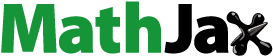
ABSTRACT
In response to growing concern about the reliability and reproducibility of published science, researchers have proposed adopting measures of “greater statistical stringency,” including suggestions to require larger sample sizes and to lower the highly criticized “p < 0.05” significance threshold. While pros and cons are vigorously debated, there has been little to no modeling of how adopting these measures might affect what type of science is published. In this article, we develop a novel optimality model that, given current incentives to publish, predicts a researcher’s most rational use of resources in terms of the number of studies to undertake, the statistical power to devote to each study, and the desirable prestudy odds to pursue. We then develop a methodology that allows one to estimate the reliability of published research by considering a distribution of preferred research strategies. Using this approach, we investigate the merits of adopting measures of “greater statistical stringency” with the goal of informing the ongoing debate.
1 Introduction
It is to be remarked that the theory here given rests on the supposition that the object of the investigation is the ascertainment of truth. When an investigation is made for the purpose of attaining personal distinction, the economics of the problem are entirely different. But that seems to be well enough understood by those engaged in that sort of investigation.
Note on the Theory of the Economy of Research,
Charles Sanders Peirce, 1879
In a highly cited essay, Ioannidis (Citation2005) uses the Bayes theorem to claim that more than half of published research findings are false. While not all agree with the extent of this conclusion (e.g., Goodman and Greenland Citation2007; Leek and Jager Citation2017), recent large-scale efforts to reproduce published results in a number of different fields (economics, Camerer et al. Citation2016; psychology, OpenScienceCollaboration Citation2015; oncology, Begley and Ellis Citation2012), have also raised concerns about the reliability and reproducibility of published science. Unreliable research not only reduces the credibility of science, but is also very costly (Freedman, Cockburn, and Simcoe Citation2015) and as such, addressing the underlying issues is of “vital importance” (Spiegelhalter Citation2017). Many researchers have recently proposed adopting measures of “greater statistical stringency,” including suggestions to require larger sample sizes and to lower the highly criticized “p < 0.05” significance threshold. In statistical terms, this represents selecting lower levels for acceptable Type I and Type II error rates. The main argument against “greater statistical stringency” is that these changes would increase the costs of a study and ultimately reduce the number of studies conducted.
Consider the debate about lowering the significance threshold in response to the work of Johnson (Citation2013), who, based on the correspondence between uniformly most powerful Bayesian tests and classical significance tests, recommends lowering significance thresholds by a factor of 10 (e.g., from p < 0.05 to p < 0.005). Gaudart et al. (Citation2014), voicing a common objection, contend that such a reduction in the allowable Type I error rate will result in inevitable increases to the Type II error rate. While larger sample sizes could compensate, this can be costly: “increasing the size of clinical trials will reduce their feasibility and increase their duration” (Gaudart et al. Citation2014). In the view of Johnson (Citation2014), this may not necessarily be such a bad thing, pointing to the excess of false positives (FPs) and the idea that (in the context of clinical trials) “too many ineffective drugs are subjected to phase III testing […] wast[ing] enormous human and financial resources.”
More recently, a highly publicized call by over seven dozen authors to “redefine statistical significance” has made a similar suggestion: lower the threshold of what is considered “significant” for claims of new discoveries from p < 0.05 to p < 0.005 (Benjamin et al. 2018). This has prompted a familiar response (e.g., Wei and Chen Citation2018). Amrhein, Korner-Nievergelt, and Roth (Citation2017) review the arguments for and against more stringent thresholds for significance and conclude that: “[v]ery possibly, more stringent thresholds would lead to even more results being left unpublished, enhancing publication bias. […] [W]hile aiming at making our published claims more reliable, requesting more stringent fixed thresholds would achieve quite the opposite.”
There is also substantial disagreement about suggestions to require larger sample sizes. In some fields, showing that a study has a sufficient sample size (i.e., high power) is common practice and an expected requirement for funding and/or publication, while in others it rarely occurs. For example, Charles et al. (Citation2009) found that 95% of randomized controlled trials (RCTs) report sample size calculations. In contrast, only a tiny fraction of articles in some fields –about 3% for psychology, and about 2% for toxicology—justify their sample size (Bosker, Mudge, and Munkittrick Citation2013; Fritz, Scherndl, and Kühberger Citation2013). The number is only marginally higher in conservation biology at approximately 8% (Fidler et al. Citation2006).
One argument is that, once a significant finding is achieved, the size of a study is no longer relevant. Aycaguer and Galbán (Citation2013) explain as follows: “If a study finds important information by blind luck instead of good planning, I still want to know the results.” Another viewpoint is that, while far from ideal, underpowered studies should be published since cumulatively, they can contribute to useful findings (Walker Citation1995). Others disagree and contend that small sample sizes undermine the reliability of published science (Button et al. Citation2013a; Dumas-Mallet et al. Citation2017; Nord et al. Citation2017). In the context of clinical trials, IntHout, Ioannidis, and Borm (Citation2016) review the many conflicting opinions about whether trials with suboptimal power are justified and conclude that, in circumstances when evidence for efficacy can be effectively combined across a series of trials (e.g., via meta-analysis), small sample sizes might be justified.
Despite the long-running and ongoing debates on significance thresholds and sample size requirements, there has been little to no modeling of how changes to a publication policy might affect what type of studies are pursued, the incentive structures driving research, and ultimately, the reliability of published science. One example is Borm, den Heijer, and Zielhuis (Citation2009) who conclude, based on simulation studies, that the consequences of publication bias do not warrant the exclusion of trials with low power. Another recent example is Higginson and Munafò (Citation2016), who, based on results from an optimality model of the “scientific ecosystem,” conclude that in order to “improve the scientific value of research” peer-reviewed publications should indeed require larger sample sizes, lower the α significance threshold, and give “less weight to strikingly novel findings.” Our work here aims to build upon these modeling efforts to better inform the ongoing discussion on the reproducibility of published science.
The model and methodology we present seeks to add three features absent from the model of Higginson and Munafò (Citation2016). While these authors consider how researchers balance available resources between exploratory and confirmatory studies, this simple dichotomy does not allow for a detailed assessment of the willingness of researchers to pursue high-risk studies (those studies that are, a priori, unlikely to result in a statistically significant finding). Our approach addresses this issue by considering a continuous spectrum of a priori risk, that is, the “pre-study probability.” Second, Higginson and Munafò (Citation2016) define the “total fitness of a researcher” (i.e., the payoff for a given research strategy) with diminishing returns for confirmatory studies, but not for exploratory studies. This choice, however well intended, has problematic repercussions for their optimality model. (Under their framework, the optimal research strategy will depend on T, an arbitrary total budget parameter.) Finally, by failing to incorporate the number of correct studies that go unpublished within their metric for the value of scientific research, many potential downsides of adopting measures to increase statistical stringency are ignored. Other differences between our approach and previous ones will be made evident and include: considering outcomes in terms of distributional differences, and specific modeling of how sample size requirements are implemented.
The remainder of this article is structured as follows. In Section 2, we describe the model and methodology proposed to evaluate different publication policies. We also list a number of metrics of interest and look to recent meta-research analyses to calibrate our model. In Section 3, we use the proposed methodology to evaluate the potential impact of lowering the significance threshold; and in Section 4, the impact of requiring larger sample sizes. Finally, in Section 5, we conclude with suggestions as to how publication policies can be defined to best improve the reliability of published research.
2 Methods
Recently, economic models have been rather useful for evaluating proposed research reforms (Gall, Ioannidis, and Maniadis Citation2017). However, modeling of how resources ought to be allocated among research projects is not new. See, for example, the work of Greenwald (Citation1975), Dasgupta and Maskin (Citation1987), McLaughlin (Citation2011), and Miller and Ulrich (Citation2016). As noted in the introduction, our framework for modeling the scientific ecosystem is closest in spirit to that of Higginson and Munafò (Citation2016) who formulate a relationship between a researcher’s strategy and their payoff, with the strategy involving a choice of mix between exploratory and confirmatory studies, and a choice of pursuing fewer studies with larger samples or more studies with smaller samples.
The publication process is complex and includes both objective and subjective considerations of efficacy and relevance. The title of this article was chosen specifically to emphasize this point (Gornitzki, Larsson, and Fadeel Citation2015). A large, complicated human process like that of scientific publication cannot be entirely reduced to metrics and numbers: there are often financial, political and even cultural reasons for an article being accepted or rejected for publication. With this in mind, the model presented here should not be seen as an attempt to precisely map out the peer-review process, but rather, as a useful tool for determining the consequences of implementing different publication policies.
Within our optimality model, many assumptions and simplifications are made. Most importantly, we assume that each researcher must make decisions consisting of only two choices: what statistical power (i.e., sample size) to adopt and what “prestudy probability” to pursue. Before elaborating further, let us briefly discuss these two concepts.
2.1 Statistical Power
Increasing statistical power by conducting studies with larger sample sizes would undeniably result in more published research being true. However, these improvements may only prove modest, given current publication guidelines. When we consider the perspectives of both researchers and journal editors, it is not surprising that statistical power has not improved substantially (Smaldino and McElreath Citation2016; Lamberink et al. Citation2018) despite being highlighted as an issue over five decades ago (Cohen Citation1962).
In practice, multiple factors can influence sample size determination (Lenth Citation2001; Hazra and Gogtay Citation2016; Roos Citation2017). Strictly in terms of publication prospects however, there is little incentive to conduct high-powered studies: basic logic suggests that the likelihood of publication is only minimally affected by power. To illustrate, consider a large number of hypotheses tested, out of which 10% are truly nonnull. Under the assumption that only (and all) positive results are published with (which may in fact be realistic in certain fields, Fanelli Citation2011), simple analytical calculation shows that increasing average power from an “unacceptably low” 55% to a “respectable” 85% (at the cost of more than doubling sample size), results in only a minimal increase in the likelihood of publication: from 10% to 13%. Moreover, the proportion of true findings amongst those published is only increased modestly: from 55% to 64%. Indeed, a main finding of Higginson and Munafò (Citation2016) is that the rational strategy of a researcher is to “carry out lots of underpowered small studies to maximize their number of publications, even though this means around half will be false positives.” This result is in line with the views of many; see, for example, Bakker, van Dijk, and Wicherts (Citation2012), Button et al. (Citation2013b), and Gervais et al. (Citation2015).
From a journal’s perspective, there is also little incentive to require larger sample sizes as a requirement for publication. There are minimal consequences from publishing false claims. Fraley and Vazire (Citation2014) review the publication history of six major journals in social-personality psychology and find that “journals that have the highest impact [factor] also tend to publish studies that have smaller samples.” This finding is in agreement with Szucs and Ioannidis (Citation2016) who conclude that, in the fields of cognitive neuroscience and psychology, journal impact factors are negatively correlated with statistical power; see also Brembs, Button, and Munafò (Citation2013).
2.2 Prestudy Probability
We use the term “prestudy probability” (psp) as shorthand for the a priori probability that a study’s null hypothesis is false. In this sense, highly exploratory research will typically have very low psp, whereas confirmatory studies will have a relatively high psp. Studies with low psp are not problematic per se. To the contrary, there are undeniable benefits to pursuing “long-shot” novel ideas that are very unlikely to work out, see Cohen (Citation2017). While replication studies (i.e., studies with higher psp) may be useful to a certain extent, there is little benefit in confirming a result that is already widely accepted. As Button et al. (Citation2013a) note: “As R [the pre-study odds] increases […] the incremental value of further research decreases.” Most scientific journals no doubt take this into account in deciding what to publish, with more surprising results more likely to be published. This state of affairs persists, despite recent calls for more replication studies (e.g., Moonesinghe, Khoury, and Janssens Citation2007). Indeed, replication studies are still often rejected on the grounds of “lack of novelty;” see Makel, Plucker, and Hegarty (Citation2012), Yeung (Citation2017), and Martin and Clarke (Citation2017). As such, researchers deciding which hypotheses to pursue toward publication will likely emphasize those with lower psp.
Recognize that the lower the psp, the less likely a “statistically significant” finding is to be true. As such, we are bound to a “seemingly inescapable trade-off” (Fiedler Citation2017) between the novel and the reliable. Journal editors face a difficult choice. Either publish studies that are surprising and exciting yet most likely false, or publish reliable studies which do little to advance our knowledge. Based on a belief that both ends of this spectrum are equally valuable, Higginson and Munafò (Citation2016) conclude that, in order to increase reliability, current incentive structures should be redesigned, “giving less weight to strikingly novel findings.” This is in agreement with the view of Hagen (Citation2016) who writes: “If we are truly concerned about scientific reproducibility, then we need to reexamine the current emphasis on novelty and its role in the scientific process.”
2.3 Model Framework
We describe our model framework in five simple steps.
We assume, for simplicity, that all studies test a null hypothesis of equal population means against a two-sided alternative, with a standard two-sample Student t-test. Each study has an equal number of observations per sample (
;
). Furthermore, let us assume that a study can have one of only two results: (1) positive (p-value
), or (2) negative (p-value
). Given the true effect size,
(the difference in population means),
, the common population variance, and the sample size, n, we can easily calculate the probability of a significant result, using the standard formula for power.1
Then, for a given true effect size of δ or zero, we have the probability of a TP, FN, FP, and TN, equal to:
, and
, respectively.
Next, we consider a large number of studies, nS, each with a total sample size of n. Of these nS studies, only a fraction, psp (where psp is the prestudy probability), have a true effect size of
. For the remaining
studies, we have
. Note that for a given sample size, n, these nS studies are each “powered” at level
. Throughout this article, we focus on scenarios, where
and
or
. These choices reference the analyses of Lamberink et al. (Citation2018) and Richard, Bond Jr, and Stokes-Zoota (2003). Lamberink et al. (Citation2018), based on an empirical analysis of over one hundred-thousand clinical trials conducted between 1975 and 2014, estimate that the median effect size of clinical trials is approximately a Cohen’s d = 0.20. In a similar analysis based on data from over 25,000 social/personality studies, Richard, Bond Jr, and Stokes-Zoota (2003) estimate that the mean effect size in social psychology research is of a Cohen’s d = 0.43.
We also label each study as either published (PUB) or unpublished (UN) for a total of 8 distinct categories (
2 (positive, negative) × 2 (true and false) × 2 (published and unpublished)). One can determine the expected number of studies (out of a total of nS studies) in each category by simple arithmetic. lists the equations for each of the eight categories with A equal to the probability of publication for a positive result, and B equal to the probability of publication for a negative result. Throughout this article, we will always assume that only positive studies are published, hence, we set B = 0. Initially, we will let the probability of publication of a positive study, A, depend on the psp according to the function
, where m
is a tuning parameter. This simple, decreasing function of psp represents a system in which positive results with lower psp are more likely to be published on the basis of novelty. A higher value of m indicates that higher psp studies (i.e., low-risk hypotheses) are less likely to be published. Consequently, a higher value of m implies a lower overall publication rate. In Section 4, we will define A differently, such that the probability of publication depends on the values of both psp and pwr.
Table 1 Equations for the expected number of studies (out of a total of nS studies) for each of the eight categories; with A
prob. of publication for a positive result and B
prob. of publication for a negative result. The number of studies nS, changes for different values of pwr. We have that:
, where n(pwr) is the required sample size to obtain a power of pwr.
We determine the total number of studies, nS, based on three parameters: T, the total resources available (in units of observations sampled); k, the fixed cost per study (also expressed in equivalent units of observations sampled); and n, the total sample size per study. Consequently, as in Higginson and Munafò (Citation2016),
. Then for any given level of power, pwr, we can easily obtain the necessary sample size per study, n, (using established sample size formula for two-sample t-tests, see Equationequations 1
(1)
(1) and Equation2
(2)
(2) ), and a resulting total number of studies, nS. Keep in mind that nS, the number of studies, is a function of pwr. Throughout this article, when necessary, we take T = 100,000. However, note that when comparing the outcomes of different publication policies, this choice is entirely irrelevant. Consider that when k is small, the cost of data, relative to the total cost of a study, is large. Conversely, when k is large, the relative cost of increasing the study sample size is small. For example, suppose k = 20 and
, then increasing the power from 0.55 to 0.85, implies doubling the total cost of the study. If instead k = 500 (all else being equal), this increase in power corresponds to only a 50% increase in the total study cost.
Finally, let us define a “research strategy” to be a given pair of values for (psp, pwr) within ([0,1] × [α,1]). Then, for a given research strategy we can easily calculate the total expected number of publications (ENP):
(3)
(3)
illustrates how is calculated for different values of psp and pwr, with fixed
, k = 100, and m = 3.
Fig. 1 The nine-gridded squares represent nine different research strategies; from left to right, we have , 0.5, and 0.8; from bottom to top, we have pwr
0.2, 0.5, and 0.8. For each pair of values for (psp, pwr), the different colored areas represent the probabilities of a TP, a FP, a TN, and a FN. The Expected Number of Publications (
) is calculated by multiplying (1) the probability of a positive finding (
), (2) the number of studies (
), and (3) the probability of publication (
). The figure corresponds to an ecosystem defined by
, m = 3, k = 100, B = 0,
, and T = 100,000.
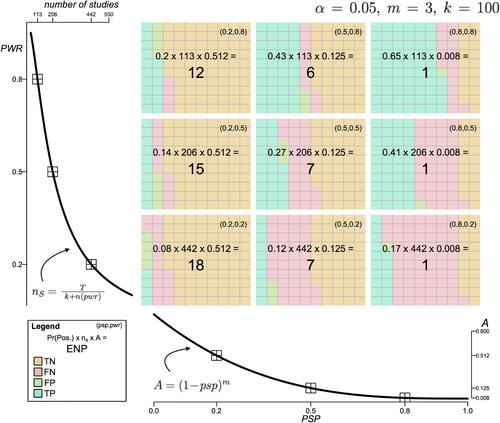
With this set-up in hand, suppose now a researcher pursues -consciously or unconsciously- strategies that maximize the expected number of publications (Charlton and Andras Citation2006). This may not be an entirely unreasonable assumption considering the influence of Goodhart’s Law (“Any observed statistical regularity will tend to collapse once pressure is placed upon it for control purposes”) on academia, see Fire and Guestrin (Citation2018) and van Dijk, Manor, and Carey (Citation2014). shows the analytical calculations of how changes over a range of values of psp and pwr and under different fixed values for k and m. (Note how the central panel of = 100, m = 3) corresponds to .) Depending on k and m, the value of (psp, pwr) that maximizes
can change substantially. With larger k, higher powered strategies will yield a greater
; with smaller m, optimal strategies are those with higher psp. It is interesting to observe how the optimal strategy changes under different scenarios. However, it may be more informative to consider a distribution of preferred strategies. This may also be a more realistic approach. While rational researchers may be drawn toward optimal strategies, surely scientists are not willing and/or able to precisely identify these.
Fig. 2 Plots show the number of expected publications, , for different values of
and
. The printed numbers correspond to values of ENP at
, 0.5, and 0.8; and
, 0.5, and 0.8. Each panel represents one ecosystem defined by
and B = 0, with k and m as indicated by column and row labels, respectively. Depending on k and m, the value of (
) that maximizes
can change substantially. With a higher k, higher powered strategies will yield a greater
; with smaller m, optimal strategies are those with higher
. Assuming that researchers behave based on optimizing the use of their resources, it is interesting to observe how the“optimal strategy” changes under different scenarios.
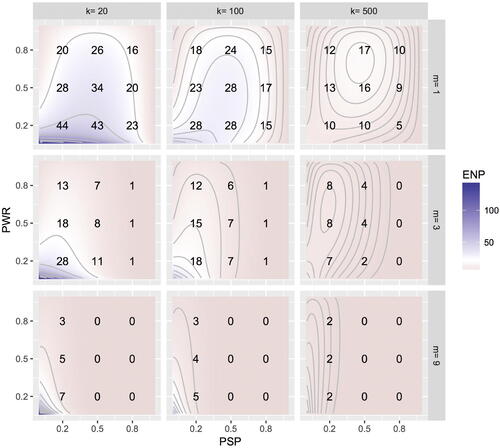
Taking the incentive to publish as a starting point, we assume scientists are more likely to spend resources on studies whose psp and pwr produce a higher expected number of publications. So we consider a distribution of resource allocation across study characteristics whose density is proportional to the expected number of publications, that is, . We emphasize that from a funder’s viewpoint,
describes where dollars are being spent on the (psp, pwr) plane. For example, consider the nine strategies illustrated in . Given the relative ENP values, we expect the expenditure on
studies to be 18 times greater than that on
studies.
In the appendix, we give expressions for two distributions that follow on from . The first is
which describes where attempted studies (not resources) fall on the (psp, pwr) plane, presuming that resources are allocated according to
. Since higher powered studies are most costly,
and
are not the same. Hence,
describes what kinds of studies are attempted more frequently.
Of course not all attempted studies are published. Thus, in the appendix we also give an expression for , the density of (psp, pwr) across published studies that is implied when
describes the characteristics of attempted studies. Note then that each of
, and
describes relatively favored and disfavored (psp, pwr) combinations. However, the distinction between the three is important. While
describes resource deployment,
describes the resulting constellation of attempted studies, and
describes the resulting constellation of published studies.
Armed with , and
, we can investigate the properties of a given scientific ecosystem, and how these properties vary across ecosystems. Specifically, an ecosystem is specified by choices of α, k, m, A, and B.
For any specification, properties of the three distributions are readily computed via two-dimensional numerical integration using a fine (200 by 200) grid of (psp, pwr) values. We do not require any simulation to obtain our results (except for those results relating to the accuracy of published research, see details in the appendix). To illustrate, consider the much coarser 3 by 3 grid plotted in and see how ENP could be easily calculated by summation across the two dimensions.
2.4 Ecosystem Metrics
We will evaluate the merits of different publication policies on the basis of the following six ecosystem metrics of interest. In the appendix, we provide further details on these metrics and introduce some compact notation that will be useful for their calculation from and its by-products.
The Publication Rate (PR). The ratio of the number of published studies over the number of attempted studies is of evident interest.
The Reliability (REL). The proportion of published findings that are correct is a highly relevant metric for the scientific ecosystem. Ideally, we wish to see a literature with as few FPs as possible.
The Breakthrough Discoveries (DSCV). The ability of the scientific ecosystem to produce breakthrough findings is an important attribute. Here, a true breakthrough result is defined as a TP and published study that results from a psp value below a threshold of 0.05. Note that we will calculate the total number of true breakthrough discoveries, DSCV, for each ecosystem for T units of resources. We will also note the reliability of breakthrough discoveries (
), the proportion of positive and published studies with psp < 0.05 that are true.
The Balance Between Exploration and Confirmation (
). The interquartile range of the distribution of psp values amongst published studies provides an assessment of the much discussed balance between exploratory and confirmatory research; see Sakaluk (Citation2016) and Kimmelman, Mogil, and Dirnagl (Citation2014).
The Median Power of Published Studies (
). As mentioned earlier, higher powered research leads to fewer yet more reliable publications.
The Accuracy of Published Research. We will report the mean absolute estimated effect size in published studies,
, as well as the proportion of published studies for which the estimated effect size is negative (
). It is well known that, due to a combination of publication bias and low powered studies, published effect sizes will be inflated, see Ioannidis (Citation2008); Lane and Dunlap (Citation1978). In addition, we will report the exaggeration ratio (the expected Type M error), and the Type S error rate (the proportion of published studies with an error in sign), see Gelman and Carlin (Citation2014).
2.5 Model Calibration
Let us begin by assessing whether or not our basic model framework is well calibrated. Are our modeling choices (specifically the choice of values to consider for parameters k and m) at all consistent with what is known about real conditions? In order to answer this important question, we considered a large number of different scenarios in which α is held fixed at 0.05, and both the fixed-cost parameter, k, and the novelty parameter, m, take various values; see .
Table 2 For ecosystems defined by fixed and B = 0, and varying values of k, m, and δ, the table lists estimates for the metrics of interest.
There is a recognized lack of empirical research measuring the relationship between experimental costs and sample sizes (Roos Citation2017), and it is even more difficult to empirically access appropriate values for m. However, we were able to choose suitable values for m (= 1, 3, and 9) and k (= 20, 100, and 500) based on how our model outputs compared with empirical estimates in the meta-research literature. Specifically, values were chosen to exhibit a sufficiently wide range and so that (1) the reliability, (2) the power, and (3) the publication rate agreed, at least to a certain extent, with what has been observed empirically. Consider the following.
Due to the success of several recent large-scale reproducibility projects, we are able to obtain reasonable approximations for the reliability of published research in different scientific fields. For example, Begley and Ioannidis (Citation2015) estimate that the reliability (i.e., TP rate) of social science experiments published in the journals Nature and Science is approximately 67%. Based on the large-scale reproducibility project of OpenScienceCollaboration (Citation2015), Wilson and Wixted (Citation2018) estimate that the reliability of published studies in well-respected social-psychology journals is approximately 49%, and in cognitive psychology journals, approximately 81%. For the chosen values of k and m, our model produces reliability measures ranging from 15% to 83%, for
, and from 25% to 86%, for
. Note that this wide range of values includes some reliability percentages that seem rather low (e.g., 15% and 25%).
There have also been a number of recent attempts to estimate the typical power of published research using meta-analytic effect sizes. For example, Button et al. (Citation2013b) estimate that the median statistical power in published neuroscience is approximately 21% and Dumas-Mallet et al. (Citation2017), using a similar methodology, conclude that the median statistical power in the biomedical sciences ranges from about 9% to 30% depending on the disease under study. Most recently, Lamberink et al. (Citation2018) analyzed the literature of RCTs and determined that the median power is approximately 9% overall (and about 20% in the subset of RCTs included in significant meta-analyses). For the chosen values of k and m, our model produces a simulated published literature with median power ranging from 9% to 60%, for
, and from 21% to 71%, for
.
While the publication rate numbers we obtain may appear rather low, ranging from 3% to 13%, consider that Siler, Lee, and Bero (Citation2015), in a systematic review of manuscripts submitted to three leading medical journals, observed a publication rate of 6.2%. In a review of top psychology journals, Lee and Schunn (Citation2011) found that acceptance rates ranged between 14% and 32%. It is important to recognize that these empirical estimates are calculated based on the subset of studies that are submitted for publication. If researchers are self-selecting their “most publishable” work for submission to journals, then these estimates will substantially overestimate the true publication rate of all studies. Song, Loke, and Hooper (Citation2014) estimate that about 85% of unpublished studies are unpublished for the simple reason that they are never submitted for publication. Our model also assumes that negative studies are never published (B = 0) and this (not entirely realistic) assumption also contributes to the low publication rate numbers we obtain. Senn (Citation2012) provides substantial insights on both these related issues: the level of publication bias, and how researchers may choose to submit research based on the perceived probability of acceptance. Note that while the scenarios with higher publication rates may appear more plausible, these scenarios have very (perhaps unreasonably) high values for reliability and median power.
Regardless of the values chosen for k and m, the overall trends for our metrics of interest will be similar. See . As k increases, the reliability of published research (REL) will increase, while the number of true breakthrough discoveries () decreases. As m increases, reliability (REL) decreases while the number of true breakthrough discoveries (
) increases. This strikes us as both reasonable and realistic. When a greater emphasis is placed on novelty (i.e., when m is larger), there will be a greater number of smaller, high-risk studies published. While these publications are less reliable, they are more numerous and more likely to produce a breakthrough finding. When data are more affordable relative to overall study cost (i.e., when k is larger), there will be fewer, larger studies and as a result the published literature will be more reliable but less likely to produce a breakthrough finding.
Before moving on, let us also briefly consider how the accuracy of published effect sizes changes with k and m, see . Overall, we see that the average of the absolute published effect size, , is always much larger than δ. Furthermore, we see that a large proportion of published effect sizes are negative. This is to be expected when psp values are small and many published studies are FPs. For the subset of studies that are positive, published, and true, we see that the Type M and the Type S errors are greatest when k is small, m is large, and δ is small. This is the same configuration that produces low-powered publications. Indeed, Type M and Type S errors are known to occur more frequently when power is low (Gelman and Carlin Citation2014).
Table 3 For ecosystems defined by fixed and B = 0, and varying values of k, m, and δ, the table lists estimates for the metrics of interest related to the accuracy of the published effects sizes.
3 The Effects of Adopting Lower Significance Thresholds
In this section, we investigate the impact of adopting lower significance thresholds. How might the published literature be different if the threshold was lowered? For positive studies, we will assume that the sample size of a study does not affect the likelihood of publication (at least not directly) and that studies with lower psp are more likely to be published according to the simple function introduced earlier,
. We compute the metrics of interest for 54 different ecosystems. Each ecosystem is uniquely defined with either
or
, with one of three possible values for m (
1, 3, 9), with one of three possible values for k (
20, 100, 500), and most importantly, with one of three possible values for the α significance threshold (
0.005, 0.020, 0.050).
3.1 Results
In , we note how the various metrics change with relative to
, by reporting ratios. While we focus our discussion specifically on scenarios for which
, the results for
are all very similar. Figures in the supplemental material plot the complete results. Based on our results, we can make the following conclusions on the impact of adopting a lower, more stringent, significance threshold.
Table 4 A comparison between ecosystems with and ecosystems with
.
Reliability is substantially increased with a lower threshold. Based on our results, comparing
to
, the impact on REL is greatest when δ is small, k is small, and m is large, see . This is due to the fact that with a lower significance threshold policy, attempted studies are typically of higher power (particularly so when k is small) and of higher prestudy probability. To understand why there would be more studies with higher power and higher prestudy probabilities, consider that with a lower α threshold, the probability of obtaining a significant result (i.e., p-value
) “by chance” is substantially reduced. To maximize one’s expected number of publications (ENP) when
, it is a much better strategy to pursue higher pwr and higher psp strategies. This is particularly true when the effect size, δ, is small.
A disadvantage of the lower threshold is that the number of breakthrough discoveries is substantially lower, see and Figure S3. This is due to the fact that with a lower α threshold, fewer “high-risk” (i.e., low psp) studies are attempted. The chance that the p-value will fall below α, when α is lowered to 0.005, is already risky enough. The
numbers we obtain suggest that, while fewer low psp studies will be published, these will be much more reliable.
When increasing study power is less costly relative to the total cost of a study (i.e., when k is larger, or when δ is larger), the benefit of lowering the significance threshold (increased
) is somewhat smaller. However, the downside (decreased
) is substantially smaller, see left-panels of Figures S1 and S3. This suggests that a policy of lowering the significance threshold would perhaps be best suited in a field of research in which increasing one’s sample size is less burdensome. This nuance recalls the suggestion of Ioannidis, Hozo, and Djulbegovic (Citation2013): “Instead of trying to fit all studies to traditionally acceptable Type I and Type II errors, it may be preferable for investigators to select Type I and Type II error pairs that are optimal for the truly important outcomes and for clinically or biologically meaningful effect sizes.”
When novelty is more of a requirement for publication (i.e., when m is larger), the benefit of lowering the significance threshold is larger and the downside smaller. This result is due to the fact that a smaller α will incentivize researchers to allocate resources in the direction toward either higher powered or higher psp studies (i.e., away from the South-West corner of the plots in ). There is a choice between moving toward higher pwr (North) or toward higher psp (East), and different costs associated with each direction. This suggests that for a lower significance threshold policy to be most effective, editors should also adopt, in conjunction, stricter requirements for research novelty. To illustrate, consider three ecosystems of potential interest with their estimated
, and PR metrics (all with
):
The baseline defined by
, m = 3, and k = 500 with:
, and PR = 0.054;
the alternative defined by
, m = 3, and k = 500 with:
, and PR = 0.040; and
the suggested defined by
, m = 9, and k = 500 with:
, and PR = 0.015.
Note that while the suggested has high REL and relatively high DSCV, the PR is substantially reduced.
As mentioned earlier, the balance between exploratory and confirmatory research is an important aspect of a scientific ecosystem. The results show that the interquartile range for psp does not change substantially with α. As such, we could conclude that even with a much lower significance threshold, there will still be a wide range of studies attempted in terms of their psp. However, psp values do tend to be substantially higher with smaller α. As such, we should expect that, with smaller α, research will move toward more confirmatory, and less exploratory studies.
With a lower α significance threshold, the published effect sizes are much more accurate. When
, the Type M error is reduced to at most 1.4 (i.e., effect size estimates are inflated by at most 40%) and Type S error is negligible; see , S7 and S8. These reductions are greatest when data are relatively expensive and when the true effect size is small (i.e. when k = 20 and
).
4 The Effects of Strict a priori Power Calculation Requirements
In this section, we investigate the effects of requiring “sufficient” sample sizes. In practical terms, this means adopting publication policies that require studies to show a priori power calculations indicating that sample sizes are “sufficiently large” to achieve the desired level of statistical power, typically 80%. Whereas before, the chance of publishing a positive study in our framework depended only on psp, we now set the probability of publication, A, to also depend on power. In doing so however, we wish to acknowledge the fact that a priori sample size claims are often “wildly optimistic” (Bland Citation2009).
The “sample size samba” (Schulz and Grimes Citation2005)—the practice of “tweaking aspects of sample size calculation” (Hazra and Gogtay Citation2016) in order to obtain what is affordable—often results in a study with less than 80% power being advertised as having 80% power. Even in ideal circumstances, power can be exaggerated due to “optimism bias” (Djulbegovic et al. Citation2011), also known as the “illusion of power” (Vasishth and Gelman Citation2017), which occurs when the anticipated effect size is based on a literature filled with overestimates due to publication bias.
To take into account the problematic nature of a priori power calculations, our model is defined such that possessing only 50% power substantially reduces, but does not eliminate, the chance of publication. For studies which really do have 80% power and higher, there will be no notable reduction in the probability of publication. See the appendix for details on how we define A as a continuous function of both psp and pwr.
We calculated the metrics of interest for the same 54 different ecosystems as in Section 3, with our new definition of A. To contrast these ecosystems with those discussed in the previous section, we refer to these ecosystems as “with SSR” (sample size requirements).
4.1 Results
See . Based on our results, we can make the following main conclusions on the measurable consequences of adopting a journal policy requiring an a priori sample size justification.
Table 5 A comparison between ecosystems with a sample size requirement and ecosystems without.
With SSR, we observed much higher powered studies, see Figure S5. Reliability is also increased, particularly when novelty is highly prized (m is large) and effect sizes are small. This result is as expected. As soon as having a small sample size jeopardizes the probability of publication, it is in the researcher’s best interest to conduct higher powered studies.
Requiring “sufficient sample sizes” for publication can be quite detrimental in terms of the number of breakthrough discoveries. The impact on
is greatest when δ, k, and m are all small; see . The
numbers show that reliability is particularly increased for low psp publications.
In conjunction with requiring larger sample sizes, it may be wise to place greater emphasis on research novelty. As in Section 3, such a combined approach could see an increase in reliability with only a limited decrease in discovery. This trade-off is most beneficial when k is small. Consider three ecosystems of potential interest (all with
, and
) with their estimated REL, DSCV, and PR metrics:
The baseline defined by m = 3, k = 100, and without SSR; with REL = 0.524, DSCV = 0.216, and PR = 0.043;
the alternative defined by m = 3, k = 100 and with SSR; with REL = 0.757, DSCV = 0.122, and PR = 0.044; and
the suggested defined by m = 6, k = 100 and with SSR; with REL = 0.502, DSCV = 0.325, and PR = 0.022.
Note that the while the suggested has both higher REL and higher
than the baseline, the PR is reduced.
With SSR, the Type M error is at most 1.2 (i.e., effect size estimates are inflated by at most 20%) and Type S error is negligible; see , S7 and S8.
4.2 In Tandem: The Effects of Adopting Both a Lower Significance Threshold and a Power Requirement
We are also curious as to whether lowering the significance threshold in addition to requiring larger sample sizes would carry any additional benefits relative to each policy innovation on its own. See Table A.1 for results, and consider two main findings:
For ecosystems with SSR, the distribution of published studies does not change dramatically when α is lowered. shows the density describing the characteristics of published studies,
, for the four policies of interest, with k = 500 and m = 3: (1)
, without SSR; (2)
, with SSR; (3)
, without SSR; and (4)
, with SSR. The difference between the densities in (2) and (4) is primarily a matter of a shift in psp.
As expected, lowering the significance threshold further increases reliability and the number of breakthrough discoveries is further decreased; see Table A.1, and Figures S1 and S3.
Fig. 3 Heat-maps show the density of published articles, , for the four policies of interest, with fixed
, k = 500 and m = 3.
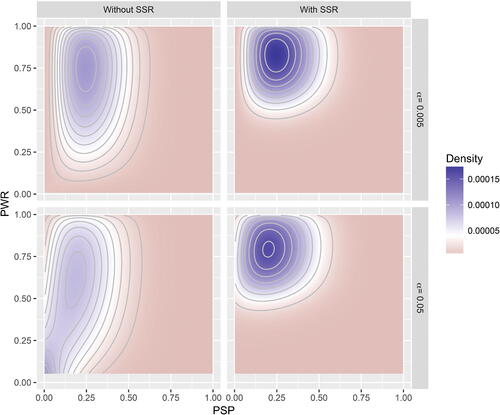
Fig. 4 Values of for varying values of k, m and α; results for
and
on the left and right panels, respectively. Top panels shows results with no power requirement (i.e.,
). Bottom panels shows results with power requirement (i.e., A defined as per Equationequation A4
(A4)
(A4) (with
and
)).
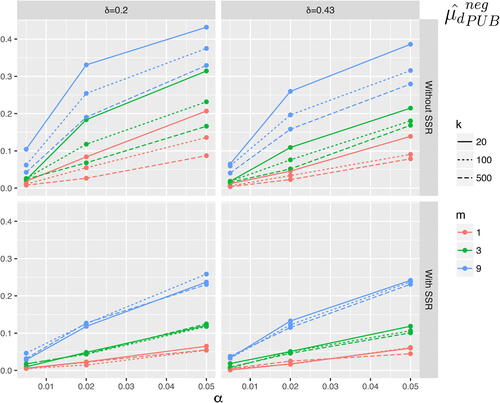
Fig. 5 Values of for varying values of k, m and α; results for
and
on the left and right panels respectively. Top panels shows results with no power requirement (i.e.,
). Bottom panels shows results with power requirement (i.e., A defined as per Equationequation A4
(A4)
(A4) (with
and
)).
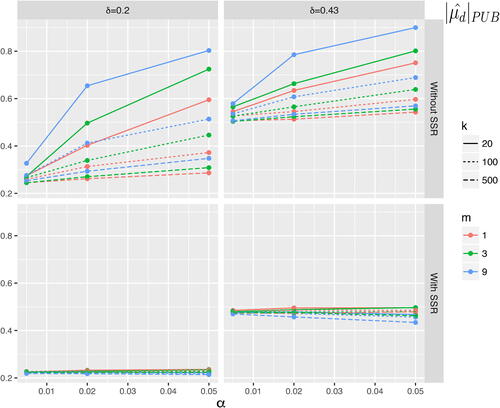
5 Conclusion
There remains substantial disagreement on the merits of requiring greater statistical stringency to address the reproducibility crisis. Yet all should agree that innovative publication policies can be part of the solution. Going forward, it is important to recognize that current norms for Type 1 and Type 2 error levels have been driven (almost) entirely by tradition and inertia rather than careful coherent planning and result-driven decisions (Hubbard and Bayarri Citation2003). Hence, improvements should be possible.
In response to Amrhein, Korner-Nievergelt, and Roth (Citation2017) who suggest that a more stringent α threshold will lead to published science being less reliable, our results suggest otherwise. However, just as Amrhein, Korner-Nievergelt, and Roth (Citation2017) contend, our results indicate that the publication rate will end up being substantially lower with a smaller α. While going from p < 0.05 to p < 0.005 may be beneficial to published science in terms of reliability, we caution that there may be a large cost in terms of fewer true breakthrough discoveries. One must ask whether a large reduction in novel discoveries is an acceptable price to pay for a large increase in their reliability? Importantly, and somewhat unexpectedly, our results suggest that this can be mitigated (to some degree) by adopting a greater emphasis on research novelty. In practice, implementing stricter requirements for research novelty, could be accomplished by greater editorial emphasis on “surprising results” as a requisite for publication. This approach, however, might be difficult to achieve unless one is willing to accept a much lower publication rate. In summary, publishing less may be the necessary price to pay for obtaining more reliable science.
Recently, some have suggested that researchers choose (and justify) an “optimal” value for α, for each unique study; see Mudge et al. (Citation2012), Ioannidis, Hozo, and Djulbegovic (Citation2013), and Lakens et al. (Citation2018). Each study within a journal would thereby have a different set of criteria. This is a most interesting idea and there are persuasive arguments in favor of such an approach. Still, it is difficult to anticipate how such a policy would play out in practice and how the research incentive structure would change in response. A model, like the one presented here, but with the α threshold set to vary with psp, could provide useful insight.
We are also cautious about greater sample size requirements. In summary, we found that the impacts of adopting a sample size requirement policy are similar to the impacts of lowering the α significance threshold. While improving reliability, requiring studies to show “sufficient power” will severely limit novel discoveries in fields where acquiring data is expensive. Is it beneficial for editors and reviewers to consider whether a study has “sufficient power”? How much should these criteria influence publication decisions? Answers to these questions are not at all obvious. Again, using the methodology introduced, we suggest that adopting a greater emphasis on research novelty may mitigate, to a certain extent, some of the downside of adopting greater sample size requirements at the cost of lowering the overall number of published studies. Given that, as a result of publication bias, it can often be better to discard 90% of published results for meta-analytical purposes (Stanley, Jarrell, and Doucouliagos Citation2010), this may be an approach worth considering.
Our main recommendation is that, before adopting any (radical) policy changes, we should take a moment to carefully consider, and model how, these proposed changes might impact outcomes. The methodology we present here can be easily extended to do just this. Two scenarios of interest come immediately to mind.
First, it would be interesting to explore the impact of publication bias, i.e., the tendency of journals to reject non-significant results (Sterling, Rosenbaum, and Weinkam Citation1995). This could be done by allowing B to take different non-zero values. Based on simulation studies, de Winter and Happee (Citation2013) suggest that publication bias can in fact be beneficial for the reliability of science. However, under slightly different assumptions, van Assen et al. (Citation2014) arrive at a very different conclusion. Clearly, a better understanding of how publication bias changes a scientist’s incentives is needed. If statistical significance becomes a much less important screening criterion for publication, how will published science change? Recently, a number of influential researchers have argued that to address low reliability, reviewers should “abandon statistical significance” (McShane et al. Citation2017). While our framework could be extended to explore this proposal (e.g., by taking A = B), these researchers suggest substituting statistical significance with a “more holistic view of the evidence” to inform publication decisions. Clearly, this does not lend itself to our modeling framework, or similar meta-research paradigms.
Second, it would be worthwhile to investigate the potential impact of requiring study preregistration. Coffman and Niederle (Citation2015) use the accounting of Ioannidis (Citation2005) to evaluate the effect of preregistration on reliability and conclude that pre-registration will have only a modest impact. However, the impact on the publication rate and on the number of breakthrough findings is still not well understood. This is particularly relevant given the current trend to adopt “result-blind peer-review” (Greve, Bröder, and Erdfelder Citation2013) policies including, most recently, the policy of Registered Reports (Chambers et al. Citation2015). The conditional equivalence testing publication policy proposed in our earlier work, see Campbell and Gustafson (Citation2018), could also be considered.
Our methodology assumes above all that researchers’ decisions are driven exclusively by the desire to publish. But the situation is more complex. Publication is not necessarily the end goal for a scientific study and requirements with regards to significance and power are not only encountered at the publication stage. In the planning stages, before a study even begins, ethics committees and granting agencies will often have certain minimal requirements; see Ploutz-Snyder, Fiedler, and Feiveson (Citation2014) and Halpern, Karlawish, and Berlin (Citation2002). And after a study is published, regulatory bodies and policy makers will also often subject the results to a different set of norms.
We also assumed a framework of independent Bernoulli trials. Of course, in practice, most studies are not conducted independently. For example, in the context of clinical trials, phase 3 studies are conducted based on the success or failure of earlier phase 2 studies (see Burt et al. (Citation2017) who model the advantages and disadvantages of different clinical development strategies). It is difficult to anticipate the consequences of failing to incorporate such dependencies in our model. A more elaborate model, in which studies are correlated and the psp of subsequent studies is updated appropriately, could prove very informative.
Finally, it is important to acknowledge that no publication policy will be perfect. Science is inherently challenging and we must always be willing to accept that a certain proportion of research is potentially false (Contopoulos-Ioannidis, Ntzani, and Ioannidis Citation2003; Djulbegovic and Hozo Citation2007). Each policy will have its advantages and disadvantages. Our modeling exercise makes this all the more evident and forces us to carefully consider different potential trade-offs.
Code
Please note that all code to produce the results, tables, and figures in this article have been posted to a repository on the Open Science Framework, DOI 10.17605/OSF.IO/YQCVA.
Note
where is the upper 100
-th percentile of the t-distribution with n – 2 degrees of freedom,
, and
is the cdf of the noncentral t distribution with df degrees of freedom and noncentrality parameter ncp. We calculate the minimum required sample size, n, to obtain a desired power, pwr, as follows:
(2)
(2)
Supplemental Material
Download PDF (126.7 KB)Acknowledgments
We wish to gratefully acknowledge Prof. Will Welch and Prof. John Petkau for their valuable suggestions and advice. Furthermore, we wish to acknowledge funding from the Natural Sciences and Engineering Research Council of Canada (NSERC grant number RGPIN 183772-13).
References
- Amrhein, V., Korner-Nievergelt, F., and Roth, T. (2017), “The Earth is Flat (p < 0.05): Significance Thresholds and the Crisis of Unreplicable Research,” Technical report, PeerJ Preprints.
- Aycaguer, L. C. S., and Galbán, P. A. (2013), Explicación Del Tamaño Muestral Empleado: Una Exigencia Irracional De Las Revistas Biomédicas. Gaceta Sanitaria 27, 53–57.
- Bakker, M., van Dijk, A., and Wicherts, J. M. (2012), “The Rules of the Game Called Psychological Science,” Perspectives on Psychological Science, 7, 543–554. DOI: 10.1177/1745691612459060.
- Begley, C. G., and Ellis, L. M. (2012), “Drug Development: Raise Standards for Preclinical Cancer Research,” Nature, 483, 531–533. DOI: 10.1038/483531a.
- Begley, C. G., and Ioannidis, J. P. (2015), “Reproducibility in Science: Improving the Standard for Basic and Preclinical Research,” Circulation Research, 116, 116–126. DOI: 10.1161/CIRCRESAHA.114.303819.
- Benjamin, D. J., Berger, J. O., Johannesson, M., Nosek, B. A., Wagenmakers, E.-J., Berk, R., Bollen, K. A., Brembs, B., Brown, L., Camerer, C., Cesarini, D., Chambers, C. D., Clyde, M., Cook, T. D., De Boeck, P., Dienes, Z., Dreber, A., Easwaran, K., Efferson, C., Fehr, E., Fidler, D., Field, A. P., Forster, M., George, E. I., Gonzalez, R., Goodman, S., Green, E., Green, D. P., Greenwald, A. G., Hadfield, J. D.,. Hedges, L. V., Held, L., Hua Ho, T., Hoijtink, H., Hruschka, D. J., Imai, K., Imbens, G., Ioannidis, J. P. A., Jeon, M., Holland Jones, J., Kirchler, M., Laibson, D., List, J., Little, R., Lupia, A., Machery, E., Maxwell, S. E., McCarthy, M., Moore, D. A.,. Morgan, S. L., Munafó, M., Nakagawa, S., Nyhan, B., Parker, T. H., Pericchi, L., Perugini, M., Rouder, J., Rousseau, J., Savalei, V., Schönbrodt, F. D., Sellke, T., Sinclair, B., Tingley, D., Van Zandt, T., Vazire, S., Watts, D. J., Winship, C., Wolpert, R. L., Xie, Y. Young, C., Zinman, J. and Johnson V. E. (2018), “Redefine Statistical Significance,” Nature Human Behaviour, 2, 6–10. DOI: 10.1038/s41562-017-0189-z.
- Bland, J. M. (2009), “The Tyranny of Power: Is There a Better Way to Calculate Sample Size? BMJ, 339, b3985. DOI: 10.1136/bmj.b3985.
- Borm, G. F., den Heijer, M., and Zielhuis, G. A. (2009), “Publication Bias Was Not a Good Reason to Discourage Trials With Low Power,” Journal of Clinical Epidemiology, 62, 47–53. DOI: 10.1016/j.jclinepi.2008.02.017.
- Bosker, T., Mudge, J. F., and Munkittrick, K. R. (2013), “Statistical Reporting Deficiencies in Environmental Toxicology,” Environmental Toxicology and Chemistry, 32, 1737–1739. DOI: 10.1002/etc.2226.
- Brembs, B., Button, K., and Munafò, M. (2013), “Deep Impact: Unintended Consequences of Journal Rank,” Frontiers in Human Neuroscience, 7, 1–12.
- Burt, T., Button, K., Thom, H., Noveck, R., and Munafò, M. R. (2017), “The Burden of the “False-Negatives” in Clinical Development: Analyses of Current and Alternative Scenarios and Corrective Measures,” Clinical and Translational Science, 10, 470–479. DOI: 10.1111/cts.12478.
- Button, K. S., Ioannidis, J. P., Mokrysz, C., Nosek, B. A., Flint, J., Robinson, E. S., and Munafò, M. R. (2013a). “Empirical Evidence for Low Reproducibility Indicates Low Pre-study Odds,” Nature Reviews Neuroscience 14, 877–877. DOI: 10.1038/nrn3475-c6.
- Button, K. S., Ioannidis, J. P., Mokrysz, C., Nosek, B. A., Flint, J., Robinson, E. S., and Munafò, M. R.(2013b), Power Failure: Why Small Sample Size Undermines the Reliability of Neuroscience,” Nature Reviews Neuroscience 14 (5), 365–376. DOI: 10.1038/nrn3475.
- Camerer, C. F., Dreber, A., Forsell, E., Ho, T.-H., Huber, J., Johannesson, M., Kirchler, M., Almenberg, J., Altmejd, A., Chan, T., et al. (2016), “Evaluating Replicability of Laboratory Experiments in Economics,” Science, 351, 1433–1436. DOI: 10.1126/science.aaf0918.
- Campbell, H., and Gustafson, P. (2018), “Conditional Equivalence Testing: An Alternative Remedy for Publication Bias,” PloS One 13, e0195145. DOI: 10.1371/journal.pone.0195145.
- Chambers, C. D., Dienes, Z., McIntosh, R. D., Rotshtein, P., and Willmes, K. (2015), “Registered Reports: Realigning Incentives in Scientific Publishing,” Cortex, 66, A1–A2. DOI: 10.1016/j.cortex.2015.03.022.
- Charles, P., B. Giraudeau, A. Dechartres, G. Baron, and P. Ravaud (2009). Reporting of sample size calculation in randomised controlled trials. BMJ 338, b1732. DOI: 10.1136/bmj.b1732.
- Charlton, B. G., and Andras, P. (2006). “How Should We Rate Research?: Counting Number of Publications May Be Best Research Performance Measure,” BMJ 332, 1214–1215. DOI: 10.1136/bmj.332.7551.1214-c.
- Coffman, L. C., and Niederle, M. (2015), “Pre-analysis Plans Have Limited Upside, Especially Where Replications Are Feasible,” Journal of Economic Perspectives, 29, 81–98. DOI: 10.1257/jep.29.3.81.
- Cohen (1962), “The Statistical Power of Abnormal-Social Psychological Research: A Review,” The Journal of Abnormal and Social Psychology, 65, 145–153. DOI: 10.1037/h0045186.
- Cohen, B. A. (2017), “How Should Novelty be Valued in Science?” eLife 6:e28699.
- Contopoulos-Ioannidis, D. G., Ntzani, E., and Ioannidis, J. (2003), “Translation of Highly Promising Basic Science Research Into Clinical Applications,” The American Journal of Medicine, 114, 477–484. DOI: 10.1016/S0002-9343(03)00013-5.
- Dasgupta, P., and Maskin, E. (1987), “The Simple Economics of Research Portfolios,” The Economic Journal, 97, 581–595. DOI: 10.2307/2232925.
- de Winter, J., and Happee, R. (2013), “Why Selective Publication of Statistically Significant Results Can Be Effective,” PLoS One, 8, e66463.
- Djulbegovic, B., and Hozo, I. (2007), “When Should Potentially False Re-search Findings Be Considered Acceptable?” PLoS Medcine, 4, e26. DOI: 10.1371/journal.pmed.0040026.
- Djulbegovic, B., Kumar, A., Magazin, A., Schroen, A. T., Soares, H., Hozo, I., Clarke, M., Sargent, D., and Schell, M. J. (2011), “Optimism Bias Leads to Inconclusive Results—An Empirical Study,” Journal of Clinical Epidemiology 64, 583–593. DOI: 10.1016/j.jclinepi.2010.09.007.
- Dumas-Mallet, E., Button, K. S., Boraud, T., Gonon, F., and Munafò, M. R. (2017), “Low Statistical Power in Biomedical Science: A Review of Three Human Research Domains,” Royal Society Open Science, 4, 160254. DOI: 10.1098/rsos.160254.
- Fanelli, D. (2011), “Negative Results Are Disappearing From Most Disciplines and Countries,” Scientometrics, 90, 891–904. DOI: 10.1007/s11192-011-0494-7.
- Fidler, F., Burgman, M. A., Cumming, G., Buttrose, R., and Thomason, N. (2006), “Impact of Criticism of Null-hypothesis Significance Testing on Statistical Reporting Practices in Conservation Biology,” Conservation Biology, 20, 1539–1544. DOI: 10.1111/j.1523-1739.2006.00525.x.
- Fiedler, K. (2017). What Constitutes Strong Psychological Science? The (neglected) Role of Diagnosticity and a Priori Theorizing,” Perspectives on Psychological Science, 12, 46–61. DOI: 10.1177/1745691616654458.
- Fire, M., and Guestrin, C. (2018), “Over-optimization of Academic Publishing Metrics: Observing Goodhart’s Law in Action,” arXiv preprint arXiv:1809.07841.
- Fraley, R. C., and Vazire, S. (2014), “The n-pact Factor: Evaluating the Quality of Empirical Journals With Respect to Sample Size and Statistical Power,” PloS One 9, e109019. DOI: 10.1371/journal.pone.0109019.
- Freedman, L. P., Cockburn, I. M., and Simcoe, T. S. (2015), “The economics of reproducibility in preclinical research. PLoS Biology, 13, e1002165. DOI: 10.1371/journal.pbio.1002165.
- Fritz, A., Scherndl, T., and Kühberger, A. (2013), “A Comprehensive Review of Reporting Practices in Psychological Journals: Are Effect Sizes Really Enough?” Theory & Psychology, 23, 98–122. DOI: 10.1177/0959354312436870.
- Gall, T., Ioannidis, J., and Maniadis, Z. (2017), “The Credibility Crisis in Research: Can Economics Tools Help?” PLoS Biology, 15, e2001846. DOI: 10.1371/journal.pbio.2001846.
- Gaudart, J., Huiart, L., Milligan, P. J., Thiebaut, R., and Giorgi, R. (2014), “Reproducibility Issues in Science, is p value Really the Only Answer?” Proceedings of the National Academy of Sciences of the United States of America 111, E1934. DOI: 10.1073/pnas.1323051111.
- Gelman, A., and Carlin, J. (2014), “Beyond Power Calculations Assessing Type s (sign) and Type m (magnitude) Errors,” Perspectives on Psychological Science, 9, 641–651. DOI: 10.1177/1745691614551642.
- Gervais, W. M., Jewell, J. A., Najle, M. B., and Ng, B. K. (2015), “A Powerful Nudge? Presenting Calculable Consequences of Underpowered Research Shifts Incentives Toward Adequately Powered Designs,” Social Psychological and Personality Science, 6, 847–854. DOI: 10.1177/1948550615584199.
- Goodman, S., and Greenland, S. (2007), “Assessing the Unreliability of the Medical Literature: A Response to ‘Why Most Published Research Findings Are False?”’ Johns Hopkins University, Department of Biostatistics; Working Papers.
- Gornitzki, C., Larsson, A., and Fadeel, B. (2015), “Freewheelin’ Scientists: Citing Bob Dylan in the Biomedical Literature,” BMJ 351:h6505. DOI: 10.1136/bmj.h6505.
- Greenwald, A. G. (1975), “Consequences of Prejudice Against the Null Hypothesis,” Psychological Bulletin, 82, 1–20. DOI: 10.1037/h0076157.
- Greve, W., Bröder, A., and Erdfelder, E. (2013), “Result-blind Peer Reviews and Editorial Decisions: A Missing Pillar of Scientific Culture,” European Psychologist, 18, 286–294. DOI: 10.1027/1016-9040/a000144.
- Hagen, K. (2016), “Novel or Reproducible: That is the Question,” Glycobiology, 26, 429–429.
- Halpern, S. D., Karlawish, J. H., and Berlin, J. A. (2002), “The Continuing Unethical Conduct of Underpowered Clinical Trials,” JAMA 288, 358–362. DOI: 10.1001/jama.288.3.358.
- Hazra, A., and Gogtay, N. (2016), “Biostatistics Series Module 5: Determining Sample Size,” Indian Journal of Dermatology 61, 496–504. DOI: 10.4103/0019-5154.190119.
- Higginson, A. D., and Munafò, M. R. (2016), “Current Incentives for Scientists Lead to Underpowered Studies With Erroneous Conclusions,” PLoS Biology, 14, e2000995. DOI: 10.1371/journal.pbio.2000995.
- Hubbard, R., and Bayarri, M. J. (2003), “Confusion Over Measures of Evidence (p’s) Versus Errors (α’s) in Classical Statistical Testing,” The American Statistician, 57, 171–178. DOI: 10.1198/0003130031856.
- IntHout, J., Ioannidis, J. P., and Borm, G. F. (2016), “Obtaining Evidence by a Single Well-powered Trial or Several Modestly Powered Trials,” Statistical Methods in Medical Research, 25, 538–552. DOI: 10.1177/0962280212461098.
- Ioannidis, J. P. (2005), “Why Most Published Research Findings Are False,” PLoS Medicine, 2, e124. DOI: 10.1371/journal.pmed.0020124.
- Ioannidis, J. P. (2008),“Why Most Discovered True Associations Are Inflated,” Epidemiology, 640–648. DOI: 10.1097/EDE.0b013e31818131e7.
- Ioannidis, J. P., Hozo, I., and Djulbegovic, B. (2013), “Optimal Type I and Type II Error Pairs When the Available Sample Size Is Fixed,” Journal of Clinical Epidemiology, 66, 903–910. DOI: 10.1016/j.jclinepi.2013.03.002.
- Johnson, V. E. (2013), “Revised Standards for Statistical Evidence,” Proceedings of the National Academy of Sciences 110, 19313–19317. DOI: 10.1073/pnas.1313476110.
- Johnson, V. E. (2014), “Reply to Gelman, Gaudart, Pericchi: More Reasons to Revise Standards for Statistical Evidence,” Proceedings of the National Academy of Sciences, 111, E1936–E1937. DOI: 10.1073/pnas.1400338111.
- Kimmelman, J., Mogil, J. S., and Dirnagl, U.(2014), “Distinguishing Between Exploratory and Confirmatory Preclinical Research Will Improve Translation,” PLoS Biology, 12, e1001863. DOI: 10.1371/journal.pbio.1001863.
- Lakens, D., Adolfi, F. G., Albers, C. J., Anvari, F., Apps, M. A., Argamon, S. E., Baguley, T., Becker, R. B., Benning, S. D., Bradford, D. E. et al. (2018), “Justify Your Alpha,” Nature Human Behaviour 2, 168–171. DOI: 10.1038/s41562-018-0311-x.
- Lamberink, H. J., Otte, W. M., Sinke, M. R., Lakens, D., Glasziou, P. P., Tijdink, J. K., and Vinkers, C. H. (2018), “Statistical Power of Clinical Trials Increased While Effect Size Remained Stable: An Empirical Analysis of 136,212 Clinical Trials Between 1975 and 2014,” Journal of Clinical Epidemiology 102, 123–128. DOI: 10.1016/j.jclinepi.2018.06.014.
- Lane, D. M., and Dunlap, W. P.(1978), “Estimating Effect Size: Bias Resulting From the Significance Criterion in Editorial Decisions,” British Journal of Mathematical and Statistical Psychology, 31, 107–112. DOI: 10.1111/j.2044-8317.1978.tb00578.x.
- Lee, C. J., and Schunn, C. D. (2011), “Social Biases and Solutions for Procedural Objectivity,” Hypatia 26, 352–373. DOI: 10.1111/j.1527-2001.2011.01178.x.
- Leek, J. T., and Jager, L. R. (2017), “Is Most Published Research Really False?” Annual Review of Statistics and Its Application, 4, 109–122. DOI: 10.1146/annurev-statistics-060116-054104.
- Lenth, R. V. (2001), “Some Practical Guidelines for Effective Sample Size Determination,” The American Statistician, 55, 187–193. DOI: 10.1198/000313001317098149.
- Makel, M. C., Plucker, J. A., and Hegarty, B. (2012), “Replications in Psychology Research: How Often Do They Really Occur?” Perspectives on Psychological Science, 7, 537–542. DOI: 10.1177/1745691612460688.
- Martin, G., and Clarke, R. M. (2017). Are Psychology Journals Anti-Replication? A Snapshot of Editorial Practices, Frontiers in Psychology, 8, 1–6.
- McLaughlin, A. (2011), “In Pursuit of Resistance: Pragmatic Recommendations for Doing Science Within One’s Means,” European Journal for Philosophy of Science, 1, 353–371. DOI: 10.1007/s13194-011-0030-x.
- McShane, B. B., Gal, D., Gelman, A., Robert, C., and Tackett, J. L. (2017), “Abandon Statistical Significance,” arXiv preprint arXiv:1709.07588.
- Miller, J., and Ulrich, R. (2016), “Optimizing Research Payoff,” Perspectives on Psychological Science 11, 664–691. DOI: 10.1177/1745691616649170.
- Moonesinghe, R., Khoury, M. J., and Janssens, A. C. J. (2007), “Most Published Research Findings Are False–But a Little Replication Goes a Long Way,” PLoS Medicine 4, e28. DOI: 10.1371/journal.pmed.0040028.
- Mudge, J. F., Baker, L. F., Edge, C. B., and Houlahan, J. E. (2012). “Setting an Optimal α That Minimizes Errors in Null Hypothesis Significance Tests,” PloS One 7, e32734. DOI: 10.1371/journal.pone.0032734.
- Nord, C. L., Valton, V., Wood, J., and Roiser, J. P. (2017), “Power-up: A Reanalysis of ‘Power Failure’ in Neuroscience using Mixture Modelling,” Journal of Neuroscience, 3592–16.
- OpenScienceCollaboration (2015), “Estimating the Reproducibility of Psychological Science,” Science, 349, aac4716.
- Ploutz-Snyder, R. J., Fiedler, J., and Feiveson, A. H. (2014), “Justifying Small-n Research in Scientifically Amazing Settings: Challenging the Notion That Only? Big-n? Studies Are Worthwhile,” Journal of Applied Physiology, 116, 1251–1252. DOI: 10.1152/japplphysiol.01335.2013.
- Richard, F. D., Bond C. F. Jr, and Stokes-Zoota, J. J. (2003), “One Hundred Years of Social Psychology Quantitatively Described,” Review of General Psychology, 7, 331–363. DOI: 10.1037/1089-2680.7.4.331.
- Roos, J. M. (2017), “Measuring the Effects of Experimental Costs on Sample Sizes.” under review, available at: http://www.jasonmtroos.com/assets/media/papers/Experimental_Costs_Sample_Sizes.pdf
- Sakaluk, J. K. (2016), “Exploring Small, Confirming Big: An Alternative System to the New Statistics for Advancing Cumulative and Replicable Psychological Research,” Journal of Experimental Social Psychology, 66, 47–54. DOI: 10.1016/j.jesp.2015.09.013.
- Schulz, K. F., and Grimes, D. A. (2005), “Sample Size Calculations in Randomised Trials: Mandatory and Mystical,” The Lancet 365, 1348–1353. DOI: 10.1016/S0140-6736(05)61034-3.
- Senn, S. (2012), “Misunderstanding Publication Bias: Editors Are Not Blameless After All,” F1000Research 1.
- Siler, K., Lee, K., and Bero, L. (2015), “Measuring the Effectiveness of Scientific Gatekeeping,” Proceedings of the National Academy of Sciences 112, 360–365. DOI: 10.1073/pnas.1418218112.
- Smaldino, P. E., and McElreath, R. (2016), “The Natural Selection of Bad Science,” Royal Society Open Science, 3, 160384. DOI: 10.1098/rsos.160384.
- Song, F., Y. Loke, and Hooper, L. (2014), “Why Are Medical and Health-related Studies Not Being Published? A Systematic Review of Reasons Given by Investigators,” PLoS One 9 (10), e110418. DOI: 10.1371/journal.pone.0110418.
- Spiegelhalter, D. (2017), “Trust in Numbers,” Journal of the Royal Statistical Society, Series A, 180, 948–965. DOI: 10.1111/rssa.12302.
- Stanley, T., Jarrell, S. B., and Doucouliagos, H. (2010), “Could It Be Better to Discard 90% of the Data? A Statistical Paradox,” The American Statistician 64, 70–77. DOI: 10.1198/tast.2009.08205.
- Sterling, T. D., Rosenbaum, W. L., and Weinkam, J. J. (1995), “Publication Decisions Revisited: The Effect of the Outcome of Statistical Tests on the Decision to Publish and Vice Versa,” The American Statistician, 49, 108–112. DOI: 10.2307/2684823.
- Szucs, D., and Ioannidis, J. P. (2016), “Empirical Assessment of Published Effect Sizes and Power in the Recent Cognitive Neuroscience and Psychology Literature,” bioRxiv, 071530.
- van Assen, M. A., van Aert, R. C., Nuijten, M. B., and Wicherts, J. M. (2014), “Why Publishing Everything is More Effective Than Selective Publishing of Statistically Significant Results,” PLoS One, 9, e84896. DOI: 10.1371/journal.pone.0084896.
- van Dijk, D., Manor, O., and Carey, L. B. (2014), “Publication Metrics and Success on the Academic Job Market,” Current Biology, 24, R516–R517. DOI: 10.1016/j.cub.2014.04.039.
- Vasishth, S., and Gelman, A. (2017), “The Illusion of Power: How the Statistical Significance Filter Leads to Overconfident Expectations of Replicability,” arXiv preprint arXiv:1702.00556.
- Walker, A. M. (1995), “Low Power and Striking Results—A Surprise But Not a Paradox,” Mass Medical Soc. 332 (16), 1091–1092. DOI: 10.1056/NEJM199504203321609.
- Wei, Y., and Chen, F. (2018), “Lowering the p Value Threshold–Reply,” JAMA 320, 937–938.
- Wilson, B. M., and Wixted, J. T. (2018), “The Prior Odds of Testing a True Effect in Cognitive and Social Psychology,” Advances in Methods and Practices in Psychological Science, 2515245918767122.
- Yeung, A. W. (2017), “Do Neuroscience Journals Accept Replications? A Survey of Literature,” Frontiers in Human Neuroscience, 11, 468.
Appendix
Here, we introduce some compact notation that will be useful for expressing distributional quantities of interest. Particularly, the probabilities comprising the distribution of a study across the eight categories are expressed as , where
indicates the truth (a = 0 for null, a = 1 for alternative),
indicates the statistical finding (b = 0 for negative, b = 1 for positive), and
indicates publication status. As examples, we could write
, or
. We also use a plus notation to add over subscripts, so, for instance,
.
As motivated above, we consider properties that result from a scientist or group of scientists stochastically allocating T resources (not studies per se) according to a distribution across (psp, pwr). We denote the function n(pwr) as the required sample size to obtain a power of pwr. Presuming the incentive to publish, the density of this distribution is taken proportional to ENP(psp, pwr), which we express as(A1)
(A1)
Consequently, the distribution of (psp, pwr) across attempted studies has density(A2)
(A2)
In turn, the distribution of (psp, pwr) across published studies has density(A3)
(A3)
Note particularly that . Hence, the distribution of (psp, pwr) across published studies is a concentrated version of the distribution describing how resources are deployed.
A1. Ecosystem Metrics: Further Details
We evaluate each ecosystem of interest on the basis of the following five metrics.
A1.1.Publication Rate and the Number of Studies Attempted/Published
If T units of resources are deployed according to , then we expect that
studies will be attempted, where
Similarly, studies will be published, with
The ratio , which does not depend on T, is of evident interest, as the publication rate (PR) for attempted studies.
A1.1.2. The Reliability (REL)
A highly relevant metric for the scientific ecosystem is the proportion of published findings that are correct. In all the ecosystems, we consider in this article, we make the assumption that only positive results are published (i.e., B = 0). Therefore, we can express reliability (REL) simply as
More generally, in ecosystems where negative results might be published (i.e., ), the reliability would equal the proportion of published articles that reach a correct conclusion, i.e.,
A1.1.3. Breakthrough Discoveries (DSCV)
The ability of the scientific ecosystem to produce breakthrough findings is an important attribute. We quantify this in terms of spending T resource units yielding an expectation of breakthrough results. Here, a true breakthrough result is defined as a TP and published study that results from a psp value below a threshold, that is, a very surprising positive finding that gets published and also happens to be true. If we set the breakthrough threshold as psp < 0.05, then
Note that we can also write this out in terms of the density of attempted studies, :
where we define the function:
.
We also wish to quantify the reliability of those published studies with psp values below a threshold of 0.05. We have that:
A1.1.4. The Median Power of Published Studies (
)
We already mentioned the relevance of the psp marginals of (A2) and (A3). In a similar vein, the marginal distributions of pwr under each of these distributions are readily interpreted metrics of the ecosystem. We define as the median of pwr under the
distribution.
A1.1.5. The Balance between Exploration and Confirmation (
)
There has been much discussion about the desired balance between researchers looking for a priori unlikely relationships versus confirming suspected relationships put forth by other researchers; see for example, Sakaluk (Citation2016) and Kimmelman, Mogil, and Dirnagl (Citation2014). The marginal distribution of psp arising from describes the balance between exploration versus confirmation for attempted studies, while the psp marginal from
does the same for published studies. More specifically, we report the interquartile ranges of these marginal distributions for a given ecosystem.
A1.1.6. The Accuracy of Published Research
Consider extending to
, where:
, and where
is the density of a standard uniform distribution; and
is the density of a two-component mixture distribution such that:
; and
.
In order to obtain a sample from the distribution of published effect sizes, we proceed according to the following steps.
Set w = 1, q = 1 and b = 1. While w < 1000:
Draw a Monte Carlo realization of
from
.
Simulate a two-sample Normally distributed dataset,
, with a sample size of
, a true difference in population means of
, and a true variance of
.
Run a standard t-test on
, and obtain the estimated effect size,
, and the two-sided p-value,
.
If
AND
, then do:
If
AND
, AND
, then do:
Set
.
Then we have that is a Monte Carlo sample from the conditional distribution of effect sizes given that studies are both positive and published. We also have that
is a Monte Carlo sample from the conditional distribution of effect sizes given that studies are positive, and published, and true.
Using these Monte Carlo samples, we can easily approximate the following metrics of interest:
Mean Absolute Published Effect Size (
). Approximated as the mean of
.
Proportion of Published Effect Sizes that are Negative (
). Approximated as the proportion of
that are less than zero.
The “Type S” error. Approximated as the proportion of
less than 0.
The “Type M” error. Approximated as the mean of the absolute effect size divided by the true effect size:
.
A2. The Probability of Publication as a Function of Both psp and pwr
In Section 3, the chance of publishing a positive study in our framework depended only on novelty via
For Section 4, a convenient choice of function to represent a journal policy of requiring an a priori sample size justification would be:(A4)
(A4)
So A is reduced more when power is lower, with the extent of the reduction parameterized by . Specifically, c95 is the value for pwr at which the multiplicative reduction is near negligible (factor of 0.95), while c50 is the value for pwr at which the multiplicative reduction is a factor of 0.5. We conduct our experimentation using
, with the following rationale. If a journal does require an a priori sample size justification, a claim of 80% power is the typical requirement. Hence, a study which really attains 80% power is not likely to suffer in its quest for publication, motivating
.
A3. Additional Results
Supplemental materials
The supplementary material includes additional figures which plot various metrics of interest across the entire set of ecosystems considered.
Table A.1 A comparison between ecosystems with both a sample size requirement and and ecosystems without a sample size requirement and
.