Abstract
In the semiconductor industry, virtual metrology (VM) is a cost-effective and efficient technique for monitoring the processes from one wafer to another. This technique is implemented by generating a predictive model that uses real-time data from equipment sensors in conjunction with measured wafer quality characteristics. Before establishing a prediction model for the VM system, appropriate selection of relevant input variables should be performed to maintain the efficiency of subsequent analyses considering the large dimensionality of the sensor data inputs. However, wafer production processes usually employ multiple sensors, which leads to cost escalations. Herein, we propose a variant of the sparse principal component analysis (PCA) called true sparse PCA (TSPCA). The proposed method uses a small number of input variables in the first few principal components. The main contribution of the proposed TSPCA is reducing the number of essential sensors. Our experimental results demonstrate that compared to the existing sparse PCA methods, the proposed approach can reduce the number of sensors required while explaining an approximately equivalent amount of variance.
Disclosure statement
No potential conflict of interest was reported by the author(s).
Data Availability Statement
The data that support the findings of this study are available from the corresponding author upon reasonable request.
Additional information
Funding
Notes on contributors
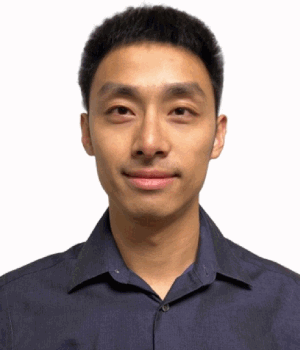
Yifan Xie
Yifan Xie received the B.S. degrees in statistics from Zhongnan University of Economics and Law, Wuhan, China and University of Glasgow, Glasgow, United Kingdom in 2015 and M.S. degrees in applied urban science and informatics from New York University, New York, NY, in 2016, economics and finance from Pompeu Fabra University, Barcelona, Spain, in 2018, and management research from Rutgers University, Newark, NJ, in 2020. He is currently a doctoral candidate in industrial and systems engineering at Rutgers University, New Brunswick, NJ. In addition to research, his is interested in the areas of machine learning algorithms, kernel method and data mining.
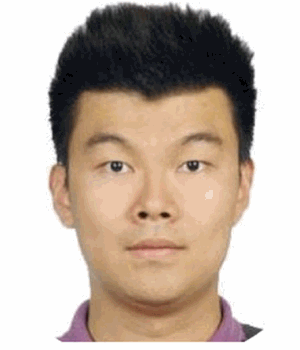
Tianhui Wang
Tianhui Wang received the B.S. degree in transportation engineering from Tongji University, Shanghai, China in 2014. He is currently a doctoral candidate in industrial and systems engineering at Rutgers University, New Brunswick, NJ. His research interests include data mining, optimisation methods in machine learning.
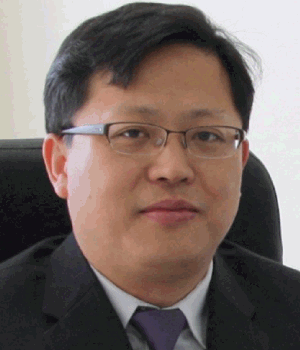
Young-Seon Jeong
Dr. Young-Seon Jeong is currently a Professor with the Department of Industrial Engineering, Chonnam National University, Gwangju, South Korea. He received the Ph.D. degree in industrial and systems engineering from Rutgers University in 2011. His research interests include statistical data mining and process analytics and monitoring from diverse applications.
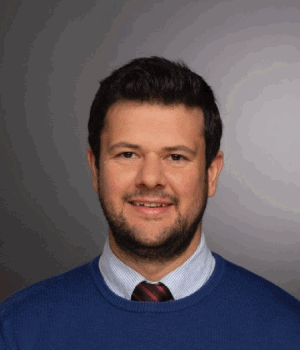
Ali Tosyali
Dr. Ali Tosyali is an Assistant Professor in the Department of MIS, Marketing, and Analytics in the Saunders College of Business at RIT. He received his MS and PhD in industrial and systems engineering from Rutgers University. His research focuses on developing new methodologies for various data-driven problems. His research interests lie broadly at the intersection of information systems, network science, machine learning, and complex systems, driven by applications in financial services analytics, social network analysis, opinion mining and analysis, and business analytics.”
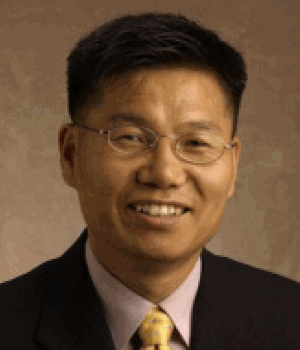
Myong K. Jeong
Dr. Myong K. Jeong is a Professor with the Department of Industrial and Systems Engineering and the Rutgers Center for Operations Research, Rutgers University. He has focused on developing data mining techniques, process monitoring and control procedures, and optimisation techniques for machine learning.