Abstract
The wear of a cutting tool can lead to tool failure and product quality deterioration, and thus timely maintenance of tools is crucial. Meanwhile, the wear of tools from a same population usually exhibits heterogeneous patterns. Therefore, this paper proposes a dynamic condition-based maintenance (CBM) policy for heterogeneous-wearing tools with considering the product quality deterioration caused by tool wear. The tool wear is modelled by an Inverse Gaussian (IG) process, and the wear rate is assumed to be a random variable to characterise the heterogeneity among tool wear processes. The posterior distribution of reciprocal of tool wear rate is dynamically estimated using the online wear data based on a Bayesian approach. Moreover, the impact of tool wear on product quality deterioration is modelled. The IG process is discretized into a discrete time Markov chain (DTMC). Under the frame of the DTMC, a cost function, containing product quality loss, preventive maintenance (PM) cost and corrective maintenance cost, is developed to determine the optimal PM threshold. The cost function updates dynamically with the dynamic estimation of tool wear rate and thus enables the optimal PM threshold to be dynamically revised. The effectiveness of the proposed CBM policy is demonstrated through a case study.
Acknowledgements
The authors are grateful to the editor and the two anonymous referees for their valuable comments and suggestions.
Disclosure statement
No potential conflict of interest was reported by the author(s).
Data availability statement
The authors confirm that the data supporting the findings of this study are available within the article [and/or] its supplementary materials.
Additional information
Funding
Notes on contributors
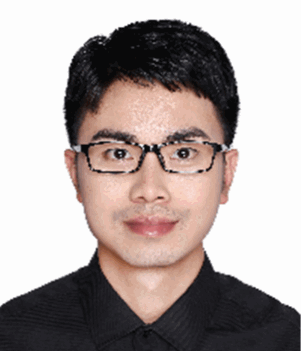
Biao Lu
Biao Lu is currently an assistant professor with the Department of management science and engineering, College of Economics and Management, Nanjing University of Aeronautics and Astronautics. He received his doctoral degree in Mechanical Engineering in 2019 from Shanghai Jiao Tong University, China. He was an Academic Visitor to the Department of Industrial Systems Engineering and Management, College of Design and Engineering, National University of Singapore from 1 Sep 2022 to 1 Sep 2023. His research interests include prognostics and health management, maintenance modelling and optimisation and smart manufacturing systems. He is currently the author of more than 20 journal papers in the field of reliability and production engineering.
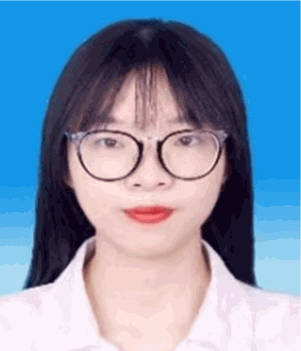
Yumei Luo
Yumei Luo is a graduate student majoring in Management Science and Engineering in College of Economics and Management, Nanjing University of Aeronautics and Astronautics. She received her B.S. degree in Engineering Management from Nanchang Hangkong University in 2017. Her research direction is quality and reliability engineering.