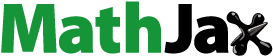
ABSTRACT
Aiming at reducing the effect of time delay on the control performance of magnetorheological (MR) damper semi-active suspension, a control strategy with strong robustness to time delay is investigated in this study, including the force controller of MR damper, the robust controller and the predictive compensator. The force controller of MR damper is built based on the hyperbolic tangent function; the robust controller is built based on the simplified model of car body lateral vibration and is designed by μ-synthesis method considering the effect of time delay; then the Smith predictive model and the lateral velocity predictor are designed, which form the predictive compensator of semi-active controller to reduce the effect of time delay. To validate the effectiveness of the proposed control strategy, several conventional semi-active controllers are designed as comparative objects. The simulation results show that the proposed semi-active control strategy can maintain good performance when time delay is in the range of 0–100 ms and has better robustness to time delay compared with the conventional control strategy.
1. Introduction
As the core part of high-speed rail, high-speed trains (HSTs) play an important role in railway modernization. The suspension system of HSTs is very important to the handling stability and ride comfort of the train [Citation1,Citation2]. Generally, the suspension system can be divided into three types: passive suspension, active suspension and semi-active suspension. A large number of studies show that [Citation3–5] the semi-active suspension can provide better performance with lower cost and complexity, which has advantages of less energy consumption, low cost and convenient maintenance.
The performance of semi-active suspension largely depends on the semi-active control strategy. The earliest research on semi-active control strategy can be traced back to the famous sky hook damper (SH) control proposed by Karnopp [Citation6] in 1974. Subsequently, many semi-active control strategies based on SH control were proposed, including Rakheja-Sankar (RS) control [Citation7], ground hook damper (GH) control [Citation8], no-jerk SH control [Citation9] and acceleration driven damping (ADD) control [Citation10]. Meanwhile, a lot of advanced control methods are used in semi-active suspension control. Liao and Wang [Citation11] used the semi-active LQG controller using acceleration feedback to control the 9-DOF vehicle model, and later extended it to the 17-DOF vehicle model [Citation12]. Chen [Citation13] applied generalized predictive control to semi-active suspension control. Zong et al. [Citation14] designed a semi-active robust controller based on H∞ theory, and verified that the controller can effectively suppress the lateral vibration of car body through the 17-DOF vehicle model.
In many studies, simulation results show that the semi-active suspension can achieve good performance. However, in practical application, due to the inevitable time delay from the signal collection, transmission, controller calculation, actuator response and so on, the actual control force deviates from the desired control force, which may greatly degrade the performance. Take magnetorheological (MR) semi-active suspension as an example, the time delay mainly comes from the response time of MR damper itself. The existing relevant research [Citation15–18] shows that the response time of MR damper is about dozens to hundreds millisecond, and there are obvious differences in the response time for dampers of different models and sizes. Generally, the response time of large-size dampers will be relatively large.
In recent years, some scholars have studied the effect of time delay on semi-active suspension. Wang [Citation19] pointed out that the effect of time delay on semi-active suspension and active suspension is different. For the former, time delay will only cause the change of vibration reduction performance, while for the latter, too large time delay will cause system instability. Cha et al. [Citation16] studied the effect of time delay on buildings with large-size MR dampers when different control strategies are adopted. Qin et al. [Citation20] gave a comprehensive analysis for the influence of controllable damper with time delay on different semi-active suspension control strategies based on the quarter vehicle model. Liao et al. [Citation21] designed several semi-active controllers based on SH, ADD and Mixed SH-ADD control strategy, applied them to the 50-DOF model of HSTs and analysed the effect of time delay. The simulation results show that the performance will degrade with the increase of time delay, even worse than the passive control.
Scholars have done relevant research on the control strategy for semi-active suspension with time delay. Zhao et al. [Citation17] designed a controller based on the fuzzy control strategy for the MR semi-active suspension and used the Smith predictive compensation method to compensate the time delay. However, this method needs obtain the road disturbance and the accurate model of the controlled object in advance, which is difficult to achieve in practical application. Zhang et al. [Citation22] studied the semi-active control strategy of air suspension based on LMI and H∞ theory, which has good robustness to parameter uncertainty and time delay. Wang et al. [Citation23] proposed two Taylor series compound robust control methods to solve the time delay compensation problem of MR semi-active suspension.
At present, there have been many studies on the control strategy of active suspension with time delay, among which robust control is widely used [Citation24–27], but studies on semi-active suspension are relatively few, especially for semi-active suspension of HSTs. Therefore, this paper investigates a control strategy to reduce the effect of time delay on the control performance of MR semi-active suspension of HSTs.
The structure of this paper is as follows. After the introduction, the 17-DOF model of HSTs is established and the track irregularity is numerically simulated in Section 2. In Section 3, based on the Bouc–Wen model, the dynamic model of MR damper is established as the actuator of semi-active suspension, and the MR semi-active suspension with time delay is built. In Section 4, the core part of this paper, a hyperbolic tangent model is proposed to fit the results of Bouc–Wen model, on which the force calculator and force controller of MR damper are established based. Then a simplified model of car body lateral vibration is established. Based on the simplified model, the robust controller is designed by μ-synthesis method after transforming time delay into model perturbation. Later, the Smith predictive compensation model is built based on the simplified model and the lateral velocity predictor is designed based on phase-lead compensator, which forms the predictive compensator together. In Section 5, the performance of the proposed control strategy is evaluated by simulation, and several conventional controllers are designed for comparison and analysis. The final conclusion of this paper is in Section 6.
2. Analytical model of HST
When studying the HST semi-active suspension control strategy, it is necessary to establish an appropriate dynamics model of HSTs, which needs to reflect the real situation as much as possible, but high complexity will make the design of the control strategy difficult.
In this paper, the 17-DOF dynamics model of HSTs (17-DOF model) is used to study the control strategy. 17-DOF model is a good choice with comprehensive consideration of control algorithm design and control performance analysis. And it is used wildly in the research about lateral vibration control of HSTs, due to including all lateral motion degrees of freedom for a single vehicle and reflecting lateral dynamic of the vehicle realistically.
The model assumes that the car body, bogie and wheel set are rigid, and considers the lateral, yaw and roll motion of the car body and two bogies, and the lateral and yaw motion of four wheelsets, which can basically reflect the actual lateral vibration of HSTs when driving on the track. The specific form of the model is described in Refs. [Citation14,Citation28]. Figure shows the 17-DOF model using MR damper as secondary lateral adjustable damper, and Table lists the input and output of the model. By comparing with the credible data from other published high-quality papers, the 17-DOF model built in this paper can be adopted, and the verification of it is presented in Appendix B.
Figure 1. 17-DOF dynamic model of HSTs with MR damper [Citation14,Citation28].
![Figure 1. 17-DOF dynamic model of HSTs with MR damper [Citation14,Citation28].](/cms/asset/4b2c649b-7ff7-4a40-bb1a-e0eb6e2b8ce0/taut_a_2277492_f0001_ob.jpg)
Table 1. Input and output of 17-DOF model.
Random track irregularity is the primary cause of the vibration of HSTs, which can be regarded as the excitation input of dynamic model of HSTs. Meanwhile, the lateral vibration of HSTs is mainly caused by lateral alignment track irregularity and cross-level track irregularity. Therefore, in this paper, the lateral alignment track irregularity and cross-level track irregularity based on German low interference spectrum are selected as the track excitation of the model, and their power spectral densities (PSDs) are described as follows, respectively [Citation29].
(1)
(1)
(2)
(2) where Ω is the spatial frequency (rad/m); Ωc, Ωr and Ωs are truncated frequencies (rad/m); Aa and Av are roughness coefficients (m·rad) and b is half of the wheel rolling circle distance. The PSDs only describe the frequency domain characteristics of the track irregularity, and the time series describing track irregularity needs to be obtained according to (1) and (2) for simulations. In this paper, a trigonometric series method based on frequency domain sampling is used to simulate the irregularity signal [Citation30].
3. MR semi-active suspension with time delay
The time delay of semi-active suspension can be divided into collection time delay and execution time delay [Citation21]. Under the existing technical conditions, the time delay caused by signal collection, processing and transmission is negligible [Citation36], so this paper only considers the execution time delay caused by the response time of the MR damper. Based on relevant material (see Appendix C), the achievable delay is determined as 0–100 ms.
In this paper, the modified Bouc–Wen model proposed by Spencer [Citation31] is used to describe the dynamics of MR damper. And the specific form of MR damper with time delay is as follows:
(3)
(3)
(4)
(4)
(5)
(5)
(6)
(6)
(7)
(7) where F is the damping force; I is the command current; c1 represents the viscous damping at low velocities; c0 represents the viscous damping at high velocities; x is the piston relative displacement; y is the internal displacement and z is the evolutionary variable; α, β, γ, n and A are parameters used to adjust the size and shape of hysteresis loop; τ is the response time delay, ranging from 0 to 100 ms. The best parameter values when the Bouc–Wen model is used to describe dynamics of MR damper are listed in Table .
Table 2. Parameter values of the modified Bouc–Wen model [Citation14].
Figure is the schematic of MR semi-active suspension with time delay, in which the MR damper with time delay generates the actual control force after receiving the command current calculated by the semi-active controller. However, there will be a deviation between the actual force and the desired because of time delay, which causes a degradation of control performance. To reduce the negative effect of time delay, a semi-active control strategy is proposed by combining the robust control and Smith predictive compensation method in this paper.
4. Robust predictive compensation control strategy
4.1. Force calculator and force controller of MR damper
The control force and command current are difficult to calculate through the Bouc–Wen model, while they are often needed in semi-active control strategy. Therefore, it’s necessary to establish the force calculator and force controller of MR damper. The former is used to calculate the output control force of MR damper, while the latter can calculate the command current to adjust MR damper.
The curve of hyperbolic tangent function is similar with the velocity force versus curve of MR damper, which can simulate the strong nonlinearity of MR damper with few parameters and high accuracy. Some scholars have described the dynamic characteristics of MR damper through hyperbolic tangent model [Citation23]. In this paper, a modified hyperbolic tangent model is proposed to describe MR damper, as shown in (8), and the force calculator and force controller are established based on the model.
(8)
(8) where FMR is the damping force (N), I is the command current (A), v is the piston relative velocity (mm/s), a is the piston relative acceleration, and k1, k2, b1 and b2 are the parameters to be fitted. To facilitate the solution of I, it’s assumed that k1 and k2 are functions of I, b1 and b2 are functions of the vibration state vm of the damper, and vm is defined as the root-mean-square (RMS) value of v in the last 1 s, as shown in (9).
(9)
(9)
The parameters of hyperbolic tangent model are fitted through the simulation results of Bouc–Wen model. After a series of fitting, the specific forms of k1, k2, b1 and b2 are determined, as shown in (10) and (11). Table lists the final fitting results.
(10)
(10)
(11)
(11) Replace a by v(n)–v(n–1) and the force calculator is obtained based on (8) and parameters in Table , which takes the command current I, the velocity v(n) and v(n–1) as input and control force of MR damper as the output.
Table 3. Fitting results of the hyperbolic tangent model.
Then apply the hyperbolic tangent model to the force controller. It’s clear that when the vibration state of MR damper is certain, if I = Imax, F = Fmax; if I = Imin, F = Fmin, which means the damping force F can change from Fmin and Fmax. In this paper, Imax = 1, Imin = 0. And the solution of command current is divided into the following two cases:
Case 1: When the desired control force Fi ∈[-∞, Fmin)∪[Fmax, +∞), or Fi∈[–∞, Fmax)∪[Fmin, +∞), Fi cannot be completely tracked and can only be approached as close as possible. Let e1 = |Fi–Fmax |, e1 = |Fi–Fmax |, and I is determined through e1 and e1: when e1 ≥ e2, I = Imin; e2>e1, I = Imax.
Case 2: When the desired control force Fi ∈[Fmin, Fmax], or Fi∈ [Fmax, Fmin], the command current can be obtained by solving a quadratic equation with one variable based on (16). The specific form is as follows:
(12)
(12)
(13)
(13)
(14)
(14)
(15)
(15)
(16)
(16)
I is the final command current output and limited through (17).
(17)
(17) Finally, the force controller of MR damper is determined, which takes the desired control force F, the velocity v(n) and v(n–1) as input and the estimated value of the command current as the output.
4.2. Simplified model of car body lateral vibration
Since the control purpose of this paper is to suppress the lateral vibration of the car body, a simplified model of the lateral vibration of the car body is developed with reference to the research of Zong et al. [Citation14], which is used to ensure the accuracy of the model and facilitate the subsequent controller design. Ignoring the influence of bogie yaw and roll motion on car body, the motion equation of lateral, yaw and roll of car body is as follows.
Car body lateral motion:
(18)
(18) Car body yaw motion:
(19)
(19)
Car body roll motion:
(20)
(20) The lateral vibration of car body is the coupled result of lateral, yaw and roll motion in practice. In this paper, two measuring points located on the bottom of car body are selected to measure the lateral vibration of car body, which displacements are y1 and y2 respectively, and the relationship between yc, ψc and θc and y1, y2 is as follows:
(21)
(21) By regarding the lateral motion velocity of the front and rear bogies
t1 and
t2 as external excitations, the control forces F1 and F2 generated by the MR damper as control force inputs, and the lateral acceleration of the car body
1 and
2 as the measurement outputs, a 3-DOF simplified model of car body lateral vibration (simplified model) is developed (Figure ).
The state space equation describing the simplified model is presented in (22) based on Equations (18)–(20).
(22)
(22) To facilitate writing, define the following variables: yYc = (y1–y2)/2, yYt = (yt1–yt2)/2, ΔyY = (yYc–yYt)/2, yLc = (y1 + y2)/2, yLt = (yt1 + yt2)/2, ΔyL = (yLc–yLt)/2. And the specific form of x, y, w, u, A, B1, C, D1 and D2 is as follows:
4.3. Robust controller design
The following is the design of the robust controller, the core of the control system, whose function is to calculate the desired control force according to the vibration signal measured by the sensor. In this paper, a robust controller is built based on the simplified model, which is designed by the μ-synthesis method considering the effect of time delay.
The lateral vibration of the car body includes yaw and rolling pendulum motion (the coupling of lateral and roll motion), so the controller is divided into yaw controller and rolling pendulum controller. According to the simplified model, state space models describing the yaw and rolling pendulum motion of car body are presented as follows:
Yaw motion model of car body:
(23)
(23) where
Rolling pendulum motion model of car body:
(24)
(24) where
The yaw and rolling pendulum motion model takes uY and uL as the control force input, takes yY and yL as the measurement output. yY and yL can be obtained by measuring the acceleration of the car body
1 and
2. uY and uL are the control forces calculated by the controller, which can be converted into F1 and F2.
Figure 4. Closed loop control system of car body lateral vibration with time delay: (a) closed loop control system for yaw motion and (b) closed loop control system for rolling pendulum motion.
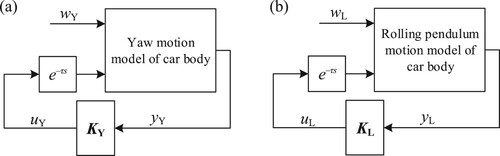
Assuming that the control force output by the controller can be fully tracked, a car body lateral vibration closed-loop control system with time delay in input channel is developed, on which the yaw motion controller KY and rolling pendulum motion controller KL are designed based (Figure ).
To design the robust controller, the time delay e−τs in the input channel is transformed into the model perturbation Δτ of the control system in this paper and the process is shown in Figure .
First, let Δ1 = e−τs–1, e−τs decomposes into parallel connection of Δ1 and 1. According to the robust control theory, the following requirements need to be met: when τ > 0, ||Δ1||∞ < 1, so further conversion is required.
Then draw amplitude frequency curve of Δ1 in different τ and fit the upper bound Wc so that |Wc|>|Δ1| is established in all frequency ranges, which is shown in (25). And the comparison of Wc and Δ1 in different τ is shown in Figure .
(25)
(25) Finally, normalize Δ1, let Δτ = Δ1Wc−1, ||Δτ||∞<1, so that time delay e−τs is transformed into model perturbation Δτ (Figure ).
According to the performance requirements of the controller, specify the controlled output. First, the controller needs to suppress the lateral vibration of the car body, which is mainly concentrated in the low-frequency range within 0.5–10 Hz, so the lateral vibration outputs yY and yL are weighted through the band-stop filter Wy to obtain the controlled outputs zyY and zyL. Meanwhile, the control force controller should be concentrated in the low-frequency range as much as possible, so the control forces uY and uL are weighted through the high-pass filter Wu to obtain the controlled outputs zuY and zuL. Finally, the design structure of the robust controller for yaw and rolling pendulum motion is shown in Figure .
(26)
(26) The “musyn” function in the robust controller toolbox of Matlab® is used to calculate the controllers KY and KL respectively. The final controller is integrated by KY and KL which takes
1 and
2 as input and F1 and F2 as output.
1 and
2 are measured by the sensor, then yY and yL are obtained after operation 1 shown in (27). uY and uL are obtained according to yY and yL, which are further transformed into desired control forces F1 and F2 after operation 2 shown in (28). Considering the contribution of yaw and rolling pendulum motion to the lateral vibration of car body and comparing the results of a series of weight values, select wL = 8 and wY = 1.
(27)
(27)
(28)
(28) Finally, the robust controller and the force controller form the semi-active controller (Figure ).
4.4. Predictive compensator design
4.4.1. Smith predictive compensation model
To reduce the effect of time delay, this paper adopts Smith predictive compensation control method [Citation32], whose schematic is shown in Figure . Where Gc(s) represents the transfer function of the controller, Gp(s) and Ĝp(s) represent the actual and estimated transfer function of the controlled object, τ represents time delay.
The transfer functions of the close-loop system with and without Smith predictive compensation model are described as (29) and (30). It can be found that the denominator of the transfer function will not contain e(−τs) after Smith predictive compensation model is added if both the estimated transfer function of the controlled object and estimated value of time delay are accurate (Ĝp(s) = Gp(s)), which means the effect of time delay is eliminated.
(29)
(29)
(30)
(30) However, it’s difficult to obtain accurate model describing dynamic system of HSTs and the track irregularity is difficult to measure using sensors directly in practice. Therefore, it’s not practicable to directly use Smith predictive compensation method to reduce the effect of time delay.
The actual controlled object is the whole model of HSTs in this paper, but the purpose is supressing the lateral vibration of car body. Therefore, regarding the simplified model as an approximation of the whole model of HSTs, the Smith predictive compensation method of MR semi-active suspension system is proposed (Figure ).
The Smith predictive compensation model includes the simplified model and force calculator, takes the command current I1 and I2, the relative velocity of front and rear MR damper d1 and
d2, and lateral velocity of front and rear bogie
t1 and
t2 as input and takes the predictive value of lateral acceleration of front and rear ends of car body
p1 and
p2 as output. I1 and I2 are calculated by semi-active controller,
d1,
d2,
t1 and
t2 can be measured by sensors. The Smith predictive compensation model is an approximation of the actual model of HST to predict
1 and
2 to reduce the effect of time delay.
4.4.2. Lateral velocity predictor
1 and
2 depend on I1, I2 and
d1,
d2,
t1 and
t2 for the simplified model, among which I1 and I2 are obtained through the semi-active controller and not delayed. Therefore, to obtain more accurate
p1 and
p2, it’s necessary to design predictor to predict
d1,
d2,
t1 and
t2, which is called lateral velocity predictor in this paper.
The transfer function of predictor is Gv(s), whose amplitude frequency characteristics and phase frequency characteristics meet (31) ideally, where τ is the prediction length, φ is the leading angle, and ω is the frequency of the input signal.
(31)
(31) The ideal predictor is not possible to realize, but possible to approach in a certain frequency range. In this paper, a predictor is designed to predict
d1,
d2,
t1 and
t2 based on the phase-lead compensator that is often used in the research of time delay problems [Citation33–35].
As shown in (32), the transfer function describing the lateral velocity predictor can be regarded as the combination of two phase-lead compensators and one proportional amplification link, where the parameters av and Tv determine the characteristics of the predictor. In (33), φm is the maximum lead angle, ωm is corresponding frequency of φm, and |Gc(ωm)| = 1. The predictor can advance the phase of the signal by about φm near ωm with the amplitude not too distorted.
(32)
(32)
(33)
(33) If the frequency component of the signal is concentrated near fm and prediction length is τ, then ωm = 2πfm and φm = 360fmτ, parameters av and Tv can be obtained through (33).
(34)
(34)
d1,
d2,
t1 and
t2 are collectively referred to as the lateral velocity in this paper. The frequency domain analysis of the lateral velocity is carried out to find out its main frequency components, before designing the predictor. Figure shows the amplitude frequency curve of
d1,
d2,
t1 and
t2 when passive control is adopted and it can be found that the frequency component concentrated mainly in the range of 2–4 Hz, and the main frequency is about 2.8 Hz.
The amplitude frequency curve of the lateral velocity is related to the level of excitation, running speed, and it's also the reflection of the vibration mode. The vibration model mainly depends on the parameters related to mass and stiffness in the suspension, which will not change during semi-active control. Meanwhile, this paper assumes that the level of excitation is constant and the running speed is 300 km/h. Therefore, it should be similar to Figure when the semi-active control is applied.
In summary, the characteristics of the lateral velocity in frequency domain include concentrated in low frequency, and stable under certain working conditions. Therefore, the predictor proposed above can be used to predict it.
The predictor is designed with fm = 2.8 Hz and ωm = 5.6π rad/s, then φm = 504τ and the design parameters av and Tv can be obtained according to (34). It can be found that av and Tv are only related to τ. Therefore, when τ is known, the predictor can be obtained directly through (35). Figure is the frequency characteristic curve of the predictor with different τ.
(35)
(35) It can be seen that the magnification is about 0 and the lead angle is about 504τ near 2.8 Hz, which meets the requirements; In the range of 0–2.8 Hz, the phase can be approximately advanced, but the magnification will be reduced. In the range of more than 2.8 Hz, the leading effect of phase is poor, and the magnification of high-frequency components in the signal is amplified, which means the performance of predictor on high-frequency vibration is very poor. In practical, it is necessary to add a low-pass filter in front of the predictor to limit its high-frequency response.
Figure 12. Frequency characteristic curve of the predictor with different time delay: (a) amplitude frequency curve and (b) phase frequency curve.
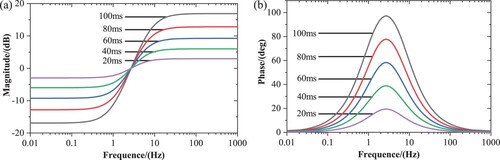
Apply the proposed predictor to predict d1,
d2,
t1 and
t2 in different τ when passive control is adopted respectively. The accuracy of prediction is evaluated through the prediction error ep, shown in (36), where va represents the actual values of
d1,
d2,
t1 and
t2, and vp represents their corresponding predicted values.
(36)
(36) The results of ep are shown in Table . It can be found that ep increases with τ, which indicates that the accuracy of prediction decreases with τ, but still remains in an acceptable range when τ = 100 ms, so the designed predictor can be used to predict the lateral velocity. Meanwhile, to show the accuracy of prediction clearly, the comparison between predicted value and the actual value when τ = 50 ms is shown in Figure .
Figure 13. Validation of the lateral velocity predictor when τ = 50 ms: (a) d1; (b)
d2; (c)
t1; (d)
t2.
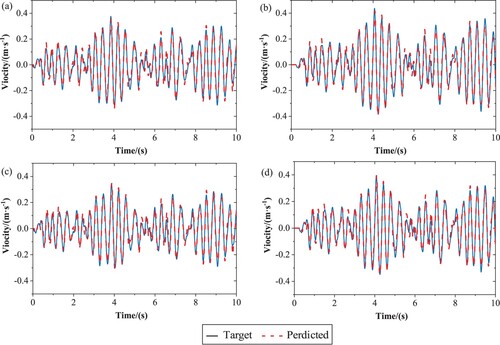
Table 4. Errors of the lateral velocity predictor with different τ.
4.5. Robust predictive compensation control system for MR semi-active suspension
According to the study above, the control strategy consists of three parts: robust controller that calculates the desired control force of the front and rear ends of the car body, force controller that calculates the command current according to the control force and the motion velocity of MR damper, and the predictive compensator that predicts the lateral velocity including d1,
d2,
t1 and
t2.
The final semi-active control system is shown in Figure . Collecting the relative velocity of the front and rear MR dampers d1 and
d2, the lateral motion velocity of the front and rear bogies
t1 and
t2, and the command current I1 and I2 as the input of the predictive compensator to calculate
p1 and
p2, and the robust controller calculates the desired control force of front and rear ends of the car body Fi1 and Fi2 through
p1 and
p2, then command currents I1 and I2 are obtained through the force controller. After the MR damper receives I1 and I2, the actual control forces Fa1 and Fa2 generated suppress the lateral vibration of the car body.
5. Simulation results
For brevity, the semi-active controller designed in this paper is denoted as controller 1. To invalidate the effectiveness of the proposed control strategy, several controllers are designed for comparing and analysing. It is noted that these controllers use the same force controller. Because the lateral vibration of car body of different vehicle models is relatively similar, only one vehicle model is adopted. Its parameters are presented in Appendix A.
The robust controller considering the effect of time delay is designed in 4.3, and the semi-active controller composed of it and the force controller is denoted as controller 2. Compared with controller 1, controller 2 does not contain predictive compensator. And a conventional robust controller is designed, not considering the effect of time delay, which is similar to that of controller 2 and does not include Wc and Δτ in the structure of controller design, and the semi-active controller formed by its combination with the force controller is denoted as controller 3. Finally, according to the SH-ADD control strategy [Citation21,Citation36], an active controller is designed, which consists of two parts to generate the control forces F1 and F2 respectively, and the specific mathematical form is as follows:
(37)
(37) where Cmax and Cmin represent the maximum and minimum damping coefficients of the damper. Considering the MR damper used in this paper, Cmax = 40 kN/m·s−1, Cmin = 7 kN/m·s−1 are taken here. α1 and α2 is the conversion coefficient, which is obtained here through the (38), where
and
are the root mean square values within the last 10 s. For convenience, it is replaced by the root mean square value within 10 s when passive control is adopted, and then α1 and α2 are determined in advance. The semi-active controller composed of SH-ADD active controller and the inverse model is denoted as controller 4.
(38)
(38) The control performance of each controller is evaluated by simulation compared with the passive control, which keeps the lateral damping of secondary suspension at 25 kN/m·s−1. The “Bogacki–Shampine” solver is adopted for the simulation, the step size is fixed at 1e–3s, and the total simulation time is 25 s.
When time delay is 50 and 100 ms, the time-domain curves (8–10 s) of car body acceleration with different controllers adopted are shown in Figures and , RMS values of car body acceleration and lateral ride index [Citation37] are listed in Table . It can be found that controller 1 can always suppress the lateral vibration of the car body, and the control performance of controller 2 is weaker than that of controller 1, but better than that of controllers 3 and 4, which indicates that controller 1 has strong robustness to time delay, followed by controller 2, and controllers 3 and 4 are the worst, which do not consider the effect of time delay in their design.
Figure 15. Time histories of the car body accelerations with different controller adopted when τ = 50 ms: (a) lateral accelerations, (b) yaw accelerations and (c) roll accelerations.
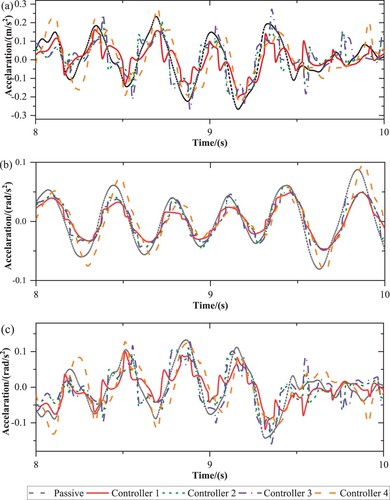
Figure 16. Time histories of the car body accelerations with different controllers adopted when τ = 100ms: (a) lateral accelerations, (b) yaw accelerations and (c) roll accelerations.
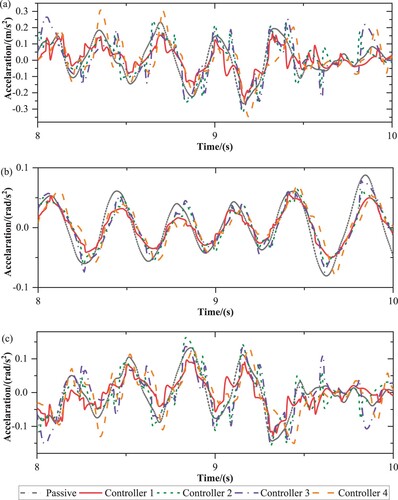
Table 5. RMS values of car body accelerations and lateral ride index with different controller adopted when τ = 50, 100 ms.
Figures and show PSDs of car body acceleration with different controllers adopted when the time delay is 50 and 100 ms. It can be found that the car body lateral vibration is mainly concentrated in the range of 0.5–10 Hz, and the vibration around 1 and 3 Hz is strong. Controller 1 can still suppress the lateral vibration of the car body better around 3 Hz well, while the vibration around 3 Hz is significantly strengthened when other controllers are adopted.
Figure 17. PSDs of the car body accelerations with different controller adopted when τ = 50 ms: (a) lateral accelerations, (b) yaw accelerations and (c) roll accelerations.
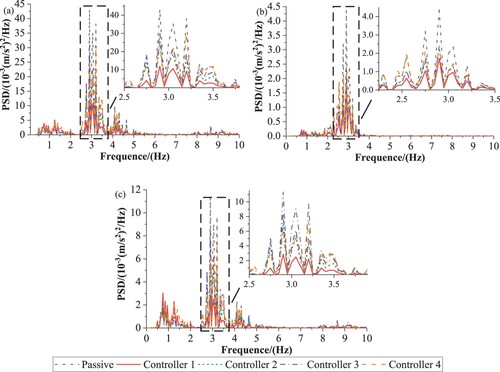
Figure 18. PSDs of the car body accelerations with different controllers adopted when τ = 100 ms: (a) lateral accelerations, (b) yaw accelerations and (c) roll accelerations.
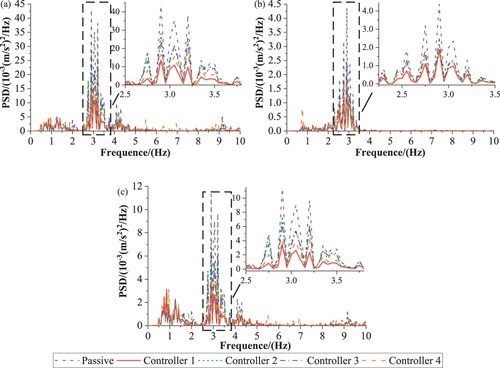
To further validate robustness to time delay of controller 1, Figures and show the RMS values of car body acceleration and lateral ride index with different controllers adopted when the time delay increases from 0 to 100 ms.
Figure 19. RMS values of car body accelerations with different controllers adopted: (a) lateral accelerations, (b) yaw accelerations and (c) roll accelerations.
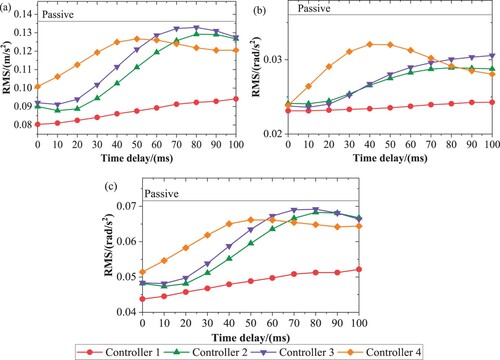
Figure 20. Lateral ride index with different controller adopted: (a) lateral accelerations, (b) yaw accelerations and (c) roll accelerations.

For controller 1, the increase of RMS values and lateral ride index with time delay is the slowest. Even when time delay is 100 ms, better control performance can still be achieved. The performance of controllers 2 and 3 is close, but that of controller 2 is slightly stronger. The robustness to time delay of controller 4 is the worst, whose performance will degrade obviously when the delay is small and increase first and then decrease with the increase of delay. Meanwhile, the difference between controllers 1 and 2 is only whether there is a predictive compensator, the comparison of their results can prove that the predictive compensator can effectively reduce the effect of time delay.
To sum up, the semi-active controller proposed in this paper has strong robustness to time delay and can maintain good control performance when time delay of MR semi-active suspension in the range of 0–100 ms.
6. Conclusion and discussion
Aiming at the time delay problem of MR semi-active suspension of HSTs, this paper proposes a control strategy with strong robustness to time delay, including the force controller of MR damper, robust controller and predictive compensator. The effectiveness of the control strategy is validated by simulation. Some conclusions are summarized as follows.
Each controller can maintain good performance without time delay, but the performance of conventional controller will be significantly reduced with the increase of time delay, even worse than passive control.
The control strategy proposed in this paper can maintain good performance when time delay is no more than 100 ms, which indicates it has strong robustness to time delay.
The comparison of the controller with and without predictive compensator shows that the predictive compensator proposed in this paper can significantly reduce the effect of time delay.
On the other hand, this paper only considers the vibration control of vehicles and ignores the interaction between vehicles, track and railway. Currently, some experts have studied the vehicle–track–bridge dynamic interaction and proposed the vehicle–track–bridge dynamic interaction model [Citation38], which is a structural system consisting of a high-speed railway bridge and a high-speed train. Meanwhile, the tuned mass dampers (TMDs), including multiple tuned mass dampers (MTMDs), series tuned mass dampers (STMDs) and so on, have been adopted for vibration control of railway bridges [Citation39–42]. Based on that, the problem of applying semi-active control to TMDs and the vibration control of a structural system composed of vehicle, track and bridge need to be explored in depth.
Disclosure statement
No potential conflict of interest was reported by the author(s).
Additional information
Funding
Reference
- Li WF, Xie ZC, Zhao J, et al. Fuzzy finite-frequency output feedback control for nonlinear active suspension systems with time delay and output constraints. Mech Syst Signal Proc. 2019;132:315–334. doi: 10.1016/j.ymssp.2019.06.018
- Liu YJ, Zeng Q, Tong SC, et al. Adaptive neural network control for active suspension systems with time-varying vertical displacement and speed constraints. IEEE Trans Ind Electron. 2019;66(12):9458–9466. doi:10.1109/TIE.2019.2893847
- Ata WG, Salem AM. Semi-active control of tracked vehicle suspension incorporating magnetorheological dampers. Veh Syst Dyn. 2017;55(5):626–647. doi:10.1080/00423114.2016.1273531
- Biglarbegian M, Melek W, Golnaraghi F. A novel neuro-fuzzy controller to enhance the performance of vehicle semi-active suspension systems. Veh Syst Dyn. 2008;46(8):691–711. doi:10.1080/00423110701585420
- Sun SS, Yang J, Li WH, et al. Development of a novel variable stiffness and damping magnetorheological fluid damper. Smart Mater Struct. 2015;24(8):085021, doi:10.1088/0964-1726/24/8/085021
- Karnopp D, Crosby MJ, Harwood RA. Vibration control using semi-active force generators. J Eng Ind-Trans ASME. 1974;96(2):619–626. doi:10.1115/1.3438373
- Rakheja S, Sankar S. Vibration and shock isolation performance of a semi-active “On–Off” damper. J Vibr Acoust Stress Reliab Des. 1985;107(4):398–403. doi:10.1115/1.3269279
- Valasek M, Novak M, Sika Z, et al. Extended ground-hook – new concept of semi-active control of truck's suspension. Veh Syst Dyn. 1997;27(5–6):289–303. doi:10.1080/00423119708969333
- Ahmadian M, Song XB, Southward SC. No-jerk skyhook control methods for semiactive suspensions. J Vib Acoust-Trans ASME. 2004;126(4):580–584. doi:10.1115/1.1805001
- Savaresi SM, Silani E, Bittanti S. Acceleration-driven-damper (ADD): an optimal control algorithm for comfort-oriented semiactive suspensions. J Dyn Syst Meas Control-Trans ASME. 2005;127(2):218–229. doi:10.1115/1.1898241
- Wang DH, Liao WH. Semi-active suspension systems for railway vehicles using magnetorheological dampers. Part I: system integration and modelling. Veh Syst Dyn. 2009;47(11):1305–1325. doi:10.1080/00423110802538328
- Wang DH, Liao WH. Semi-active suspension systems for railway vehicles using magnetorheological dampers. Part II: simulation and analysis. Veh Syst Dyn. 2009;47(12):1439–1471. doi:10.1080/00423110802538336
- Chen CJ, Wang KY. Modeling and analysis of lateral semi-active suspension system of high-speed train. J Vib Shock. 2006;4(04):151–154–169 + 184. doi: 10.13465/j.cnki.jvs.2006.04.041
- Zong LH, Gong XL, Xuan SH, et al. Semi-active H∞ control of high-speed railway vehicle suspension with magnetorheological dampers. Veh Syst Dyn. 2013;51(5):600–626. doi:10.1080/00423114.2012.758858
- Koo JH, Goncalves FD, Ahmadian M. A comprehensive analysis of the response time of MR dampers. Smart Mater Struct. 2006;15(2):351–358. doi:10.1088/0964-1726/15/2/015
- Cha YJ, Agrawal AK, Dyke SJ. Time delay effects on large-scale MR damper based semi-active control strategies. Smart Mater Struct. 2013;22(1):015011. doi: 10.1088/0964-1726/22/1/015011
- Zhao YS, Zhou KK, Li ZX, et al. Time lag of magnetorheological damper semi-active suspensions. J Mech Eng Chin Ed. 2009;45(7):221–227. doi:10.3901/JME.2009.07.221
- Zhu MF, Lv G, Zhang CP, et al. Delay-dependent sliding mode variable structure control of vehicle magneto-rheological semi-active suspension. IEEE Access. 2022;10:51128–51141. doi:10.1109/ACCESS.2022.3173605
- Wang YM. Research on control of semi-active suspension of high speed railway vehicles [dissertation]. Chengdu: Southwest Jiaotong University; 2002.
- Qin YC, Zhao F, Wang ZF, et al. Comprehensive analysis for influence of controllable damper time delay on semi-active suspension control strategies. J Vib Acoust-Trans ASME. 2017;139(3):031006, doi:10.1115/1.4035700
- Liao YY, Liu YQ, Yang SP. Semiactive control of high-speed railway vehicle suspension systems with magnetorheological dampers. Shock Vib. 2019;2019:5279380. doi: 10.1155/2019/5279380
- Zhang ZY, Wang JB, Wu WG, et al. Semi-active control of air suspension with auxiliary chamber subject to parameter uncertainties and time-delay. Int J Robust Nonlinear Control. 2020;30(17):7130–7149. doi:10.1002/rnc.5169
- Wang JC, Lv LF, Ren JY, et al. Time delay compensation control using a Taylor series compound robust scheme for a semi-active suspension with magneto rheological damper. Asian J Control. 2022;24(5):2632–2648. doi: 10.1002/asjc.2674
- Du HP, Sze KY, Lam J. Semi-active H∞ control of vehicle suspension with magneto-rheological dampers. J Sound Vibr. 2005;283(3–5):981–996. doi:10.1016/j.jsv.2004.05.030
- Li HY, Liu HH, Gao HJ, et al. Reliable fuzzy control for active suspension systems with actuator delay and fault. IEEE Trans Fuzzy Syst. 2012;20(2):342–357. doi:10.1109/TFUZZ.2011.2174244
- Li HY, Jing XJ, Karimi HR. Output-feedback-based H∞ control for vehicle suspension systems with control delay. IEEE Trans Ind Electron. 2014;61(1):436–446. doi:10.1109/TIE.2013.2242418
- Afshar KK, Javadi A, Jahed-Motlagh MR. Robust H∞ control of an active suspension system with actuator time delay by predictor feedback. IET Contr Theory Appl. 2018;12(7):1012–1023. doi:10.1049/iet-cta.2017.0970
- Chen CJ. Active and semi-active control of high-speed trains. Chendu: Southwest Jiaotong University Press; 2015.
- Claus H, Schiehlen W. Modeling and simulation of railway bogie structural vibrations. Veh Syst Dyn. 1998;29:538–552. doi:10.1080/00423119808969585
- Chen CJ, Li HC. Track irregularity simulation in frequency domain sampling. J China Railw Soc. 2006;3(03):38–42.
- Spencer BF, Dyke SJ, Sain MK, et al. Phenomenological model for magnetorheological dampers. J Eng Mech-ASCE. 1997;123(3):230–238. doi:10.1061/(ASCE)0733-9399(1997)123:3(230)
- Smith OJM. A controller to overcome dead time. ISA Trans. 1959;6(2):28–33.
- Golestan S, Guerrero JM, Abusorrah AM. MAF-PLL with phase-lead compensator. IEEE Trans Ind Electron. 2015;62(6):3691–3695. doi: 10.1109/tie.2014.2385658
- Chen PC, Tsai KC. Dual compensation strategy for real-time hybrid testing. Earthq Eng Struct Dyn. 2013;42(1):1–23. doi:10.1002/eqe.2189
- Tavazoei MS, Tavakoli-Kakhki M. Compensation by fractional-order phase-lead/lag compensators. IET Contr Theory Appl. 2014;8(5):319–329. doi:10.1049/iet-cta.2013.0138
- Savaresi SM, Spelta C. Mixed sky-hook and ADD: approaching the filtering limits of a semi-active suspension. J Dyn Syst Meas Control-Trans ASME. 2007;129(4):382–392. doi:10.1115/1.2745846
- Wang FT. Vehicle system dynamics. Beijing: China Railway Publishing House; 1994.
- Zhai WM, Han ZL, Chen ZW, et al. Train-track-bridge dynamic interaction: a state-of-the-art review. Veh Syst Dyn. 2019;57(7):984–1027. doi:10.1080/00423114.2019.1605085
- Kahya V, Araz O. Series tuned mass dampers in train-induced vibration control of railway bridges. Struct Eng Mech. 2017;61(4):453–461. doi:10.12989/sem.2017.61.4.453
- Araz O, Kahya V. Series tuned mass dampers in vibration control of continuous railway bridges. Struct Eng Mech. Jan 2020;73(2):133. doi: 10.12989/sem.2020.73.2.133
- Kahya V, Araz O. A simple design method for multiple tuned mass dampers in reduction of excessive vibrations of high-speed railway bridges. J Fac Eng Archit Gazi Univ. 2020;35(2):607–618. doi: 10.17341/gazimmfd.493102
- Araz O, Kahya V. Optimization of multiple tuned mass dampers for a two-span continuous railway bridge via differential evolution algorithm. Structures. 2022;39:29–38. doi:10.1016/j.istruc.2022.03.021
- Hua YY, Zhu SY, Shi X. High-performance semiactive secondary suspension of high-speed trains using negative stiffness and magnetorheological dampers. Veh Syst Dyn. 2022;60(7):2290–2311. doi:10.1080/00423114.2021.1899251
Appendices
Appendix A.
Vehicle model parameters
Table A1. Parameter values and definitions of the 17-DOF model.
Appendix B.
Verification of the dynamic model of HSTs
Table B1. Comparison of time domain results at different speed.
Figure B1. Comparison of PSDs of car body lateral acceleration: (a) ref. [Citation12], (b) model in this paper.
![Figure B1. Comparison of PSDs of car body lateral acceleration: (a) ref. [Citation12], (b) model in this paper.](/cms/asset/97b08ba9-ffc6-4746-8320-4c8da14a69f0/taut_a_2277492_f0021_oc.jpg)
Figure B2. Comparison of PSDs of car body yaw acceleration: (a) ref. [Citation12], (b) model in this paper.
![Figure B2. Comparison of PSDs of car body yaw acceleration: (a) ref. [Citation12], (b) model in this paper.](/cms/asset/8e3d530b-dc71-4c87-9c88-d189f46d1f74/taut_a_2277492_f0022_oc.jpg)
Figure B3. Comparison of PSDs of car body roll acceleration: (a) ref. [Citation12], (b) model in this paper (“Passive-on”: the command current of MR damper is maximum, “Passive-off”: the command current of MR damper is minimum, and the speed is 200 km/h, the track irregularities is from the German high-speed high disturbance).
![Figure B3. Comparison of PSDs of car body roll acceleration: (a) ref. [Citation12], (b) model in this paper (“Passive-on”: the command current of MR damper is maximum, “Passive-off”: the command current of MR damper is minimum, and the speed is 200 km/h, the track irregularities is from the German high-speed high disturbance).](/cms/asset/131d342e-542f-428b-8914-1e15a918dee6/taut_a_2277492_f0023_oc.jpg)
Figure C1. The change of damping force when the command current raises from 0.5 A to 1 A [Citation17] (RD-1005-3 MR damper).
![Figure C1. The change of damping force when the command current raises from 0.5 A to 1 A [Citation17] (RD-1005-3 MR damper).](/cms/asset/377b7ba1-ab08-43ac-8bf9-288a7ae5eed5/taut_a_2277492_f0024_oc.jpg)
Appendix C.
Relevant supporting material of the time delay discussed in this paper
Table C1. Response time under different system compliance [Citation17] (RD-1005-3 MR damper).