experiments. Originally developed and market-tested by William S. Gosset aka “Student” in his job as Head Experimental Brewer at the Guinness Brewery in Dublin, Gosset’s economic and common sense approach to statistical inference and scientific method has been unwisely neglected. In many areas of science and life, the 10 principles of Guinnessometrics or G-values outlined here can help. Other things equal, the larger the G-values, the better the science and judgment. By now a colleague, neighbor, or YouTube junkie has probably shown you one of those wacky psychology experiments in a video involving a gorilla, and testing the limits of human cognition. In one video, a person wearing a gorilla suit suddenly appears on the scene among humans, who are themselves engaged in some ordinary, mundane activity such as passing a basketball. The funny thing is, prankster researchers have discovered, when observers are asked to think about the mundane activity (such as by counting the number of observed passes of a basketball), the unexpected gorilla is frequently unseen (for discussion see Kahneman Citation2011). The gorilla is invisible. People don’t see it.
1 Invisible Giant of Statistics Inside The Archives (And Out)
I am an economic statistician and historian who has studied the history of statistical significance and experimental design in what is considered by most people to be a foreign and ancient land—the archives, that is, historical archival libraries—for nearly 25 years. I’ve logged countless hours in some of the grandest and oldest reading rooms and their opposites in England, Ireland, and the United States. On and off since 2008, my research efforts have been especially focused in Dublin, Ireland, primarily in the archives of the Guinness Storehouse Museum (wicked, I know: nice work if you can get it).
I have found by comparing the archives with published literature a similar pattern in the history of statistics, an “unseeing” of a statistical giant whose methods, when heeded, could help out much of the economy, from Big Pharm and agri-business to the World Bank and higher education, more or less instantly. Statisticians and their clients have narrowed their focus on the wrong thing, the back and forth of statistical significance testing, “pass” or “no pass”. We have become so routinized in our focus on bright-line significance levels such as p < 0.05 (an example of what the behavioral economist Daniel Kahneman calls “thinking fast”) that we are often blinded to larger, perhaps more important things—such as estimation of the substantive meaning of our results (requiring “slower”, deliberate thought).
In fact, I argue that this unseen giant of statistics holds the keys for unlocking our current crises in science: the crises of replication, of reproducibility, and of statistical significance; in sum, close study of this giant’s methods can help to undo the crisis of validity currently haunting science and society, including business and law (Goodman Citation2002; Ziliak and McCloskey Citation2008; Greenland, et al. Citation2016; Wasserstein and Lazar, Citation2016; Pierson, Broman, et al. 2017).
2 How a Giant Statistician and Brewer Sidestepped the Crisis of Validity
Who is this invisible giant with a hero’s cape? “Student” is the name which graces Student’s t, the most popular test of statistical significance in the world, and the foundation from which Fisher’s p-values were originally derived (Student Citation1908a, Citation1925; Fisher 1925; Ziliak Citation2008). Most statisticians have heard that “Student” is in reality the pen name of William Sealy Gosset (1876–1937), a brewer of Guinness beer.1
But even professional statisticians struggle to distinguish “Student’s” actual methods from those of Fisher, erroneously conflating the ideas and opinions of the two men (Ziliak and McCloskey (Citation2008), Chaps. 20–23). The much older and far more experienced “Student”, who had been comparing random with balanced layouts in agricultural plots since 1905 was not, as some observers seem to think, just another “Fisher”-replicate donning a brewer’s costume, a minor figure appearing on the scene to spice up a boring statistics lecture. Brewing and shipping unpasteurized beer for profit, taste, and quality assurance on the global scale is serious business—the economic element is fundamental, and a lot can be lost, “Student” knew and warned. Conflation of Student’s methods with the admittedly hackneyed mathematical and antieconomic re-interpretation of them by Fisher (1925, Citation1933, Citation1935) and most other textbook authors is both a historical blind spot and scientific blunder with, as I have shown elsewhere, enormous practical and human consequences, many of them far from good.2 I call this valuable if neglected approach to statistics, “Guinnessometrics”.
3 G-Values: The 10 Principles of Guinnessometrics
Guinnessometrics is an experimental philosophy of inference and decision-making innovated and market-tested between 1904 and 1937 by William S. Gosset, a self-trained statistician who rose to Head Brewer of Guinness during the decades when Guinness was the largest brewery in the world (Ziliak Citation2008). At Guinness the scientific brewers, including Gosset, were allowed by the company to publish research so long as they did not mention (1) beer, (2) Guinness, or (3) their own surname. Ironically the hundred-million gallon a year brewery did not rely on statistical significance, Student’s t, randomized trials, or Big Data. Closer to the opposite. Guinnessometrics takes a repeated small-sample economic approach to experimental statistics and decisions, in cooperation with agents up and down supply chains, all with real “skin in the game” (Taleb Citation2018). Gosset’s Guinnessometrics inverts the usual matrix of statistical science and emerges with little or no need for a p-value or placebo-controlled randomized trial. And as any Guinness drinker can tell you, results of this science are not only repeatable and replicable, they are reproducible. Crisis averted.
The crisis of validity in the statistical sciences has been caused largely, though not entirely, by the following common yet erroneous practices undermining trust in data-based decisions:
Attempted falsification of an assumed-to-be true null hypothesis without a loss function;
Statistical significance testing at a bright line level (such as p < 0.05 or t > 1.96) independent of the substantive meaning of the result, coefficient, or model;
Running a randomized, placebo controlled trial (RCT) assuming the independence of observations;
Making decisions based on one, large-scale experiment, random or not (“One and done”);
Accepting or rejecting a hypothesis based on a single sample of convenience and arbitrary size (the majority of social science, and much of life science);
Promoting what turns out to be irreproducible results;
Investing prematurely in “Big Data”;
Assuming in statistical tests there is “no prior” subject-matter knowledge; and
Making false binary and merely qualitative assessments based on the alleged bright line “significance” or “insignificance” of a result (important/unimportant, yes/no, exists/does not exist)
Guinnessometrics reverses these misleading yet widespread practices and replaces them with 10 principles or G-values, just as Gosset always said. In listicle form, the Ten Principles of Guinnessometrics are:
G-10 Consider the Purpose of the Inquiry, and Compare with Best Practice
Falsification of a null hypothesis is not the main purpose of the experiment or observational study. Making money or beer or medicine—ideally more and better than the competition and best practice—is. Estimating the importance of your coefficient relative to results reported by others, is. To repeat, as the 2016 ASA Statement on Statistical Significance and P-values makes clear, merely falsifying a null hypothesis with a qualitative yes/no, exists/does not exist, significant/not significant answer, is not itself significant science, and should be eschewed.
G-9 Estimate the Stakes (or Eat Them)
Estimation of magnitudes of effects, and demonstrations of their substantive meaning, should be the center of most inquiries. Failure to specify the stakes of a hypothesis is the first step toward eating them (gulp).
G-8 Study Correlated Data: ABBA, Take a Chance on Me
Most regression models assume “i.i.d.” error terms—independently and identically distributed—yet most data in the social and life sciences are correlated by systematic, nonrandom effects—and are thus not independent. Gosset solved the problem of correlated soil plots with the “ABBA” layout, maximizing the correlation of paired differences between the As and Bs with a perfectly balanced chiasmic arrangement (Ziliak Citation2014).
G-7 Minimize “Real Error” with the 3 R’s: Represent, Replicate, Reproduce
A test of significance on a single set of data is nearly valueless. Fisher’s p, Student’s t, and other tests should only be used when there is actual repetition of the experiment. “One and done” is scientism, not scientific. Random error is not equal to real error, and is usually smaller and less important than the sum of nonrandom errors. Measurement error, confounding, specification error, and bias of the auspices, are frequently larger in all the testing sciences, agronomy to medicine. Guinnessometrics minimizes real error by repeating trials on stratified and balanced yet independent experimental units, controlling as much as possible for local fixed effects.
G-6 Economize With “Less Is More”: Small Samples of Independent Experiments
Small-sample analysis and distribution theory has an economic origin and foundation: changing inputs to the beer on the large scale (for Guinness, enormous global scale) is risky, with more than money at stake. But smaller samples, as Gosset showed in decades of barley and hops experimentation, does not mean “less than”, and Big Data is in any case not the solution for many problems.
G-5 Keep Your Eyes on the Size Matters/How Much? Question
There will be distractions but the expected loss and/or profit functions rule, or should. Are regression coefficients or differences between means large or small? Compared to what? How do you know?
G-4 Visualize
Parameter uncertainty is not the same thing as model uncertainty. Does the result hit you between the eyes? Does the study show magnitudes of effects across the entire distribution? Advances in visualization software continue to outstrip advances in statistical modeling, making more visualization a no brainer.
G-3 Consider Posteriors and Priors too (“It pays to go Bayes”)
The sample on hand is rarely the only thing that is “known”. Subject matter expertise is an important prior input to statistical design and affects analysis of “posterior” results. For example, Gosset at Guinness was wise to keep quality assurance metrics and bottom line profit at the center of his inquiry. How does prior information fit into the story and evidence? Advances in Bayesian computing software make it easier and easier to do a Bayesian analysis, merging prior and posterior information, values, and knowledge.
G-2 Cooperate Up, Down, and Across (Networks and Value Chains)
For example, where would brewers be today without the continued cooperation of farmers? Perhaps back on the farm and not at the brewery making beer. Statistical science is social, and cooperation helps. Guinness financed a large share of modern statistical theory, and not only by supporting Gosset and other brewers with academic sabbaticals (Ziliak and McCloskey Citation2008, Chp. 22). And last but not least:
G-1 Answer the Brewer’s Original Question (“How Should you set the odds?”)
No bright-line rule of statistical significance can answer the brewer’s question. As Gosset said way back in 1904, how you set the odds depends on “the importance of the issues at stake” (the expected benefit and cost, for example) together with the cost of obtaining new material.
No one could plausibly claim that the 10 G-values are the end-all, be-all of statistical science. Only that for a great variety of business, medical, and scientific purposes, the Guinnessometric approach to data and decision-making answers far more questions, and far more satisfactorily, than the conventional, unrepeated observational study or placebo controlled RCT judged by the level of a p-value (compare Ziliak Citation2010a, Citation2010b; Senn 2010).
4 How to Get Large G-Values
Gosset’s routine was to produce as many of the 10 G-values as possible, and to labor at them at maximum possible strength, so long as doing so does not subtract too much from one or more of the other G-values. We briefly illustrate below the value of each G-value. For example, throwing too many resources into G-value Number 7 by replicating too much (such as by spending $10 million on a 12th replication of the overrated “invisible gorilla” experiment made famous by YouTube) would encroach too negatively on G-value Number 6: the need to “Economize.” Economizing in Gosset’s sense means studying a series of small but independent and well-structured samples (rather than plunking down millions for Big, unstructured Data, today’s fashion) to learn about regression input X or dependent variable Y. It should be said that the validity of G-values is completely general and does not depend on whether or not one fancies a beverage by Guinness. Thus:
5 G-10 Consider The Purpose Of The Inquiry, And Compare With Best Practice
Falsification of a null hypothesis is not, we have said, the “purpose” of a study or experiment helped along by statistical methods. Saving lives or money or malted barley, or advancing the health and wealth of schools and nations, is. In his magisterial Theory of Probability Harold Jeffreys (1961 [1961], p. 377) wrote in agreement with Gosset that the null test-and-p-value procedure advocated by the Fisher School “is merely something to set up like a coconut to stand until it is hit.” “Hence the hypotheses made by “Student” are completely equivalent to mine; they have merely been introduced in a different order” (Jeffreys 1961, p. 380).
Presumably there is prior interest, otherwise, why test? Why invest in an experiment that is thought in advance to make no difference whatsoever? In their hearts, most investigators do not. But in their statistical science most are in Jeffreys’s sense dedicated coconut swatters.
Whatever the purpose of the experiment, best practice research compares a novel treatment or variable with best practice and/or prevailing wisdom, not with an assumed-to-be-true null hypothesis or blank placebo. At the largest brewery in the world measured by annual output and sales, Gosset was determined, indeed he was incentivized, to mash the beer, not a low hanging coconut. At Guinness, he could not afford to spend his days taking whacks at easy to hit coconuts. At stake was nothing less than 100 million gallons of Guinness stout produced and sold annually by one of the most recognized brands in the world, first established by Arthur Guinness in 1759.
In an important letter of 1905, Gosset told Karl Pearson that one can “aim at” the odds of attaining some result by way of repeated experimentation. He told Pearson he decided that one cannot judge the “significance” of results—or decide a course of business action—without, in effect, employing some scale of human values capable of balancing the utility of expected gains against the disutility of losses (Gosset Citation1905). The point is fundamental:
When I first reported on the subject [of “The Application of the ‘Law of Error’ to the Work of the Brewery” (1904)], I thought that perhaps there might be some degree of probability which is conventionally treated as sufficient in such work as ours and I advised that some outside authority [in mathematics, such as Karl Pearson] should be consulted as to what certainty is required to aim at in large scale work. However it would appear that in such work as ours the degree of certainty to be aimed at must depend on the pecuniary advantage to be gained by following the result of the experiment, compared with the increased cost of the new method, if any, and the cost of each experiment.
Gosset upped the odds of farming and brewing success by designing and repeating small samples of stratified and balanced experiments, reducing what he called the “real error.” The object or purpose of an experiment has little or nothing to do with the “significance” of a null hypothesis in and of itself, independent of some scale of values and range of action.
In a seminal Biometrika article “On Testing Varieties of Cereals” Gosset wrote (Student Citation1923):
The object of testing varieties of cereals is to find out which will pay the farmer best. This may depend on quality, but in general it is an increase of yield which is profitable, and since yield is very variable from year to year and from farm to farm it is a difficult matter upon which to obtain conclusive evidence.
Citing his own research undertaken in cooperation with the Irish Department of Agriculture and Technical Instruction (IDATI), Gosset continued:
Yet it is certain that very considerable improvements in yield have been made as the result of replacing the native cereals by improved varieties; as an example of this I may cite the case of Ireland, where varieties of barley have been introduced which were shown by experiment [since 1898] to have an average yield of 15 to 20% above those which they replaced. This represents, probably a gain to the country of not less than £250,000 per year. As the cost of experiments from the commencement to the present time [namely, about 25 years] cannot have reached £40,000 the money has been well spent.
In the same article Gosset told the assembled at the Royal Statistical Society—including Fisher, Neyman, and Egon Pearson—that the net gain to Ireland alone after 25 years of continuous experimentation using balanced (not RCT) designs was approximately:
Gain: + £250,000 per year × 25 years
Cost: - £40,000
That is, a ballpark net gain = £6.21 million on a £40,000 investment
In his last published paper, “Comparison of Random and Balanced Arrangements of Field Plots,” Gosset said (Student Citation1938, p. 206):
I personally choose the method which is most likely to be profitable when designing the experiment rather than use Prof. Fisher’s system of a posteriori choice* which has always seemed to me to savour rather too much of “heads I win, tails you lose.”
6 G-9 Estimate The Stakes (Or Eat Them)
Thus, the purpose of an experiment puts something substantive at stake—a belief, an input, a technology, or policy variable. The purpose of the experiment is not to reject a null hypothesis; the purpose of a study is usually not even “statistical” in meaning. Science is substantive. Its meaning lies in magnitudes of bees, or beer, or basketball, of love, or family, or famous movie stars. Not in columns and rows of Student’s t or Fisher’s p.
The stakes of a study are normally speaking the substantive stakes of life—a gamble on a new pill or product or pilot study—with expected magnitudes of gains and losses expressed in the form of an expected loss function (Manski Citation2018, Press 2005), Jeffreys’s minimum difference (Jeffreys 1961), a Ramsey bet (Ramsey Citation1926) or Gosset gamble (Gosset Citation1905) on a scale of values not captured by tables of probability alone. As Ramsey (Citation1926, p. 51) wrote in “Truth and Probability”:
We all agree that a man who did not make inductions would be unreasonable: the question is only what this means.
Like Gosset, Ramsey believed that probability “is a measurement of belief qua basis of action.”3 Quantities of substantive gain or loss are central to the Guinnessometric approach. The much-admired Frank Plumpton Ramsey (Citation1926)—who by chance had attended the same school as Gosset (Winchester College) and studied mathematics with one of Gosset’s friends (the mountain-climber, Robert Irving)—observed that the “expected value” of an experiment or a set of observations is not a reliable guide for action. (Most economists first learned the point from Savage, though Savage credits Ramsey.) Expected value is an unreliable metric for gauging human behavior, though many social and life scientists interpret regression models as if. As the stakes rise, or as the gamble is rephrased or otherwise altered rhetorically and thus psychologically, risk taking behavior changes, Ramsey perceived. And this fact has led economists and statisticians to favor “expected utility” over expected value (in statistics, see Lindley (Citation1991) and Press (2005); also see Taleb (Citation2018) for the weakness of utility under “ruin” probabilities). The expected value of something is the sum of all the possible outcomes (in nominal terms) weighted by their respective probabilities of occurrence.
It is easy to see Ramsey’s point in a comparison of three different gambles subject to one random flip of a fair coin (illustrated by Frank Citation2017, pp. 179–180):
Gamble 1: If a coin flip shows “heads”, win $100; if tails, lose $0.50
Gamble 2: If a coin flip shows “heads”, win $200; if tails, lose $100
Gamble 3: If a coin flip shows “heads”, win $20,000; if tails, lose $10,000.
Now Gamble 1 would be accepted by many. Assuming as convention does that the probability of heads and tails are equal (at 1/2 each) the expected value of the gamble is (1/2)(win $100) + (1/2)(lose $0.50) for an expected win of $49.75. The worst case scenario is “lose 50 cents”, which many would be willing to risk for an equal chance at winning $100.
Gamble 2 will draw fewer contestants, though many will still accept the gamble. Gamble 3 has the highest expected value but this bet would be rejected by many (though not all) even though the payoff structure is identical to Gamble 2 (both have a 2-to-1 win-loss ratio). The reason is that, although winning $20,000 could be lovely, losing $10,000 from a single coin flip could be tragic.
Suppose now that subjects are expected utility maximizers instead of expected value maximizers. Utility theory has problems and limitations, too, but it offers something more. Expected utility is a scale of values which accounts for, among other things, attitudes toward risk and the diminishing marginal utility of money (for example Savage Citation1954; Lindley Citation1991; Press 2005). Suppose you are a conventional risk-averse person with a strictly concave utility function, such as the square-root function, which is concave in money accumulation (EU = √ money).
Your initial amount of money (or wealth) is $10,000. What is the expected utility of each of the three gambles? Notice that the “value” ranking of the gambles is now reversed.
The expected utility of Gamble 1 is highest even though its expected value is lowest ((1/2)(√ 10,100) + (1/2)(√ 9999.50) = 100.248 utils) while the expected utility of Gamble 3 is lowest even though its expected value is by far the highest (= 86.60 utils). “The old established way of measuring a person’s belief is to propose a bet, and see what are the lowest odds which he will accept. This method I regard as fundamentally sound” (Ramsey Citation1926, p. 34). “[B]ut it suffers from being insufficiently general, and from being necessarily inexact. It is inexact partly because of the diminishing marginal utility of money, partly because the person may have a special eagerness or reluctance to bet, because he either enjoys or dislikes excitement or for any other reason, e.g., to make a book” (Ramsey, pp. 34–35).
Yet from the point of view of a statistical scientist who is charged with practical estimation and interpretation in a particular context, Ramsey’s approach is backward way around, and too abstract. What Gosset’s expected loss function approach lacks in abstract shine, it gains in economic profit and plain common sense.
7 G-8 Study Correlated Data: Abba, Take a Chance on Me
Completely randomized studies, where treatment and control groups are determined as if by random coin flip, are now fashionable. Yet, statisticians have long known that stratification or blocking adds precision and efficiency to a study otherwise based on complete randomization. Gosset (Student 1911) used blocking or stratification long before the synonymous words existed in the statisticians’ vocabulary. He called his balanced approach to field layouts, “ABBA,” the closely arranged mirror pattern of the layout, treatments, and controls, the As and Bs being compared. Student (Citation1923, p. 273) said:
The art of designing all experiments lies even more in arranging matters so that ρ [the correlation coefficient] is as large as possible than in reducing and
[the variance].
The peculiar difficulties of the problem lie in the fact that the soil in which the experiments are carried out is nowhere really uniform; however little it may vary from eye to eye, it is found to vary not only from acre to acre but from yard to yard, and even from inch to inch. This variation is anything but random [Gosset himself noted], so the ordinary formulae for combining errors of observation which are based on randomness are even less applicable than usual.
As Deming (1938, p. 879), an admirer of Gosset, noted: “Stratification is equivalent to blocking in the design of an experiment.” Box, Hunter, and Hunter (2005, p. 92) explain that “A block is a portion of the experimental material (the two shoes of one boy, two seeds in the same pot) that is expected to be more homogenous than the aggregate (the shoes of all the boys, all the seeds not in the same pot). By confining comparisons to those within blocks (boys, girls), greater precision is usually obtained because the differences associated between the blocks are eliminated.”
Deming, who before turning to manufacturing did a long stint as researcher at the U.S. Department of Agriculture, agreed with Gosset’s nonrandom point: random sampling and randomized experiments are at best preliminary steps to scientific study. Complete randomization has a purpose when the investigator knows little or nothing at all about strata or when the cost of being wrong is negligible. Said Deming (p. 879):
The primary aim of stratified sampling is to increase the amount of information per unit of cost. A further aim may be to obtain adequate information about certain strata of special interest. One way to carry out stratification is to rearrange the sampling units in the frame so as to separate them into classes, or strata, and then to draw sampling units from each class. The goal should be to make each stratum as homogeneous as possible, within limitations of time and cost.4
Likewise in his book, Planning of Experiments, David Cox (1958) recommends “completely randomized arrangement …[only] in experiments in which no reasonable grouping into blocks suggests itself”—that is, when ignorance prevails, or priors are flat.
Normally speaking, ignorance does not prevail, and real economic and statistical gains can be found by stratifying. Deming (1938) and Tippett (1952) simplified Student’s (1911, 1923) proof that stratification (blocking) can reduce sample size requirements by 40% or more, holding variance constant.5 And as Tippett noted, “At the worst”—assuming the rare case that calculated variance between strata is zero—“sampling in strata is no better than random sampling, but it is never worse.”
8 G-7 Minimize “Real Error” With The 3 R’S: Represent, Replicate, Reproduce
Recently the ASA has adopted the following widely used definitions of reproducibility and replicability in a set of “Recommendations” designed to assuage the reproducibility “crisis” which is currently being observed throughout the sciences (Pierson, Broman, et al. 2017):
Reproducibility: A study is reproducible if you can take the original data and the computer code used to analyze the data and reproduce all of the numerical findings from the study. This may initially sound like a trivial task but experience has shown that it’s not always easy to achieve this seemingly minimal standard.
For Gosset and Guinnessometrics, “reproducibility” is the ability to brew Guinness stout or ale (Smithwick’s) in such a way as to taste and to otherwise behave the same, pint after pint, gallon after gallon, millions of times over worldwide.
Replicability: This is the act of repeating an entire study, independently of the original investigator without the use of original data (but generally using the same methods)
In 35 years of barley yield and quality trials, Gosset and Guinness commissioned experiments with Irish barley farmers scattered across the different barley growing regions of the country. On average there were 10 farmers running simultaneously the same or nearly identical experimental design on new barley (treatment) and one or more “old” barley. And for insurance each planted a replicate locally, thus there were approximately 20 replications in the average year.]
These definitions suit well the Guinnessometric practice of replication and reproducibility. Gosset explained the 3 R’s of minimum real error in a letter of April 1937 to Egon S. Pearson, who was his close friend and editor of Biometrika (quoted in Pearson Citation1939, pp. 247–248):
Many thanks for yours of [April] 10th; I feel I’m wasting your time but as long as you ask questions you must expect to get answers …Now I was talking about Cooperative experiments and obviously the important thing in such is to have a low real error, not to have a “significant” result at a particular station. The latter seems to me to be nearly valueless in itself. Even when experiments are carried out only at a single station, if they are not mere five finger exercises, they will have to be part of a series in time …. But in fact experiments at a single station [that is, tests of statistical significance on a single set of data] are almost valueless; you can say “In heavy soils like Rabbitsbury potatoes cannot utilise potash manures”, but when you are asked “What are heavy soils like Rabbitsbury?” you have to admit—until you have tried elsewhere—that what you mean is “At Rabbitsbury etc.” And that, according to X may mean only “In the old cow field at Rabbitsbury”. What you really want to find out is “In what soil and under what conditions of weather do potatoes utilise the addition of potash manures?”{PRIVATE}
To do that you must try it out at a representative sample of the farms of the country and correlate with the characters of the soil and weather. It may be that you have an easy problem, like our barleys which come out in much the same order whatever–in reason–you grow them or like Crowther’s cotton which benefitted very appreciably from nitro-chalk in seven stations out of eight, but even then what you really want is a low real error. You want to be able to say not only “We have significant evidence that if farmers in general do this they will make money by it”, but also “we have found it so in nineteen cases out of twenty and we are finding out why it doesn’t work in the twentieth”. To do that you have to be as sure as possible which is the 20th—your real error must be small (emphasis added).
Representation, the third “R,” we can define in a number of different ways but something like this: coverage and stratification of all systematic sources of fluctuation, whether the source be a temporal, a spatial, or other exogenous force (such as weather: rainy or not rainy, or soil quality: loamy or clay). These definitions work as a first approximation. It’s not about random error: that’s the main point. We are trying to minimize and control for the systematic errors, which are of larger importance and number than are the assumed-to-be “random sampling errors.” These are the 3 R’s of Guinnessometrics and G-value No. 7.
The reason for G-value Number 7, the 3 R’s of Minimum Real Error is simple: the out-of-sample experience of life is difficult to predict, and for both systematic and random reasons. Unless you are a late night psychic broadcasting on cable television to well sedated individuals, you do not hold the crystal ball for ensuring external validity and economic profit should we decide to “scale up” from an unbalanced, un-stratified village-level experiment on eyeglasses or to nets to, let’s say, a whole nation (the lack doesn’t stop some from donning a purple scarf at The World Bank: Banerjee and Duflo Citation2011; Glewwe et al, Citation2012).
Yet most statistical studies in the social sciences, economics and psychology included, and many more in health and medicine are of the “one and done” variety: one RCT (which assumes independence) is conducted on a single sample; one approach is taken to regression modeling, followed by 100 tests of significance on the data using NHST and p-values on the single sample on offer. P-hack until you publish the paper (Ziliak and Teather-Posadas Citation2016 document the ethical side of this). This treating of single samples as if they are repeated samples is old news to older psychologists, many of whom remember the great Sterling (1959) survey showing that the probability of replication decreased with the level of statistical significance (the lower the p value, the less likelihood the study will be replicated).
From 1901 until the start of World War II, Guinness (and thus Gosset) invested heavily in the 3 R’s of G-values: that is by repeating annually a small series of independent, representative, and balanced experiments (what sociologists call repeated, stratified-random samples). The positive results of the Guinness and Irish investment are nothing short of astonishing, with one of the byproducts being that Gosset invented or inspired half of the toolkit of modern statistics and experimental science.
9 G-6 Economize With “Less is More”: Small Samples of Independent Experiments
Replication and reproduction does not always require millions of dollars in grant money, though at the NIH, NSF, and elsewhere it can.
Small samples are an economic and scientific choice, not a mathematical problem to be solved in abstract mathematical terms. For example, in a small-sample analysis, a brewer may wish to know with 10 to 1 or better odds how many samples of malt extract he needs to mix to be confident that the saccharine level of the beer stays within 0.5 degrees of the 133 degree standard he is targeting. The example is “Student’s”: brewing over 100 million gallons of Guinness stout per annum, “Student” and Guinness stakeholders needed to know (Ziliak Citation2008, p. 206). “Real” errors in this context include uneven temperature changes, heterogeneous barley malt, and mismeasurement of saccharine levels—adding up to more error than is allegedly described by p or t.
Student rejected artificial rules about significance from the beginning of his inquiries at the Brewery—at least four years before he published the first table and small sample test of significance (Gosset Citation1904; Student Citation1908a). In November, 1904, Gosset—he would not be known as Student until three years later—discussed his first break-through on the economic meaning of statistical significance, in an internal report titled “The Application of the ‘Law of Error’ to the Work of the Brewery.” The Apprentice Brewer said:
Results are only valuable when the amount by which they probably differ from the truth is so small as to be insignificant for the purposes of the experiment. What the odds should be depends —
On the degree of accuracy which the nature of the experiment allows, and
On the importance of the issues at stake.
Comparing the level of saccharine content in a series of malt extracts which he and others’ mixed in the Experimental Brewery with that found in malts being used in the Main Brewery, Gosset brought attention to a positive correlation he found between “the square root of the number of observations”— that is, the number of calculated differences in saccharine content between Experimental and Main Brewery malts—and the level of statistical significance. Other things equal, he said “the greater the number of observations of which means are taken [the larger the sample size of extract differences], the smaller the [probable or standard] error” of the estimates. “And the curve which represents their frequency of error,” he showed in a graph and plot drawing, “becomes taller and narrower.”
Prior to Gosset the relation between sample size and the level of statistical significance was rarely explored. For example, while looking at biometric samples with up to thousands of observations, Karl Pearson declared that a result departing by more than three standard deviations is “definitely significant.” The normal tables assumed very large samples. Yet Gosset, self-trained in statistics, found by experiment that at such large samples nearly everything is statistically “significant”— though not, in Gosset’s terms, economically or scientifically “important”. And, likewise, Gosset found that a small number of observations can be profitable, though not statistically significant in Pearson’s conventional sense. Regardless, Gosset did not have the luxury of large samples. One of his earliest experiments employed a sample size of n = 2, which helps to explain why in the original 1908 article Gosset calculated a z statistic for n = 2 (Student Citation1908a).
His 1904 article is worth exploring a bit further – especially for the econometrician and real-world firm that wants to earn more with less. Guinness malt was produced in Gosset’s time primarily from Irish and English barley stock—Old Irish, Prentice, Plumage Archer, and Spratt Archer were effective varieties. Malt extract was measured by “degrees saccharine” per barrel of 168 pounds malt weight.
An extract in the neighborhood of 133 degrees saccharine gave the targeted level of alcohol for Guinness’s beer. A much higher degree of saccharine would affect the stability and life of the beer, but it also increases alcohol content—which in turn increases the excise tax which Guinness owes to the British government, which—sad to say—ups the price of Dad’s pint. If, on the other hand, the alcohol content comes in too low, if the degree of saccharine is insufficient, customers would riot, or switch to Beamish and Beck’s. In Gosset’s view, +/– 0.5 degrees saccharine was a difference or error in malt extract which Guinness and its customers could swallow. “It might be maintained,” he said, “that malt extract “should be [estimated] within 0.5 of the true result with a probability of 10 to 1.” Gosset calculated the odds of observing the stipulated accuracy for small and then large numbers of extracts. He found that:
Odds in favour of smaller error than 0.5 [are with:]
2 observations 4:1
3 “7:1
4 “12:1
5 “19:1
82 “practically infinite
Thus, Gosset concluded, “In order to get the accuracy we require [that is, 10 to 1 odds with 0.5 accuracy], we must, therefore, take the mean of [at least] four determinations.” The Guinness Board cheered. The Apprentice Brewer found an economical way to assess the behavior of population parameters, using very small samples.
Small samples and their analysis originate from a fundamental economic cause: scarcity—the economic scarcity and expense of gaining new information about barley, malt, hops, and other beer inputs. Thus, one can say in general that Gosset took an economic approach to the logic of uncertainty, from the choice of sample size on up.
10 G-5 Keep Your Eyes on The Size Matters/How Much? Questions
We’re all in search of that “Goldilocks” zone, not too high, not too low; not too hot, not too cold, et cetera. In 1995, some cancer epidemiologists made history (discussed by Ziliak and McCloskey (Citation2008), pp. 184–186). The authors of 10 independent and randomized clinical trials involving thousands of patients in treatment and control groups had come to an agreement on an effect size. Consensus on a mere direction of effect—up or down, positive or negative—is rare enough in science. After four centuries of public assistance for the poor in the United States and Western Europe for example, economists, do not speak with one voice on the direction of effect on labor supply exerted by tax-financed income subsidies. Medicine is no different. Disagreement on the direction of effect—let alone the size of effect—is more rule than exception.
So the Prostate Cancer Trialists’ Collaborative Group was understandably eager to publicize the agreement. Each of the 10 studies showed that a certain drug “flutamide” —for the treatment of prostate cancer—can increase the likelihood of patient survival by an average of 12% (the 95% confidence interval in the pooled data put an upper bound on flutamide-enhanced survival at about 20% [Rothman, Johnson, and Sugano Citation1999]). Odds of 5 in 100 is not the best news to deliver to a prostate patient. But if castration followed by death is the next best alternative, a noninvasive 12-to-20% increase in survival sounds good.
But in 1998 the results of still another, 11th trial were published in the New England Journal of Medicine (Eisenberger et al. Citation1998, pp. 1036–1042). The authors of the new study found a similar size effect. But when the two-sided p value for their odds ratio came in at .14 they dismissed the efficacious drug, concluding “no clinically meaningful improvement” (pp. 1036, 1039). Kenneth Rothman, Eric Johnson, and David Sugano examined the individual and pooled results of the 11 separate studies, including the study conducted by Eisenberger et al.
One might suspect that [Eisenbergers et al.’s] findings were at odds with the results from the previous ten trials, but that is not so. From 697 patients randomised to flutamide and 685 randomised to placebo, Eisenberger and colleagues found an OR of 0.87 (95% CI 0.70–1.10), a value nearly identical to that from the ten previous studies. Eisenberger’s interpretation that flutamide is ineffective was based on absence of statistical significance. (Rothman, Johnson, and Sugano Citation1999, p. 1184)
Rothman and coauthors display the flutamide effect graphically in a manner consistent with a Gosset-Deming-and-Savage approach to visualization. Does the effect hit you between the eyes? Does it cause interocular trauma? Rothman and others pool data from the separate studies and plot the flutamide effect (measured by an odds ratio, or the negative of the survival probability in a hazard function) together with the p-value function. With the graphical approach, Rothman and his coauthors are able to show pictorially how the p–values vary with increasingly positive and increasingly negative large effects of flutamide on patient survival. And what they show is substantively significant:
Eisenberger’s new data only reinforce the findings from the earlier studies that flutamide provides a small clinical benefit. Adding the latest data makes the p value function narrower, which is to say that the overall estimate is now more precise, and points even more clearly to a benefit of about 12% in the odds of surviving for patients receiving flutamide.
Rothman and others conclude: “the real lesson” from the latest study is “that one should eschew statistical significance testing and focus on the quantitative measurement of effects.” That sounds right. Statistical significance is hurting people, indeed killing them. It is leaving their illnesses and a defective notion of significance “unexplained.”
Still, a recent correspondent points out that although the relative risk is high, the measured relative difference is small, adding only a month or so extra life. (Other things equal, perhaps even a little life is better than none.) If you want to draw a line in your science, in any case, draw a line of minimally important effect or minimally important magnitude of a regression coefficient. Keep your eyes on the size.
11 G-4 Visualize
And visualize. Model uncertainty is not the same thing as parameter uncertainty. From farming to pharmaceuticals, we want to know what the entire distribution looks like from the point of view of oomph and precision, magnitudes of relationships, and attendant uncertainty. Not just the point mean or median, with a superscript of asterisks declaring “significant” or “highly” so. Remember Stephen Jay Gould’s far-above-the-median experience with surviving stomach cancer, discussed in his essay “The Median Isn’t the Message” (Gould 1985). Gould’s doctor cited a median survival time from diagnosis of about 8 months; but the prolific scholar and writer looked at the graphs showing estimates. He noticed a thin but long right-hand tail, changed his lifestyle and eating habits, and lived and worked for another 22 years (rather more than 8 months)!
An illuminating study by Soyer and Hogarth (Citation2011) tested the predictive ability of more than 200 econometricians using linear models. Prediction was most accurate when the experts were only given a theoretical regression line and scatter plot of data. Take away the plots and their ability to relate model error to levels of the dependent variable fell dramatically. For novice and seasoned alike, the several books by Tufte on the art and science of visualization are invaluable.
The variance can vary, error bounds vary, degree and direction of uncertainty vary. Pictures help us to see by how much. Like Gosset, we should pay more attention to the actual distribution of our data, not only to rejections of the normal or exponential distribution (which few deign to discover) but also simulations or other confirmations of the actual distribution.
12 G-3 Consider Posteriors And Priors Too (“It Pays To Go Bayes”)
Be explicit about how prior information is or is not incorporated. Perhaps the most commonly used alternative to classical tand p is the Bayes factor (Carlin and Louis Citation2008; Press Citation2003). Gosset, I have mentioned, was a Bayesian who for reasons of efficiency, and in a world without a supercomputer, resorted frequently to frequentist methods (especially power: Student Citation1938). For discrete data and simple hypotheses, the Bayes factor represents the ratio between the probability assigned to the data under an alternative hypothesis and the null hypothesis (Johnson Citation2013). One big advantage of Bayesian analysis is that one can compute the probability of a hypothesis, given the evidence, whereas with the null hypothesis test of significance, measured by a p value, one can only speak to the probability of seeing data more extreme than have actually obtained, assuming the null hypothesis of “no difference” (or whatever) to be true. As the Bayesian Jeffreys noted (1961, p. 409):
Whether statisticians like it or not, their results are used to decide between hypotheses, and it is elementary that if p entails q, q does not necessarily entail p. We cannot get from “the data are unlikely given the hypothesis” to”the hypothesis is unlikely given the data” without some additional rule of thought. Those that reject inverse probability have to replace it by some circumlocution, which leaves it to the student to spot where the change of data has been slipped in [, in] the hope that it will not be noticed.
Jeffreys went on to explain that if one assigns prior odds between the alternative and null hypotheses, multiplication of the Bayes factor by these prior odds yields the posterior odds between the hypotheses. From the posterior odds between hypotheses, scientists can compute the posterior probability that a null hypothesis is true (or in any case useful or persuasive) relative to an explicit alternative. Classical tests of significance, measured by t and p, cannot.6 Johnson (Citation2013) observes that in certain hypothesis tests the alternative hypothesis can be specified so that an equivalence between Bayes factors and p-values can be established. Technically speaking, Johnson and others have shown, in one parameter exponential family models in which a point null hypothesis has been specified on the model parameter, specifying the size of the test is equivalent to specifying a rejection threshold for the Bayes factor, provided that it is further assumed that the alternative hypothesis is specified so as to maximize the power of the test. The correspondence between Bayes factors and p-values in this setting is just one example of the false demarcation line between objective and subjective.
When an alternative hypothesis exists—and that’s the usual situation of science: otherwise, why test?—Bayes factors can be easily reported. Bayes factors permit individual scientists and consumers to use prior information or the principle of insufficient reason together with new evidence to compute the posterior probability that a given hypothesis, H, is true (or to repeat, useful or persuasive) based on the prior probability that they assign to each hypothesis. After all—fortunately—we do not have to begin every new observation or experiment from tabula rasa; we know some stuff, but we want to know more stuff, however imperfectly. Bayes factors add that information into the calculation comparing the likelihood of alternative hypotheses. For example, Bayes factors provide a clear interpretation of the evidence contained in the data in favor of or against the null: a Bayes factor of 10 simply means that the data were 10 times more likely under the alternative hypothesis than they were under the null hypothesis. Better than mushy p’s.
13 G-2 Cooperate Up, Down, And Across (Networks And Value Chains)
Cooperation was a hallmark of Irish agricultural development (economic historians will think of Horace Plunkett, John Bennett, William Gosset and others), the growth of the Guinness brewery, and—in one very large positive externality—the development of modern statistics. Cooperation includes the human side, such as the academic Karl Pearson inviting the unknown brewer, Mr. Gosset, to London for sabbatical in 1906–1907 to work and study at University College London Biometrics Lab. Despite a strong-man reputation which Guinness has held in some quarters, for trying to monopolize, the legendary brewery cooperated up and down supply chains, and sometimes to a remarkable extent. The 40-something years of repeated experiments on barley, already mentioned, is just one example: Guinness subsidized one-half (50%) of barley and malt investment by the Irish Department of Agriculture. There is something to be learned from that: remember the yield and net profit figures shared by Gosset above. Guinness sending scientific brewers (Gosset was hardly the only one) on sabbatical for postgraduate studies in statistics and chemistry and genetics is another example. Sharing seeds and technical know-how with the Carlsberg Brewery, in Denmark, is another (and Carlsberg reciprocated generously by offering a stellar brewing barley called “Prentice” for Guinness to try: it turned out to be the mother of the greatest barley in early and midcentury Europe). One could write a book on the importance of cooperation to the history and development of science and statistics. And finally:
14 G-1 Answer The Brewer’s Original Question (“How Should You Set The Odds?”)
Finally, how should you set the odds? As a 28-year-old brewer and self-trained statistician told Karl Pearson way back in 1905: “the degree of certainty to be aimed at must depend on the pecuniary advantage to be gained by following the result of the experiment, compared with the increased cost of the new method, if any, and the cost of each experiment.” Add to Gosset’s pecuniary advantage “lives saved,” “jobs gained,” “racism abolished,” “health crisis averted,” and the like and we have the makings of a general approach.
Archival Sources
Acknowledgment
Sincere thanks to the editors and reviewers, together with Roosevelt colleagues Gary Langer and Justin Shea, for commenting on a previous version of this paper. At the Guinness Storehouse Museum (Diageo) I have enjoyed for many years the benefit of assistance from archivist Eibhlin Colgan. The seeds of the present paper were sown during a 2017 sabbatical visit at Trinity College Dublin (TRISS Research Institute) and the University of Oxford, New College. Many thanks to Ronan Lyons (Trinity College) and to Warden Miles Young (New College) for making those visits possible. Any errors are my own.
Notes
1 Harold Hotelling (Citation1930, p. 189), a vice president of the American Statistical Association and a teacher of many leading economists and statisticians, wrote: “I have heard guesses in this country, identifying ‘Student’ with Egon S. Pearson and the Prince of Wales.”
2 See also Student Citation1942; Pearson Citation1990; Ziliak Citation2014, Citation2010a, Citation2010b; Ziliak and Teather-Posadas Citation2016.
4 Deming (1978, p. 879). Deming said he learned the technique from Neyman (Citation1934). In the seminal article Neyman demonstrates the statistical and economic advantages of stratified sampling over random sampling (Neyman Citation1934, pp. 579-585). Neyman credits the idea of “purposive selection” to earlier writers, such as Bowley and Gini and Galvani.
5 Deming (1978, p. 880-881), Tippett (1958, p. 356). In a Riesling vine-and-wine experiment, Meyers, Sacks, van Es, and Vanden Heuvel (Citation2011) used blocking, balancing, and repetition (at n = 3 vineyards) to reduce sample size requirements by up to 60%.
6 Lavine and Schervich (1999) caution that Bayes factors can sometimes lead to incoherence in the technical statistical sense of that term.
References
- Banerjee, A., and Duflo, E. (2011), Poor Economics, New York: Public Affairs.
- Carlin, B., and Louis, T. (2008), Bayes and Empirical Bayes Methods for Empirical Analysis (3rd rev. ed.), London: Chapman and Hall/CRC Press.
- De Finetti, B. (1971 [1976]), Comments on Savage’s “On Rereading R. A. Fisher,” Annals of Statistics, 4, 486–487.
- Deming, W. E. (1938 [1943]), Statistical Adjustment of Data, New York: Dover.
- Deming, W. E. (1961), Sample Design in Business Research, New York: Wiley.
- Deming, W. E. (1982), Out of the Crisis, Cambridge, MA: MIT Center for Advanced Engineering Study.
- Eisenberger, M. A., et al. (1998), “Bilateral Orchiectomy with or Without Flutamide for Metastatic Protate Cancer,” New England Journal of Medicine, 339, 1036–1042. DOI: 10.1056/NEJM199810083391504.
- Fisher, R. A. (1925 [1928]), Statistical Methods for Research Workers, Edinburgh, UK: Oliver and Boyd.
- Fisher, R. A. (1933), “The Contributions of Rothamsted to the Development of the Science of Statistics,” Annual Report of Rothamsted Experimental Station, 43–50.
- Fisher, R. A. (1935), The Design of Experiments, Edinburgh, UK: Oliver & Boyd.
- Frank, R. (2017), Microeconomics and Behavior (9th ed.), New York: McGraw-Hill.
- Glewwe, P., Park, A., and Zhao, M. (2012), “Visualizing Development: Eyeglasses and Academic Performance in Rural Primary Schools in China,” Working Paper WP12-2, Center for International Food and Agricultural Policy, University of Minnesota.
- Goodman, S. (2017), “Why is Eliminating P-Values So Hard? Reflections on Science and Statistics,” ASA Symposium on Statistical Inference, Oct. 11–13, 2007.
- Goodman, S. (2002), “A Comment on Replication, P Values, and Evidence,” Statistics in Medicine, 11, 875–879.
- Gosset, W. S. (1904), “The Application of the ‘Law of Error’ to the Work of the Brewery,” Laboratory Report, 8, Arthur Guinness & Son, Ltd., Diageo, Guinness Archives, 3–16 and unnumbered appendix.
- Gosset, W. S. (1905), Letter from W.S. Gosset to K. Pearson, Guinness Archives, GDB/BRO/1102
- Gosset, W. S. (1936), “Co-Operation in Large-Scale Experiments,” Supplement to the Journal of the Royal Statistical Society, 3, 115–136.
- Gosset, W. S. (1962), Letters of William Sealy Gosset to R.A. Fisher. Vols. 1–5, Eckhart Library, University of Chicago. Private circulation.
- Greenland, S., et al. (2016), “Statistical Tests, P-values, Confidence Intervals, and Power: A Guide to Misinterpretations,” The American Statistician, 70, 1–12.
- Hotelling, H. (1930), “British Statistics and Statisticians Today,” Journal of the American Statistical Association, 25, 186–190. DOI: 10.1080/01621459.1930.10503118.
- Jeffreys, H. (1939 [1961]), Theory of Probability (3rd ed.), London: Oxford University Press.
- Johnson, V. (2013), “Revised Standards for Statistical Evidence,” PNAS, 110, 19313–19317. DOI: 10.1073/pnas.1313476110.
- Kahneman, D. (2011), Thinking Fast and Slow, New York: Farrar, Straus and Giroux.
- Lavine, M., and Schervish, M. (1999), “Bayes Factors: What They Are and What They Are Not,” The American Statistician, 53, 119–122
- Lew, M. (2012), “Bad Statistical Practice in Pharmacology (And Other Basic Biomedical Disciplines): You Probably Don’t Know P,” British Journal of Pharmacology, 166, 1559–1567. DOI: 10.1111/j.1476-5381.2012.01931.x.
- Lindley, D. (1991), Making Decisions, New York: Wiley.
- Manski, C. (2018), “Treatment Choice with Trial Data: Statistical Decision Theory should Supplant Hypothesis Testing,” The American Statistician, this issue, DOI: 10.1080/00031305.2018.1513377.
- McCloskey, D. N., and Ziliak, S. T. (1996), “The Standard Error of Regressions,” Journal of Economic Literature, 34, pp. 97–114.
- McCloskey, D. N., and Ziliak, S. T. (2010), Brief of Amici Curiae Statistics Experts Professors Deirdre N. McCloskey and Stephen T. Ziliak in Support of Respondents, Matrixx Initiatives Inc. et al. v. Siracusano et al. (vol. No. 09–1156, pp. 22). Washington, DC: Supreme Court of the United States. (Ed.) Edward Labaton et al. Counsel of Record.
- McCloskey, D. N., and Ziliak, S. T. (2009),” Signifying Nothing: Reply to Hoover and Siegler,” Journal of Economic Methodology, 15, 39–55 DOI: 10.1080/13501780801913413.
- Meyers, J., Sacks, G., van Es, H., and Vanden Heuvel, J. (2011) “Improving Vineyard Sampling Efficiency via Dynamic Spatially Explicit Optimisation,” Australian Journal of Grape and Wine Research, 17, 306–315. DOI: 10.1111/j.1755-0238.2011.00152.x.
- Neyman, J. (1934), “On the Two Different Aspects of the Representative Method: The Method of Stratified Sampling and the Method of Purposive Selection,” Journal of the Royal Statistical Society, 97, 558–625. DOI: 10.2307/2342192.
- Pearson, E. S. (1939), “’Student’ as Statistician,” Biometrika, 30, 210–250. DOI: 10.2307/2332648.
- Pearson, E. S. (1990), Student: A Statistical Biography of William Sealy Gosset, Eds. R. L. Plackett and G. A. Barnard. Oxford: Clarendon Press.
- Pierson, S., et al. (2017), “Recommendations to Funding Agencies for Supporting Reproducible Research,” American Statistical Association, available at https://www.amstat.org/ASA/News/ASA-Develops-Reproducible-Research-Recommendations.aspx
- Press, S. J. (2003), Subjective and Objective Bayesian Statistics, New York: Wiley.
- Press, S. J. (1972 [2005]), Applied Multivariate Analysis: Using Bayesian and Frequentist Methods of Inference (2nd ed.), Mineola, NY: Dover.
- Ramsey, F. P. (1926), “Truth and Probability,” reprinted in H. Kyburg and H. Smoker, eds., Studies in Subjective Probability (New York: R. E. Krieger, 1980), 25–52.
- Rothman, K. J., Johnson, E. S., and Sugano, D. S. (1999), “Is Flutamide Effective in Patients with Bilateral Orchiectomy?” Lancet, 353, 1184. DOI: 10.1016/S0140-6736(05)74403-2.
- Savage, L. (1954), The Foundations of Statistics, New York: Dover.
- Savage, L. (1971 [1976]), “On Re-Reading R. A. Fisher,” Annals of Statistics, 4, 441–500.
- Soyer, E., and Hogarth, R. (2011), “The Illusion of Predictability: How Regression Statistics Mislead Experts,” International Journal of Forecasting, 28, 695–711. DOI: 10.1016/j.ijforecast.2012.02.002.
- Student (1907), “On the Error of Counting with a Haemacytometer,” Biometrika, 5, 351–360.
- Student (1908a), “The Probable Error of a Mean,” Biometrika, VI, 1–24.
- Student (1908b), “The Probable Error of a Correlation Coefficient,” Biometrika, 2/3, 300–310.
- Student (1923), “On Testing Varieties of Cereals,” Biometrika, 15, 271–293.
- Student (1925), “New Tables for Testing the Significance of Observations,” Metron, V, 105–108.
- Student (1938), “Comparison between Balanced and Random Arrangements of Field Plots,” Biometrika, 29, 363–378.
- Student (1942), Student’s Collected Papers, eds. Pearson, E. S. and Wishart, J., London: Biometrika Office.
- Supreme Court of the United States (2011), “Matrixx Initiatives, Inc., et al., No. 09–1156, Petitioner v. James Siracusano et al.,” On Writ of Certiorari to the United States Court of Appeals for the Ninth Circuit, March 22nd, 25 pp., syllabus.
- Taleb, N. N. (2018), Skin in the Game: Hidden Asymmetries in Daily Life, New York: Random House.
- The Guinness Archives (Diageo), Guinness Storehouse Museum, Dublin; Special Collections Library, University College London; Cork County (Ireland) Archives; Museum of English Rural Life, National Library of Ireland; University of Oxford, Bodleian Library and New College Library; Winchester College (UK) Archives; and University of Chicago, Crerar Library, Eckhart Library, and Regenstein Library.
- Wasserstein, R., and Lazar, N., eds. (2016), “ASA Statement on Statistical Significance and P-Values”, The American Statistician, 70, 129–132. DOI: 10.1080/00031305.2016.1154108.
- Ziliak, S. (2008), “Guinnessometrics: The Economic Foundation of ‘Student’s’ t,” Journal of Economic Perspectives, 22, 199–216. DOI: 10.1257/jep.22.4.199.
- Ziliak, S. (2010a), “The Validus Medicus and a New Gold Standard,” The Lancet, 376, 324–325.
- Ziliak, S. (2010b), “Significant Errors—Reply to Stephen Senn,” The Lancet, 376, 1391.
- Ziliak, S. (2014), “Balanced versus Randomized Field Experiments in Economics: Why W.S. Gosset Matters,” Review of Behavioral Economics, 1, 167–208.
- Ziliak, S. (2016), “The Significance of the ASA Statement on Statistical Significance and P-Values,” The American Statistician, 70, 1–2.
- Ziliak, S., and McCloskey, D. (2008), The Cult of Statistical Significance: How the Standard Error Costs Us Jobs, Justice, and Lives, Ann Arbor, MI: University of Michigan Press.
- Ziliak, S., and Teather-Posadas, E. (2016), “The Unprincipled Randomization Principle in Economics and Medicine,” in Oxford Handbook of Professional Economic Ethics, eds. G. DeMartino and D. McCloskey, Oxford: Oxford University Press, 423–452.