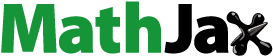
ABSTRACT
Situated expectancy-value theory (SEVT) posits that students’ expectancies for success, task values, and perceived costs affect their performance in STEM courses. Less is known about how students’ majors and timing of course completion affect motivational processes. Using the SEVT framework, this study utilised a convergent mixed methods approach to investigate academic motivation among students (N = 646) who enrolled in introductory biology at a large public university. Survey data revealed that students majoring in Biology were more motivated than students from other majors, although academic major was not directly associated with final grade. Upper-level students reported significantly lower competence-related beliefs and task values and perceived higher effort cost compared to first-year students. Upper-level students also earned significantly lower grades than first-year students. Both academic major and year in college had significant indirect effects on final course grades. Interviews with students (N = 31) helped explain and extend quantitative results. This study demonstrates that understudied factors (i.e. academic major and year in college) are important predictors of motivation in a gateway biology course and identifies themes that contribute to student motivation in this context.
Introduction
Increasing the national STEM workforce will require retention of undergraduate students in STEM majors. However, nearly half of the undergraduate students who pursue STEM disciplines ultimately change fields (National Center for Education Statistics Citation2013). Many who leave STEM majors do so after taking introductory courses that serve as ‘gatekeepers’ to degree progression. These gateway undergraduate science courses tend to be graded more stringently than non-STEM courses (Tomkin and West Citation2022) and have high failing (D/F) or withdrawal (W) rates (Freeman, Haak, and Wenderoth Citation2011). Students in gateway STEM courses describe course-related anxiety (England, Brigati, and Schussler Citation2017) and concerns (Meaders et al. Citation2020) that are associated with lower grades and intent to change majors. Low grades in gateway STEM courses may lead affected students to change their majors from a science to a non-science field (Stinebrickner and Stinebrickner Citation2014). These studies demonstrate that gateway STEM courses can present critical barriers to student persistence and STEM degree completion.
Motivational factors have been implicated in introductory biology performance. Motivation refers to a process of initiating and sustaining goal-directed behaviours and can both influence and be influenced by student performance (Anderman and Anderman Citation2020). For example, in postsecondary biology settings, low-performing students perceive less personal relevance in their academic coursework and have lower levels of intrinsic motivation than do higher-achieving students (Gasiewski et al. Citation2012; Moore Citation2007; Nacionales, Muyong, and Gavasan Citation2016). Many factors contribute to students’ academic motivation, including their self-beliefs, sense of belonging, goal orientation, values, perceived costs, mindset, and interest (Komarraju and Nadler Citation2013; Linnenbrink-Garcia and Patall Citation2015).
To create more inclusive academic environments, researchers have called for more rigorous study of what works to support motivation and achievement, for which students, and under what conditions (Sathy and Hogan Citation2022). This is particularly important in the context of introductory biology, which is required by many STEM undergraduate programs and as a prerequisite for numerous graduate and professional programs. The course enrolls students from a variety of STEM disciplines at different points in their undergraduate trajectories who may differ in their academic backgrounds, identities, and interests. A better understanding of the underlying motivations and academic outcomes in this heterogenous student population would help gateway science instructors create more inclusive spaces that better serve the needs of all students and encourage their ongoing participation in STEM. This study explores how undergraduate major and the timing of course enrollment in introductory biology might be related to learners’ motivation and achievement.
Theoretical framework: situated expectancy-value theory (SEVT)
Situated expectancy-value theory (SEVT) was used as the conceptual framework for this study. SEVT highlights the role of students’ competence-related beliefs, task values, and perceived costs in their motivation and achievement (for a review, see Rosenzweig, Wigfield, and Eccles Citation2019). Competence-related beliefs include students’ expectations for success and beliefs about their abilities. The perceived value of a task is what attracts an individual to engage in and persist in the task (Eccles et al. Citation1983). Task values include attainment value (aspects of the task that the student perceives as important to their identity), intrinsic value (beliefs about the enjoyment or interest in a task), and utility value (perceptions about the usefulness of the task for future goals). Costs concern perceived drawbacks associated with engaging in the task. Perceived costs include psychological cost (the emotional or psychological cost of completing the work, particularly the emotional and social cost of predicted anxiety and failure), opportunity cost (the degree to which executing a task prevents one from having the time or ability to perform other valuable things), and effort cost (perception of how much effort is required to finish a task and whether it is worthwhile).
According to SEVT, students are optimally motivated when they feel confident that they will be successful (competence-related beliefs), value a task or domain (task values), and perceive minimal cost to undertaking a task. These beliefs do not exist in a vacuum, however. Social scientists are increasingly focused on the way that individuals’ competence-related beliefs, task values, and perceived costs can be influenced by interpersonal and contextual factors including the sociocultural context. In fact, Eccles and Wigfield (Citation2020) recently renamed expectancy-value theory to ‘situated’ expectancy-value theory to emphasise the contextual nature of motivation. Situated views of motivation emphasise the fact that motivation is person and context dependent (Nolen Citation2020).
The relationship between competence-related beliefs, task values, perceived costs, and academic outcomes has been studied in secondary and postsecondary STEM contexts. For example, high school students’ competence-related beliefs and task values in mathematics and science were positively associated with high school GPA, the number of STEM courses taken, and students’ choice of STEM majors in college (Jiang, Simpkins, and Eccles Citation2020). Students’ expectations for success and task values have been shown to predict mathematics-related career attainment 15 years after high school graduation (Lauermann et al. Citation2017). In an undergraduate biology context, students’ competence-related beliefs and perceived costs were associated with their final course grades (Perez, Dai, et al. Citation2019). In a gateway chemistry course, students whose motivational profiles were ‘very high’ or ‘high’ completed more STEM courses and earned higher grades compared to students with a ‘low’ motivational profile (Perez, Wormington, et al. Citation2019).
The SEVT framework has also been used to understand motivational changes over time in STEM contexts. Across the first two years of college, undergraduate engineering students’ competence-related beliefs and task values decreased and they perceived higher costs associated with learning engineering (Robinson et al. Citation2019). Importantly, students with slower declines in motivation earned higher grades and were more likely to remain in an engineering major. Taken together, these studies point to the relevance of SEVT for understanding student motivation and learning in STEM contexts.
Effects of major and course enrollment timing on motivation
Students are typically more highly motivated to learn subjects that are aligned to their personal interests and identities (Renninger and Hidi Citation2016). It is reasonable to assume that Biology majors may show greater interest in learning biology compared to learners from other STEM majors. Presumably, a student majoring in Biology chose their major because of high interest, alignment with career goals, and/or positive self-beliefs about their biology capabilities. A variety of STEM majors require Introductory Biology courses (e.g. Animal Science, Kinesiology, Dietetics, Equine Science), but students in these majors may be less interested in the subject. Prior research has shown that students not majoring in science reported lower motivation, interest, and expert-level thinking in a genetics course compared to students majoring in science (Knight and Smith Citation2010). It may also be that students majoring in Biology are more motivated to succeed in introductory biology because the course is foundational to their major, whereas students from other majors may perceive the course as less relevant.
Students may also view the work required for their courses differently depending on their major. Some evidence has suggested that the effort required for a task may be perceived as a cost when the task is not aligned with one’s identity (Perez, Dai, et al. Citation2019). That is, non-Biology majors may be more likely than Biology majors to perceive introductory biology course tasks as costly because they may not be aligned with their academic goals or identity.
The timing of course enrollment (first-year vs later in the academic career) may also be associated with motivation and achievement. For instance, some students are unable to enroll in introductory biology courses during their first year of college because they have not yet met prerequisites (e.g. passing college algebra). These students may arrive in their biology courses with different academic beliefs and/or skill levels. In one study, upper-level students enrolled in a general chemistry course earned lower grades than their first-year counterparts in traditional (lecture-based) learning environments (Bastyr et al. Citation2022). The authors speculated that upper-level students entered the course with lower math preparation, which may have contributed to their relatively lower final grades.
Previous work has demonstrated that the motivation of STEM majors decreases as students move through their undergraduate career. Studies have documented motivational declines and potential barriers to maintaining high motivation among students pursuing STEM degrees (Cromley, Perez, and Kaplan Citation2016; Dai and Cromley Citation2014; Perez, Cromley, and Kaplan Citation2014, Citation2019; Robinson et al. Citation2019). Longitudinal studies have indicated that beliefs about competence, values, and other positive motivational constructs tend to decline across time periods as short as a single semester (Corpus, Robinson, and Wormington Citation2020; Kosovich, Flake, and Hulleman Citation2017) and across multiple years (Chouinard and Roy Citation2008; Fredricks and Eccles Citation2002; Jacobs et al. Citation2002; Watt Citation2004).
There are several possibilities for why students may lose motivation as they progress towards their degrees. It may be that upper-level students have adjusted their competence-related beliefs based on prior experience at the college level, consequently lowering their performance expectations. If upper-level students had college-level experiences that lowered their confidence for STEM coursework, they may begin to devalue similar work as a method of ego protection (Jacobs et al. Citation2002; Marsh et al. Citation2005). First-year students, on the other hand, have little or no prior experience at the college level and may feel more capable of succeeding and open to learning. While upper-level students may have experienced challenges and setbacks in college, first-year students have only their high school experiences to draw on, which were most likely successful.
The perceived effort costs associated with taking an introductory biology course may also be higher for upper-level students for several reasons. First, upper-level students may be more likely than first-year students to have competing priorities, such as a job or extracurricular activities, that require their time and effort. Second, upper-level students may be more likely to be part-time students, non-traditional students, or transfer students from community colleges. Third, some upper-level students may have been unsuccessful in their first attempt at a course and could be taking it for the second time. Prior evidence suggests such motivational differences. For example, students in their second year of college reported significantly higher costs associated with their coursework compared to students just beginning college (Robinson et al. Citation2019).
Finally, upper-level students may be more comfortable with didactic lecturing and more resistant to active learning practices compared with first-year students, especially if their prior college coursework did not use active learning techniques that require different skills for success. Although students feel like they learn more through passive lecture than active learning, the opposite is true (Deslauriers et al. Citation2019). These underexplored factors may also affect academic motivation and outcomes.
Study rationale and research questions
Academic major and enrollment year in college (i.e. first year, sophomore, junior, and senior) are important aspects of the sociocultural, intrapersonal, and interpersonal context of learning, which likely affect students’ motivation, learning, and performance. These factors affect the classes students must take, with whom they take their courses, when they enroll in certain courses, and what their short- and long-term goals for learning are.
In this study, we aimed to better understand the effect of academic major and timing of course enrollment on student outcomes in an introductory biology course. The course is required for a variety of undergraduate science majors at the participating institution (e.g. Kinesiology, Dietetics, Animal Science, Equine Science, Human Health Sciences, and Biomedical Engineering); non-science majors enroll in a separate general biology course. Students majoring in Biology or Neuroscience (both housed in the Biology Department) usually take the course early in their undergraduate studies as a prerequisite for more advanced coursework. Some students majoring in Biology or Neuroscience take the course later in their academic careers if they transferred from another college/university, are non-traditional students returning to undergraduate coursework after time away from school, or if they were unsuccessful in their first attempt at the course. Students majoring in other STEM disciplines have more flexibility in when they enroll in the course because it does not serve as a prerequisite for most of the courses required in other programs. Therefore, this introductory biology course context offered a unique way to directly compare biology motivation and course outcomes by both students’ academic majors and the timing of their course enrollment.
Despite evidence that, on average, learners’ motivation affects their performance in STEM courses, less is known about how the alignment of course discipline and students’ major may influence situated motivation and academic outcomes in a gateway course like this one. In addition, how the timing of course enrollment in introductory biology may be related to motivation and academic outcomes is understudied. To our knowledge, no studies have directly compared motivation and course outcomes between first-year and upper-level students in the same introductory biology course. Additionally, few studies have qualitatively investigated the factors that contribute to student motivation in this context. Understanding these relationships would signal ways to create learning environments that best support all students in this critically important gateway course and others like it. The following research questions guided our investigation:
RQ1.
Does motivation differ by academic major and timing of enrollment in an introductory biology course designed for science majors?
RQ2.
Do final course grades differ by major and timing of enrollment?
RQ3.
Does motivation mediate the relationship between academic major/timing of enrollment and final course grade?
RQ4.
How do students from different majors and who enroll in different years of study describe the factors that contribute to their academic motivation in Introductory Biology?
Methods
Using a convergent mixed-methods approach, we analyzed survey responses from 646 undergraduate students and semi-structured interview data from 31 undergraduate students enrolled in an Introductory Biology I course at a large public, four-year university in the southeastern United States. We reasoned that quantitative data would identify any differences in motivation and course outcomes that may exist, and that interview data analysed qualitatively would provide important context that would aid in and extend the interpretation of quantitative results. This approach allowed us to further understand statistical results by probing participants’ views in greater depth (Creswell and Plano Clark Citation2018). Consenting students were enrolled in one of four participating sections of the course during the Fall 2021 semester. This study was approved by the university’s Institutional Review Board (#60349 and #61595).
Course description
Introductory Biology I is a required course for the two majors housed in the Biology department (Biology and Neuroscience) as well as other science majors across the participating university such as Kinesiology, Animal Science, Dietetics, Human Health Science, Biomedical Engineering, and Equine Science. At the participating university, non-science majors enroll in a separate course designed for non-majors. Contact hours consisted of 150 minutes per week in a 16-week semester. Course topics included evolution, gene expression, cell division, inheritance, ecology, and biodiversity. Each of the four sections of the course was taught by a different instructor. Learning outcomes, grading schemes, homework questions, practice tests, and actual exams were uniform across sections. One of the four sections was taught by the first author of this study, who also developed course materials used by all sections. The course schedule was the same across sections except for adjustments for class meeting patterns. The course was structured in a ‘flipped’ classroom model in which all students read short (~1 page) summary passages and watched 10–15-minute pre-recorded lecture videos outside of class time. Reading materials and lecture videos were developed by the first author. Students turned in notes taken from the pre-class lecture videos for class credit to ensure that they were prepared for class. Class time was spent reviewing material and applying content with formative activities such as practice questions and worksheets. Students were encouraged to work together during class activities, but formal groups were not established. For all sections, overall course grades were determined by three multiple-choice semester exams (with the option to retake one semester exam, 41% of the final grade), a cumulative final exam (21% of the final grade), twice-weekly homework assignments in which the higher of two attempts was recorded (16% of the final grade), four mid-unit group quizzes (6% of the final grade), and an Earning for Learning category which included completion points for turning in notes from lecture videos, in-class worksheets, and participating in polling activities (16% of the final grade).
Participants and recruitment
All students enrolled in Introductory Biology I in the Fall 2021 semester were invited to participate in the survey via an announcement in the course learning management system during the third week of the semester. Bonus points were awarded regardless of whether students consented to share their survey data with researchers. Eighty-two percent of students enrolled in the class consented to be part of the research study (646 of 785 enrolled). Survey data were only analyzed from students who consented to be part of the research study. Survey participants (N = 646) included 387 first-year students and 259 upper-level students. Demographic information was obtained from university records and reflects institutional data categories (see ). The institutional data did not contain information about transgender or gender non-binary status. Fifty-eight percent of first-year students were Biology majors, 10% were Neuroscience majors, and 32% were enrolled in other majors. Sixteen percent of upper-level students were Biology majors, 3% were Neuroscience majors, and 80% were other majors (0.8% of the sample had missing data for major). Because Biology and Neuroscience majors both require introductory biology as a pre-requisite for major coursework and are housed in the same academic department at the participating institution, they were grouped together for analyses. Other science majors were grouped as ‘other’ because the course was not a prerequisite for upper-level coursework in most of these majors and the majors were housed in other science units.
Table 1. Student demographic information.
In Week 12 of the semester, a researcher visited the classrooms of three of the four participating course sections to describe the interview portion of the study and incentive for participating (a $10 gift card). Students who withdrew from the course were not recruited for interviews. Students in one section were not recruited for interviews because one of the authors was the instructor for that section. Interested students signed up for interviews via a secure web form and consented to participate at the beginning of the interview. Of the 31 interviewees, 17 (55%) were first-year students. Of those, 12 (71%) were Biology or Neuroscience majors and 5 were majoring in areas other than Biology/Neuroscience. Twelve of the 14 upper-level students interviewed were majoring in areas other than Biology/Neuroscience.
Quantitative phase
Measures
Student motivation was assessed via a self-report survey administered during the third and fourth weeks of the semester (before the first exam). Survey items from previously published studies were used to measure motivation, as described below. Students responded to all items using a 6-point, Likert-type scale. The full survey instrument is included as Supplemental Material. Students’ final course grades were obtained from university records.
Competence-related beliefs
The 4-item competence-related beliefs measure included items from Hecht et al. (Citation2019) and Perez et al. (Citation2019). All items had been used to assess the expectancy beliefs of students in undergraduate STEM courses. Items assessed students’ expectations for success in the course (e.g. ‘I expect to get a good grade in this course’). Cronbach’s alpha for the competence-related beliefs items was .81. Confirmatory factor analysis (CFA) was conducted to assess evidence of construct validity. CFA results confirmed the one-factor solution (χ2 (2) = 10.80, p = .005, RMSEA [90% CI] = .08[.04, .13], CFI = .99, SRMR = .02).
Subjective task values
Subjective task values (i.e. attainment, intrinsic, and utility value) were measured separately and treated as different constructs (Eccles and Wigfield Citation2020) using items adapted from Perez et al. (Citation2019) and Robinson et al. (Citation2019). Each task value construct was measured with four items previously used to measure the domain-specific values of undergraduate students in STEM contexts. CFA results demonstrated an acceptable fit; χ2 (51) = 322.05, p < .001, RMSEA [90% CI] = .09[.08, .10], CFI = .95, and SRMR = .05. These findings confirmed the three-factor solution, suggesting that these subjective task value constructs are empirically distinct from one another. Cronbach’s alpha was .71 for the attainment value items (e.g. ‘Being someone who is good at this biology course is important to me’), .91 for the intrinsic value items (e.g. ‘I find this biology course to be interesting’), and .91 for the utility value items (e.g. ‘This biology course will be useful for what I want to do after I graduate’).
Perceived costs
Three types of perceived cost – psychological cost, opportunity cost, and effort cost – were measured with items from Perez et al. (Citation2014) who used them to assess undergraduate students’ perceptions of cost in their biology courses. Each cost type was assessed with three items. CFA results for the three-factor solution showed an acceptable fit: χ2 (24) = 110.03, p < .001, RMSEA [90% CI] = .07[.06, .09], CFI = .97, and SRMR = .05. Cronbach’s alpha was .75 for the psychological cost items (e.g. ‘I’m concerned about being embarrassed if my work in this biology course is inferior to that of my peers’), .91 for the opportunity cost items (e.g. ‘I’m concerned about losing track of some valuable relationships because of the work required for this biology course’), and .81 for the effort cost items (e.g. ‘This biology course sounds like it really requires more effort than I’m willing to put into it’).
A CFA was used to assess the overall factor structure of the seven motivation constructs (i.e. competence-related beliefs, attainment value, intrinsic value, utility value, psychological cost, opportunity cost, and effort cost). Results confirmed the 7-factor solution, χ2 (254) = 1074.28, p < .001, RMSEA[90% CI] = .07[.07, .08], CFI = .92, and SRMR = .07.
Course grades
Students’ final course grades were gathered from institutional records. Grades were converted to a 5-point scale (A = 4.0, B = 3.0, C = 2.0, D = 1.0, E = 0.0, a failing grade at the participating institution; W = withdrawal).
Quantitative analyses
Boxplots were used to examine students’ motivation and final course grades by students’ academic major and timing of enrollment. We used Kruskal-Wallis tests with post-hoc Dunn’s multiple comparisons tests to compare means between student groups (research questions 1 & 2). These non-parametric tests that do not require particular data distributions were used because some variables exhibited a non-normal distribution (see Supplemental Material). There were no significant differences in motivational variables between sophomore, junior, and senior students, so these students were grouped as ‘upper-level’ students for all subsequent analyses. Students who withdrew from the course were excluded from the mean GPA calculations and from between-group comparisons.
To answer the third research question (i.e. ‘Does motivation mediate the relationship between academic major/timing of enrollment and final course grade?’), we conducted path modelling using Mplus 8.8. In the model, we used student major (Biology/Neuroscience = 1; other majors = 0) and enrollment status (first-year student = 1; upper-level student = 0) as predictors, the seven motivation constructs as mediators, and final course grades as a distal outcome variable. To control for possible differences between the four course sections, three dummy variables were included with the reference group designated as the course section taught by the instructor who designed the course materials. Controlling for gender, underrepresented minority status, and first-generation status did not change the statistical significance of results (see Supplemental Material), so these variables were omitted from the final model. Missing course grade data (about 5% of sample) were handled using full information maximum likelihood.
Model fit was comprehensively evaluated by examining the chi-square statistic and its associated p value, a root mean square error of approximation (RMSEA) less than or equal to .06, a comparative fit index (CFI) greater than or equal to .95, and a standardised root mean squared residual (SRMR) less than or equal to .08 (Asparouhov and Muthén Citation2018; Hu and Bentler Citation1999). Statistical significance of the indirect effects was determined when zero was not included in the 95% bootstrap confidence interval (CI) at the 2.5th and 97.5th percentiles (Preacher and Hayes Citation2008).
Qualitative phase
Interview Protocol
To address our fourth research question (i.e. How do students from different majors and who enroll in different years of study describe the factors that contribute to academic motivation in introductory biology?), we developed a semi-structured interview protocol based on SEVT. Fifteen pilot interviews were conducted in the Spring 2021 semester, and responses were used to modify the protocol to better align with the tenets of SEVT and to solicit more reflective insights from participants.
The first part of the interview focused on students’ major, future career plans, and the reasons for enrolling in Introductory Biology I when they did. The second part consisted of questions that addressed the motivation constructs from SEVT, probing students’ views of their competence-related beliefs, subjective task values, and perceived effort cost of the course. The full interview protocol is provided in the Supplemental Material. Interviews took place in Weeks 13 and 14 of the semester and were conducted via Zoom by undergraduate and graduate research assistants. Each interview lasted approximately 15 minutes. Transcripts were auto-generated on Zoom and de-identified by research assistants prior to analysis.
Interview analysis
We approached our qualitative data analysis with a constructivist orientation. As an instructor in the course with over a decade of experience, the first author applied her knowledge of the course context, the student population, and the tenets of SEVT to generate initial codes for the data that were most relevant to the interpretation of quantitative results (Morse Citation1997). The third author read through all transcripts, making initial notes about codes. Once a codebook was established by the team, the first and third authors individually reviewed all 31 interviews using the codebook and identified any additional codes that the initial analysis may have missed. Interviews were again read in full by the first author to identify cross-cutting themes reflected in the data and excerpts that extended and aided in the interpretation of quantitative results. The third and fourth authors independently read coded excerpts within each theme to determine whether they were aligned.
Coding disagreements were discussed by the full research team and resolved through consensus. Because our analytic goal was to explore the diversity of students’ perspectives about motivation rather than to establish a singular ‘correct’ interpretation, inter-rater reliability was inappropriate. In addition, we did not attempt to quantify our codes, which may have inadvertently suggested that certain findings were more important than others (Maxwell Citation2010).
Results
Descriptive statistics
displays descriptive statistics for the full sample as well as correlations between variables. All variables were significantly correlated, except for psychological cost, which was unrelated to intrinsic value and final course grade. Competence-related beliefs were positively correlated with subjective task values and negatively correlated with perceived costs. Competence-related beliefs and subjective task values were positively correlated, and perceived effort and opportunity costs were negatively correlated with final course grades.
Table 2. Descriptive statistics and bivariate correlations.
RQ 1:
Does motivation differ by academic major and timing of enrollment in an introductory biology course designed for science majors?
We first considered the effect of both academic major (Biology/Neuroscience vs. other majors) and timing of enrollment (first-year vs. upper-level) on all motivation variables (). First-year Biology/Neuroscience majors reported the highest levels of competence-related beliefs and value measures and the lowest level of effort costs. In contrast, upper-level students with majors other than Biology/Neuroscience had the lowest competence-related beliefs and task values and the highest effort costs.
Figure 1. Variation in motivation constructs by academic major and timing of enrollment. Students’ ratings on seven motivation constructs were compared by Kruskal-Wallis tests with post-hoc Dunn’s multiple comparisons tests for first-year and upper-level students separated by academic major (bio major = students with a biology/neuroscience major; other majors = students with other STEM majors). The center line is the median value (50th percentile), and the 25th to 75th percentiles of the dataset are represented by the box. The whiskers denote the 2.5th and 97.5th percentiles, and values beyond these upper and lower bounds are marked with dots. The mean value is indicated by the ‘+’.
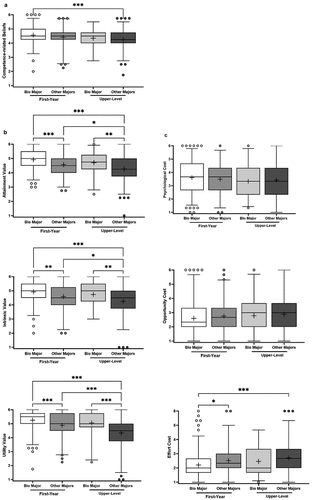
Kruskal-Wallis tests indicated significant differences by group in competence-related beliefs ( = 23.1, df = 3, p < .001), attainment value (
= 66.7, df = 3, p < .001), intrinsic value (
= 57.3, df = 3, p < .001), utility value (
= 88.3, df = 3, p < .001), and effort cost (
= 29.4, df = 3, p < .001). Pairwise comparisons revealed that first-year students who majored in Biology/Neuroscience reported significantly higher competence-related beliefs than upper-year students with other majors (p < .001; ).
First-year students who majored in Biology/Neuroscience reported significantly higher levels of attainment value than did first-year or upper-level students with other academic majors (p < .001 for all tests). Among students not majoring in Biology/Neuroscience, first-year students’ attainment value was significantly higher than upper-level students’ (p = .033; ). First-year students with Biology/Neuroscience majors also reported significantly higher intrinsic value than did first-year or upper-level students with other majors (p = .004 and p < .001, respectively; ). Among students with other majors, first-year students’ intrinsic value was higher than upper-level students’ (p = .027). Among upper-level students, Biology/Neuroscience majors reported higher intrinsic value than did other majors (p = .008).
First-year students with Biology/Neuroscience majors reported higher levels of utility value than did first-year or upper-level students with other majors (p < .001 for all tests), and among other majors, first-year students reported higher utility value than upper-level students (p < .001; ). Among upper-level students, students with Biology/Neuroscience majors reported higher utility value than did other majors (p < .001). In terms of effort cost, first-year Biology/Neuroscience majors perceived lower levels than first-year or upper-level other majors (p = .030 and p < .001, respectively; ) . Psychological and opportunity costs did not significantly differ between groups. Means and standard deviations of the motivation variables by major and timing of enrollment are included in the Supplemental Material.
RQ 2:
Do final course grades differ by major and timing of enrollment?
depicts the final course grades earned by first-year and upper-level students with Biology/Neuroscience vs. other academic majors. A Kruskal-Wallis test indicated significant differences in final course grades ( = 52.4, df = 3, p < .001). The follow-up pairwise comparisons indicated that first-year students earned significantly higher final course grades than upper-level students, regardless of their academic majors. First-year students with Biology/Neuroscience majors earned also significantly higher final course grades than upper-level students with Biology/Neuroscience majors or other majors (p < .001 for all tests; ). Further analysis showed that, whereas 73% of first-year students earned a final grade of an A or B in the course, only 46% of upper-level students earned an A or B. Upper-level students were also more likely to earn a D or E or to withdraw from the course (30% D, E, or Withdrawal rate for upper-level students vs. 10% for first-year students; ).
Figure 2. Final course grades by major and timing of enrollment. (a) a box and whisker plot of final course grades was plotted on a 5-point scale (4 = A, 3 = B, 2 = C, 1 = D, 0 = E) for first-year and upper-level students (bio major = students with a biology/neuroscience major; other majors = students with other STEM majors). The centre line is the median value (50th percentile), and the 25th to 75th percentiles of the dataset are represented by the box. The whiskers denote the 2.5th and 97.5th percentiles, and values beyond these upper and lower bounds are marked with dots. The mean value is indicated by the ‘+’. Kruskal-Wallis tests with post-hoc Dunn’s multiple comparisons tests were used to compare means. *p < .05, **p < .01, ***p < .001 (b) the proportion of students with each grade type (W = withdrawal; E = failing grade) was graphed by first-year and upper-level status and academic major.
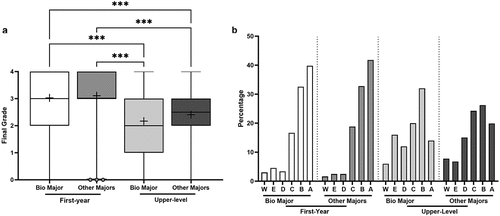
RQ3:
Does motivation mediate the relationship between academic major/timing of enrollment and final course grade?
Our path model allowed us to investigate whether the relationship between students’ major and time of enrollment and their final course grades depends on their level of motivation. The model had an approximate fit, (1) = 4.766, p = .029, RMSEA [90% CI] = .076 [.020, .151], CFI = .998, SRMR = .007. Although we focus our results on the tests of mediation, all path coefficients in the model are presented in . shows a simplified version that highlights statistically significant paths.
Figure 3. Path model illustrating significant relationships among variables. Reported coefficients are standardised. Correlations between motivation variables were included in the path model but are excluded from the figure for simplicity. *p < .05, **p < .01, ***p < .001.
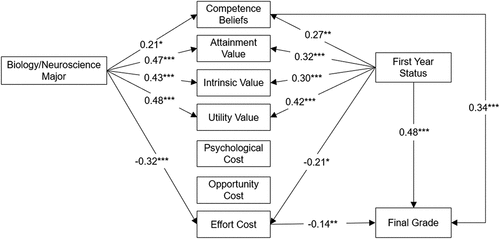
Table 3. Results of the path model.
As shown in , academic major was not directly associated with final course grades. However, path modelling allowed us to examine whether there was an indirect effect of major on course grades through motivational variables. We found that academic major was indirectly associated with course grades through its effects on students’ competence-related beliefs and the perceived effort cost of biology. Compared with those who majored in other disciplines, students who majored in Biology/Neuroscience tended to report higher competence-related beliefs and lower effort cost, which resulted in better grades. Whereas having stronger competence-related beliefs and perceiving less effort cost were associated with higher final course grades in our model, task values and psychological and opportunity costs were not significant predictors of final course grade.
We next examined whether the relationship between the timing of students’ enrollment and their course grade was mediated by student motivation. First-year status had a positive direct effect on grades. That is, first-year students performed better than upper-level students, even after controlling for motivation variables. We also found that competence-related beliefs mediated this relationship (i.e. first-year status had a significant indirect effect on students’ final grades through competence-related beliefs). Specifically, being a first-year student was associated with high perceived competence, which was in turn associated with better grades.
RQ 4:
How do students from different majors and who enroll in different years of study describe the factors that contribute to academic motivation in Introductory Biology?
Representative quotes and our interpretation of how qualitative data help explain and extend quantitative results have been included for each theme below. Longer interview segments are available from the first author upon request.
Theme 1: competence-related beliefs are based on prior experiences
An examination of interview transcripts revealed that students largely described their competence-related beliefs in relation to their previous experiences in biology. Many of those first-year students who were interviewed described positive experiences from high school as the basis for their competence-related beliefs. For example, one first-year Biology major explained, ‘I took bio in high school, so I feel like it’s a lot of like the same content that I already recognise, which I think has helped a lot’. Another first-year Biology major said that they were experiencing ‘pretty smooth sailing’ because the course was ‘a review from AP Biology’.
First-year students were also more likely to state that they were a ‘biology’ or ‘science’ person, even if they were not biology majors. For instance, one first-year Chemical Engineering major stated that he would ‘definitely’ be successful in the course because he was ‘pretty good at biology’. A first-year Human Health Sciences major stated that they ‘have a pretty good background. I took two years of honors biology in high school and really enjoyed it, so I have an idea of what I’m doing’, adding that ‘I’m more of a bio person … it comes more naturally to me’. All but one first-year student interviewed (a Human Nutrition major) expressed confidence that they would be successful in the course.
In contrast, upper-level students not majoring in Biology/Neuroscience described lower competence-related beliefs. Only half of the upper-level students interviewed stated unequivocally that they would be successful in the course. Most based their biology competence beliefs on their other college coursework. A junior Equine Science major stated that he was ‘better at other classes than biology’ and mentioned his tendency to ‘procrastinate in biology’. Another upper-level student described the course as ‘difficult for me’.
While only two upper-level students with a Biology/Neuroscience major took part in an interview, these students had very different prior experiences in biology that affected their competence-related beliefs. One, a non-traditionally aged junior, had positive prior experiences with biology that resulted in high competence beliefs: ‘I’ve taken a lot of biology background before so I feel like a lot of this course was kind of like a refresher almost’. The other, a Neuroscience major, was a sophomore retaking the course after an unsuccessful first attempt. She noted that she ‘struggles’ and that her ability ‘could be better’.
These data suggest that first-year students rely heavily on their experiences in high school when assessing their current competence whereas upper-level students’ beliefs about their biology competence appear more likely to come from comparisons with how they have fared in their other college courses. Interview data support the quantitative data in , which shows that among students with majors other than Biology/Neuroscience, first-year students had higher competence-related beliefs than upper-level students. Although the interview dataset had an insufficient sample size of upper-level students who were majoring in Biology/Neuroscience, the interviews of two students from this group suggest heterogeneity among Biology/Neuroscience students who enroll in the class as upper-level students.
Theme 2: interest is related to major and is topic dependent
When asked about their level of interest in the course, most first-year students with Biology/Neuroscience majors described high levels of enjoyment and interest in the course material. One first-year Biology student said, ‘I’m definitely a lot more interested in bio than any of my other science classes’. Another emphasised, ‘I love that they give you a way to make sense of the world; those itty-bitty things that are invisible, but they make up so much of our life and it’s just really fascinating to find the building blocks of life in something that I had no idea about’. Another explained, ‘I’ve always been interested at a very young age. I just like learning how everything in the world works’.
First-year students with Biology/Neuroscience majors often described how their interest in the course was linked to their perceptions of high competence and low perceived effort cost. For example, one first-year student noted that they ‘really like biology’ because ‘it just comes more naturally to me’. Another first-year student explained that because they ‘have pretty high interest’ they ‘don’t mind taking the notes and coming to lecture’.
First-year students with majors outside Biology/Neuroscience reported relatively high interest but often described their interest as topic dependent. For example, a first-year Human Health Sciences major noted, ‘I find myself more interested in mutations and different diseases like cystic fibrosis … like just mutations that can happen in genes. I found myself interested in that more than the animal stuff like clades’. A first-year Equine Science major said, ‘I think it’s definitely like one of the classes where I am interested in it but it’s not like my passion. There’s different topics that I’m more interested in others. I find a lot of like the cell and the microbiology stuff to be really interesting, but more of the evolution and other stuff to be like a little bit more repetitive’.
Upper-level students in majors other than Biology/Neuroscience often described low interest in the course material. A junior Dietetics major remarked that ‘I’m interested in the bio, but compared to classes from my major that I have taken, I obviously lean more towards those’. A junior Equine Science major said that ‘on a 1 to 10 scale, I would say [my interest is] probably about a 4 out of 10. It’s just because I’m retaking this course, so I kind of feel a little bored’. A senior Kinesiology major linked his low interest with a low effort investment. He described his interest level as ‘a solid 3 out of 10’. He remarked that ‘I just don’t find it as interesting as a lot of the other classes I’m taking, so it’s kind of on the back burner’. One upper-level student (a sophomore Human Health Sciences major) linked their low interest to perceptions of lower competence: ‘I guess I’m only interested in it because I have to be, if that makes sense, because biology’s not necessarily my strong suit’.
Taken together, these data shed light on the quantitative data in (middle panel) showing that intrinsic value differed by both timing of enrollment and by major. The first-year students majoring in Biology/Neuroscience who took part in an interview expressed an interest in the biological topics and had higher competence-related beliefs from their high school experiences, which may synergise to increase perceptions of interest. First-year students from other majors may be less interested in the topics themselves, but their interest is higher than is upper-level students from majors outside Biology/Neuroscience, potentially because higher perceptions of competence in first-year students are linked to higher interest.
Theme 3: perceptions of utility value depend on both future coursework and career aspirations
When asked if the course would be relevant to them in the future, most first-year students majoring in Biology/Neuroscience described the course as being foundational to their success, both in their majors and in their future careers. For example, a first-year Biology major stated, ‘I’m very interested in the sciences and biology … I feel like it is integral to my future career, so it’s important that I am successful’. Another Biology major noted that ‘I think [the course] is definitely going to benefit me in the future when I’m taking, you know, like anatomy classes or I’m actually planning on doing research in a lab’. A first-year student with a Biology and Chemistry double major said that they had ‘always been interested at a very young age – it’s also really cool learning like how humans came to be and how all the animals develop and how just how everything works’.
Students with pre-health majors that required the course as a prerequisite for other classwork mentioned the course’s relevance for future learning, but not necessarily for their careers. A first-year Human Health Sciences major remarked that ‘if the information that I’m learning now is not like extremely relevant, it’ll get me ready for the biology classes that are extremely relevant just through understanding how to take the class and how to study’. When asked whether the course is relevant in terms of their future career, a first-year student majoring in Human Nutrition answered, ‘Yes, and no. Yes, in the sense that you need it in order to start learning things that are actually relevant. But no in the sense that, like what I’m learning right now, it’s just a stepping stone. It’s not the actual information [that] is relevant. This information is relevant to learn other information that is actually relevant’.
Other upper-level students often stated that the course was not closely relevant, either to future courses or their desired careers. For example, an upper-level student planning to attend medical school (sophomore Human Health Sciences major) said, ‘I don’t think [the course is] directly relevant to my future career. I don’t think I’ll be seeing patients and want to think of like mitochondria in the cell or whatever, but I do think that it’s a stepping stone, so I like to think of it more so that way, not necessarily that I’m studying something useless, but more so that I need to know this, in order to get to the next step’. A senior majoring in Kinesiology noted that the course was ‘not necessarily useful’ for their future healthcare career or for other courses in their major. A junior student Dietetics major explained that the course material would not be relevant because they would forget it: ‘I kind of probably will forget after I take this class. [I’ll] be like Okay, well, I took the class – I want to forget [it]’.
Together, these data suggest that students consider both future coursework and career aspirations when evaluating the utility of the course. These observations may partially explain the differences in utility value observed in (bottom panel). Students majoring in Biology/Neuroscience perceived the course as useful both for later coursework and their careers. First-year students with majors outside Biology/Neuroscience often perceived the relevance for later coursework, but not necessarily for their future careers. Quantitative data similarly revealed that upper-level students with majors other than Biology/Neuroscience had the lowest perceptions of the utility value of the course. It may be that many of these students perceive that the course is not relevant for future coursework because they are already enrolled in upper-level coursework in their majors, which presumably do not require knowledge of introductory biology. In addition, these students perceive that the topics will not be relevant for their future careers – a double hit to the perceived utility value of the course.
Theme 4: effort cost perceptions are influenced by other course demands, perceived competence, and competing priorities
When describing the cost the effort required in the course, students mentioned three general sources. First, and most commonly, students assessed their effort cost in relation to the other courses they were taking in the current semester. For example, a first-year student majoring in Biology explained having to prioritise another course because ‘chemistry is a harder subject for me, so I have to put a lot more effort in it to actually learn it’. A junior Biology major similarly confessed to putting forth less effort in biology: ‘I would say I had to put in a lot of effort but maybe not as much as like my chemistry course’. A sophomore student majoring in Agricultural and Medical Technology described just the opposite: that biology required more effort than non-science courses: ‘Well, I have a business minor, and in those classes I feel like it doesn’t take as much work to be successful. But like any science class, I feel like you have to put it a lot of effort to be successful’.
Other students related their perceptions of the effort required in biology to their perceived competence and science background. For example, a first-year Biology major explained that biology came easily. ‘I do have very good background knowledge of it, so I don’t have to put too much effort into it’. Another first-year Biology major said that they had a ‘science mind’ and previous experience in AP biology, which made the course ‘basically effortless’. In contrast, students with lower competence-related beliefs often described higher perceptions of effort. A junior Psychology major said that effort ‘depends, I think, on where you are. Like, do you have some science background? Some of [the course concepts] I don’t really remember at all doing because it’s probably new to me’. Because she felt that she had a weaker science background than her classmates, she had to put more effort into the course to be successful.
Upper-level students with majors outside Biology/Neuroscience were more likely than first-year students to describe competing priorities that affected their perception of the effort cost. For example, a junior Dietetics major stated that she ‘just feel[s] overwhelmed with so much other stuff to do that I kind of put it to blame on one class. I’m like, “Oh [biology] it’s too much homework”, but in reality, it’s probably not’. A senior Kinesiology major who had taken an introductory biology class during the height of the COVID-19 pandemic but needed to retake it due to low motivation said that ‘My other classes are pretty hard … .so [this class is] kind of on the back burner’.
Discussion
Understanding and promoting student motivation has important implications for retention and matriculation in STEM disciplines. Situated expectancy-value theory can provide a useful framework for understanding motivation and achievement in STEM courses. This study examined whether students’ motivation and achievement in a gateway introductory biology course differed as a function of students’ major area of study (Biology/Neuroscience vs. other STEM disciplines) and the timing of students’ enrollment (during their first year of college or later in college). We found that students majoring in Biology/Neuroscience were more highly motivated than students from other STEM majors. We also found that undergraduate students’ competence-related beliefs, task values, and perceived costs differed based on the timing of their course enrollment. In general, students who enrolled in Introductory Biology I after their first year reported lower motivation than those who enrolled in the course during their first year of college.
Qualitative analysis of interview data largely supported the quantitative findings and provided important clues about the varied factors affecting student motivation. Our findings and evidence from prior studies indicate that many factors contribute to the motivational differences observed here. First, students typically rate their perceived competence and expectancies for success by recalling how they have done similar tasks in the past (Eccles and Wigfield Citation2020). Upper-level students have already experienced challenges in college-level courses, whereas first-year students have only their high school experiences to draw on, which were likely to be highly successful. Second, upper-level students may be more likely than first-year students to have competing priorities that affect their effort perceptions. A third possibility is that upper-level students with majors outside Biology/Neuroscience may believe that, because their biology course is ‘introductory’ or is not a prerequisite for future coursework, it is less relevant to them. Although we found support for each of these possibilities in student interviews, each should be further explored.
When interviewed, many students with majors outside Biology/Neuroscience noted that the nature of their interest and their perceptions of relevance depended on the course topic. For example, multiple students specifically mentioned that they were not interested in evolution, nor did they think that an understanding of evolutionary mechanisms was important for their future career. Given the rapid evolution of the SARS CoV-2 virus over the past three years and the ability of new variants to infect individuals with prior infection- or vaccine-based immunity, we were surprised that many students did not perceive the relevance of understanding evolution, especially those with career aspirations in healthcare. Perceptions of topic-specific relevance should be explored in future studies.
As has been found in previous studies (e.g. Perez, Dai, et al. Citation2019; Robinson et al. Citation2019), competence-related beliefs were the strongest motivational predictor of final course grades in this study. Students who majored in Biology/Neuroscience or took the course during their first year in college were more likely to feel competent and predict they would be successful in the course, which was linked to better performance.
Perceived costs as motivational factors have been comparatively understudied, but researchers have begun to document their influence on students’ persistence and academic outcomes in STEM (Perez, Wormington, et al. Citation2019). In this study, perceived effort cost significantly mediated the association between students’ major and their final course grade. Students who were Biology/Neuroscience majors perceived less effort cost in biology, which led to better performance in the course.
Our qualitative interview data suggest that higher levels of perceived effort were at least partially due to lower competence-related beliefs. In other words, if students perceive that their biology abilities are not high, they may also believe that more effort is required to be successful. These conditional relationships among students’ motivational variables should be further examined as they were beyond the scope of our analyses.
We found that task values were not significantly associated with grade outcomes. This finding aligns with studies that have shown that values, while important predictors of student choices about whether to change majors, are not consistent predictors of academic performance (Eccles and Wigfield Citation2002; Perez, Cromley, and Kaplan Citation2014).
Limitations and future directions
We acknowledge several limitations to this study that should be considered. First, the motivation variables investigated here were measured at a single time point early in the semester (before the first exam). Examining motivation at multiple time points could be useful for understanding possible changes in motivation across the semester. Second, data were collected during a single fall semester. Motivational processes might look different in a spring semester, particularly for first-year students, most of whom would have had completed at least one semester at the college level. Because the COVID-19 pandemic resulted in large-scale changes to the high school experience for students enrolled in their first semester of college during the Fall 2021 semester, we did not directly compare the high school GPAs of first-year and upper-level students. We were also unable to investigate the possible effects of prior experience in biology courses on motivation and course outcomes beyond that self-reported by our interviewees. Our interview population was limited and may not be representative of the range of student experiences. In this study, we did not specifically compare how first-year and upper-level students perceived the active learning practices utilized in the course and how these perceptions may have influenced their motivation. These would be fruitful next steps in investigating reasons why students’ motivation levels differ.
Situated expectancy-value theory posits that learners’ social identities and the broader social environment can influence competence beliefs, values, and perceived costs (Eccles and Wigfield Citation2020; Wigfield, Muenks, and Eccles Citation2021). We focused this study on students’ major and enrollment timing, but it is possible that accounting for students’ social identities and other demographic characteristics would have helped explain motivational differences. For instance, upper-level status might disproportionally affect the motivation and outcomes of first-generation students or those belonging to historically minoritized groups. It may be that upper-level status is merely a reflection of other variables not analysed in this study.
Finally, the data presented here show correlations between timing of enrollment, motivation, and final course grades, but this does not imply a causal relationship between variables. We cannot rule out variance caused by higher-achieving students being more motivated because they earn high grades, and low-achieving students feeling less motivated because of their lower grades. In other words, we cannot definitively determine the directionality of the relationship between variables in our path model. Nevertheless, experimental approaches that use random assignment would not likely be feasible nor advisable in such a study. The time lag used in the assessment of motivation variables and outcomes in this study, and our use of qualitative data to confirm quantitative findings, strengthen the inferences we made about the effects of course timing on motivation and achievement.
Implications for instructors
The findings presented here indicate that upper-level students, especially those with majors outside Biology/Neuroscience, are a unique population who may need additional supports to maximise their motivation and academic outcomes. Importantly, nearly half of the students enrolled in the course studied here were beyond their first year in college and/or were majoring in academic disciplines outside Biology/Neuroscience. Findings suggest that a large proportion of students enrolled in introductory biology courses may not be receiving the motivational supports to help them be maximally successful.
Our findings point to several implications for pedagogical support of upper-level students and those who are required to enroll in courses from disciplines outside of their academic majors. While our study was performed in a large-enrollment introductory biology course at a large, public institution, given the alignment of our findings with other studies, we suspect that the interventions suggested by this study may be generalisable to other course settings and institution types. First, interventions that provide motivational support for upper-level students in introductory courses could be useful. Given the strong relationship between competence-related beliefs and performance, instructors might look for ways to support learners’ beliefs about their own capabilities. Providing early opportunities for success helps build a foundation of perceived mastery that undergirds self-efficacy (Usher et al. Citation2019). Providing testimonials from upper-level students who have succeeded in the course may also support students’ beliefs that they too can be successful. When students feel stressed or overwhelmed, they also tend to feel less capable, so instructors can implement stress-reducing techniques that help remind students that they can manage the content and workload of introductory biology (Schunk and DiBenedetto Citation2020; Usher et al. Citation2023).
Whereas first-year students might readily see the utility of their coursework, upper-level students might especially benefit from targeted interventions that help them understand the relevance and usefulness of introductory biology to their lives (Tibbetts et al. Citation2016). Given that many students in our study did not understand the relevance of evolutionary principles to their future careers, it may be especially important to develop curricular supports to help students better understand these connections. Introducing students to the field of evolutionary medicine, the application of insights from evolution to medicine and public health, is one potential approach that has broad support from researchers and practitioners (Graves et al. Citation2016; Natterson-Horowitz et al. Citation2023). Studies have shown that writing about the personal relevance of course topics in introductory biology improves retention and performance (Canning et al. Citation2018). Integrating the broader social context into biology courses is another approach for increasing students’ perceptions of the relevance of biology. Doing so might emphasise the importance of biological literacy, which enhances perceived value of biology (Chamany, Allen, and Tanner Citation2008). These interventions may be particularly beneficial for first-generation students and those from historically underserved groups (Harackiewicz et al. Citation2016). Interventions aimed at decreasing the perceived costs of biology coursework may also benefit upper-level students. In a recent study, a cost-focused motivational intervention was associated with higher exam scores in an introductory biology course (Rosenzweig, Song, and Clark Citation2022). These interventions have the potential to foster a more motivationally supportive environment for all students, thereby contributing to the nationwide effort to increase inclusivity and belonging in gateway science courses.
Availability of data and materials
The interview datasets used and/or analysed during the current study are available from the corresponding author on reasonable request.
Supplemental Material
Download MS Word (708.7 KB)Acknowledgments
We thank Licia Henneberg, Anastacia Cole, Mayson Spillman, Shelby Vinsand, and Jaylene Patterson for helpful feedback on the interview protocol and for performing student interviews. We thank Stacey Slone for statistical consultations. We thank William Bailey, Mary Foley, and Peter Mirabito for critical reading of the manuscript.
Disclosure statement
No potential conflict of interest was reported by the author(s).
Supplementary material
Supplemental data for this article can be accessed online at https://doi.org/10.1080/00219266.2024.2342801
Additional information
Funding
References
- Anderman, E. M., and L. H. Anderman. 2020. Classroom Motivation: Linking Research to Teacher Practice. 3rd ed. New York: Routledge.
- Asparouhov, T., and B. Muthén, 2018. SRMR in Mplus. http://www.statmodel.com/download/SRMR2.pdf.
- Bastyr, C., C. Johnson, R. Lakhan, and J. W. Wainman. 2022. “Reducing Chemistry Casualties: Supporting Women Enrolled in General Chemistry I Not During Their First Term of College with Active Learning.” Journal of Chemical Education 99 (9): 3089–3095. https://doi.org/10.1021/acs.jchemed.1c01279.
- Canning, E. A., J. M. Harackiewicz, S. J. Priniski, C. A. Hecht, Y. Tibbetts, and J. S. Hyde. 2018. “Improving Performance and Retention in Introductory Biology with a Utility-Value Intervention.” Journal of Educational Psychology 110 (6): 834–849. https://doi.org/10.1037/edu0000244.
- Chamany, K., D. Allen, and K. Tanner. 2008. “Making Biology Learning Relevant to Students: Integrating People, History, and Context into College Biology Teaching.” CBE—Life Sciences Education 7 (3): 267–278. https://doi.org/10.1187/cbe.08-06-0029.
- Chouinard, R., and N. Roy. 2008. “Changes in High-School Students’ Competence Beliefs, Utility Value and Achievement Goals in Mathematics.” The British Journal of Educational Psychology 78 (1): 31–50. https://doi.org/10.1348/000709907X197993.
- Corpus, J. H., K. A. Robinson, and S. V. Wormington. 2020. “Trajectories of Motivation and Their Academic Correlates Over the First Year of College.” Contemporary Educational Psychology 63 (October): 101907. https://doi.org/10.1016/j.cedpsych.2020.101907.
- Creswell, J. W., and V. L. Plano Clark. 2018. Designing and Conducting Mixed Methods Research. 3rd ed. Thousand Oaks, CA: Sage.
- Cromley, J. G., T. Perez, and A. Kaplan. 2016. “Undergraduate STEM Achievement and Retention: Cognitive, Motivational, and Institutional Factors and Solutions.” Policy Insights from the Behavioral and Brain Sciences 3 (1): 4–11. https://doi.org/10.1177/2372732215622648.
- Dai, T., and J. G. Cromley. 2014. “Changes in Implicit Theories of Ability in Biology and Dropout from STEM Majors: A Latent Growth Curve Approach.” Contemporary Educational Psychology 39 (3): 233–247. https://doi.org/10.1016/j.cedpsych.2014.06.003.
- Deslauriers, L., L. S. McCarty, K. Miller, K. Callaghan, and G. Kestin. 2019. “Measuring Actual Learning versus Feeling of Learning in Response to Being Actively Engaged in the Classroom.” Proceedings of the National Academy of Sciences 116 (39): 19251–19257. https://doi.org/10.1073/pnas.1821936116.
- Eccles, J. S., T. F. Adler, R. Futterman, S. B. Goff, C. M. Kaczala, J. L. Meece, and C. Midgley. 1983. “Expectancies, Values, and Academic Behaviors.” In Achievement and Achievement Motives: Psychological and Sociological Approaches, edited by J. T. Spence, 75–146. San Francisco, CA: W. H. Freeman and Company.
- Eccles, J. S., and A. Wigfield. 2002. “Motivational Beliefs, Values, and Goals.” Annual Review of Psychology 53 (1): 109–132. https://doi.org/10.1146/annurev.psych.53.100901.135153.
- Eccles, J. S., and A. Wigfield. 2020. “From Expectancy-Value Theory to Situated Expectancy-Value Theory: A Developmental, Social Cognitive, and Sociocultural Perspective on Motivation.” Contemporary Educational Psychology 61:101859. https://doi.org/10.1016/j.cedpsych.2020.101859.
- England, B. J., J. R. Brigati, and E. E. Schussler. 2017. “Student Anxiety in Introductory Biology Classrooms: Perceptions About Active Learning and Persistence in the Major.” Public Library of Science ONE 12 (8): e0182506. https://doi.org/10.1371/journal.pone.0182506.
- Fredricks, J. A., and J. S. Eccles. 2002. “Children’s Competence and Value Beliefs from Childhood Through Adolescence: Growth Trajectories in Two Male-Sex-Typed Domains.” Developmental Psychology 38 (4): 519–533. https://doi.org/10.1037/0012-1649.38.4.519.
- Freeman, S., D. Haak, and M. P. Wenderoth. 2011. “Increased Course Structure Improves Performance in Introductory Biology.” CBE Life Sciences Education 10 (2): 175–186. https://doi.org/10.1187/cbe.10-08-0105.
- Gasiewski, J. A., M. K. Eagan, G. A. Garcia, S. Hurtado, and M. J. Chang. 2012. “From Gatekeeping to Engagement: A Multicontextual, Mixed Method Study of Student Academic Engagement in Introductory STEM Courses.” Research in Higher Education 53 (2): 229–261. https://doi.org/10.1007/s11162-011-9247-y.
- Graves, J. L., Jr, C. Reiber, A. Thanukos, M. Hurtado, and T. Wolpaw. 2016. “Evolutionary Science As a Method to Facilitate Higher Level Thinking and Reasoning in Medical Training.” Evolution, Medicine, and Public Health 2016 (1): 358–368. https://doi.org/10.1093/emph/eow029.
- Harackiewicz, J. M., E. A. Canning, Y. Tibbetts, S. J. Priniski, and J. S. Hyde. 2016. “Closing Achievement Gaps with a Utility-Value Intervention: Disentangling Race and Social Class.” Journal of Personality & Social Psychology 111 (5): 745–765. https://doi.org/10.1037/pspp0000075.
- Hecht, C. A., J. M. Harackiewicz, S. J. Priniski, E. A. Canning, Y. Tibbetts, and J. S. Hyde. 2019. “Promoting Persistence in the Biological and Medical Sciences: An Expectancy-Value Approach to Intervention.” Journal of Educational Psychology 111 (8): 1462–1477. https://doi.org/10.1037/edu0000356.
- Hu, L. T., and P. M. Bentler. 1999. “Cutoff Criteria for Fit Indexes in Covariance Structure Analysis: Conventional Criteria versus New Alternatives.” Structural Equation Modeling: A Multidisciplinary Journal 6 (1): 1–55. https://doi.org/10.1080/10705519909540118.
- Jacobs, J. E., S. Lanza, D. W. Osgood, J. S. Eccles, and A. Wigfield. 2002. “Changes in Children’s Self-Competence and Values: Gender and Domain Differences Across Grades One Through Twelve.” Child Development 73 (2): 509–527. https://doi.org/10.1111/1467-8624.00421.
- Jiang, S., S. D. Simpkins, and J. S. Eccles. 2020. “Individuals’ Math and Science Motivation and Their Subsequent STEM Choices and Achievement in High School and College: A Longitudinal Study of Gender and College Generation Status Differences.” Developmental Psychology 56 (11): 2137–2151. https://doi.org/10.1037/dev0001110.
- Knight, J. K., and M. K. Smith. 2010. “Different but Equal? How Nonmajors and Majors Approach and Learn Genetics.” CBE Life Sciences Education 9 (1): 34–44. https://doi.org/10.1187/cbe.09-07-0047.
- Komarraju, M., and D. Nadler. 2013. “Self-Efficacy and Academic Achievement: Why Do Implicit Beliefs, Goals, and Effort Regulation Matter?” Learning & Individual Differences 25:67–72. https://doi.org/10.1016/j.lindif.2013.01.005.
- Kosovich, J. J., J. K. Flake, and C. S. Hulleman. 2017. “Short-Term Motivation Trajectories: A Parallel Process Model of Expectancy-Value.” Contemporary Educational Psychology 49:130–139. https://doi.org/10.1016/j.cedpsych.2017.01.004.
- Lauermann, F., Y. Tsai, and J. S. Eccles. 2017. “Math-Related Career Aspirations and Choices within Eccles et Al.’s Expectancy–Value Theory of Achievement-Related Behaviors.” Developmental Psychology 53 (8): 1540–1559. https://doi.org/10.1037/dev0000367.
- Linnenbrink-Garcia, L., and E. A. Patall. 2015. “Motivation.” In Handbook of Educational Psychology, edited by L. Corno and E. M. Anderman, 91–103. New York: Routledge.
- Marsh, H. W., U. Trautwein, O. Lüdtke, O. Köller, and J. Baumert. 2005. “Academic Self-Concept, Interest, Grades, and Standardized Test Scores: Reciprocal Effects Models of Causal Ordering.” Child Development 76 (2): 397–416. https://doi.org/10.1111/j.1467-8624.2005.00853.x.
- Maxwell, J. A. 2010. “Using Numbers in Qualitative Research.” Qualitative Inquiry 16 (6): 475–482. https://doi.org/10.1177/1077800410364740.
- Meaders, C. L., A. K. Lane, A. I. Morozov, J. K. Shuman, E. S. Toth, M. Stains, M. R. Stetzer, E. Vinson, B. A. Couch, and M. K. Smith. 2020. “Undergraduate Student Concerns in Introductory STEM Courses: What They Are, How They Change, and What Influences Them.” Journal for STEM Education Research 3 (2): 195–216. https://doi.org/10.1007/s41979-020-00031-1.
- Moore, R. 2007. “Academic Motivation and Performance of Developmental Education Biology Students.” Journal of Developmental Education 31 (1): 24–34.
- Morse, J. M. 1997. “Perfectly Healthy, but Dead: The Myth of Inter-Rater Reliability.” Qualitative Health Research 7 (4): 445–447. https://doi.org/10.1177/104973239700700401.
- Nacionales, E. N., P. M. Muyong, and J. C. Gavasan. 2016. “The Impact of Motivation and Learning Strategies As Predictors of Biology Performance Among Non-Science Majors.” Asia Pacific Higher Education Research Journal 3 (2): 14–22.
- National Center for Education Statistics. 2013. STEM Attrition: College Students’ Paths into and Out of STEM Fields. Statistical Analysis Report U.S. Department of Education. https://nces.ed.gov/pubs2014/2014001rev.pdf.
- Natterson-Horowitz, B., A. Aktipis, M. Fox, P. D. Gluckman, F. M. Low, R. Mace, A. Read, P. E. Turner, and D. T. Blumstein. 2023. “The Future of Evolutionary Medicine: Sparking Innovation in Biomedicine and Public Health.” Frontiers in Science 1:997136. https://doi.org/10.3389/fsci.2023.997136.
- Nolen, S. B. 2020. “A Situative Turn in the Conversation on Motivation Theories.” Contemporary Educational Psychology 61:101866. https://doi.org/10.1016/j.cedpsych.2020.101866.
- Perez, T., J. G. Cromley, and A. Kaplan. 2014. “The Role of Identity Development, Values, and Costs in College STEM Retention.” Journal of Educational Psychology 106 (1): 315–329. https://doi.org/10.1037/a0034027.
- Perez, T., T. Dai, A. Kaplan, J. G. Cromley, W. D. Brooks, A. C. White, K. R. Mara, and M. J. Balsai. 2019. “Interrelations Among Expectancies, Task Values, and Perceived Costs in Undergraduate Biology Achievement.” Learning & Individual Differences 72:26–38. https://doi.org/10.1016/j.lindif.2019.04.001.
- Perez, T., S. V. Wormington, M. M. Barger, R. D. Schwartz‐Bloom, Y. K. Lee, and L. Linnenbrink‐Garcia. 2019. “Science Expectancy, Value, and Cost Profiles and Their Proximal and Distal Relations to Undergraduate Science, Technology, Engineering, and Math Persistence.” Science Education 103 (2): 264–286. https://doi.org/10.1002/sce.21490.
- Preacher, K. J., and A. F. Hayes. 2008. “Asymptotic and Resampling Strategies for Assessing and Comparing Indirect Effects in Multiple Mediator Models.” Behavior Research Methods 40 (3): 879–891. https://doi.org/10.3758/BRM.40.3.879.
- Renninger, K. A., and S. Hidi. 2016. The Power of Interest for Motivation and Engagement. New York, NY: Routledge.
- Robinson, K. A., Y.-K. Lee, E. A. Bovee, T. Perez, S. P. Walton, D. Briedis, and L. Linnenbrink-Garcia. 2019. “Motivation in Transition: Development and Roles of Expectancy, Task Values, and Costs in Early College Engineering.” Journal of Educational Psychology 111 (6): 1081–1102. https://doi.org/10.1037/edu0000331.
- Rosenzweig, E. Q., Y. Song, and S. Clark. 2022. “Mixed Effects of a Randomized Trial Replication Study Testing a Cost-Focused Motivational Intervention.” Learning & Instruction 82:101660. https://doi.org/10.1016/j.learninstruc.2022.101660.
- Rosenzweig, E. Q., A. Wigfield, and J. S. Eccles. 2019. “Expectancy-Value Theory and Its Relevance for Student Motivation and Learning.” In The Cambridge Handbook of Motivation and Learning, edited by K. A. Renninger and S. E. Hidi, 617–644. United Kingdom: Cambridge University Press.
- Sathy, V., and K. A. Hogan. 2022. “How to Make Your Teaching More Inclusive”. The Chronicle of Higher Education. Accessed December 30, 2022. https://www.chronicle.com/article/how-to-make-your-teaching-more-inclusive/.
- Schunk, D. H., and M. K. DiBenedetto. 2020. “Motivation and Social Cognitive Theory.” Contemporary Educational Psychology 60 (1): 101832. https://doi.org/10.1016/j.cedpsych.2019.101832.
- Stinebrickner, R., and T. R. Stinebrickner. 2014. “A Major in Science? Initial Beliefs and Final Outcomes for College Major and Dropout.” The Review of Economic Studies 81 (1): 426–472. https://doi.org/10.1093/restud/rdt025.
- Tibbetts, Y., J. M. Harackiewicz, S. J. Priniski, and E. A. Canning. 2016. “Broadening Participation in the Life Sciences with Social–Psychological Interventions.” CBE—Life Sciences Education 15 (3): es4. https://doi.org/10.1187/cbe.16-01-0001.
- Tomkin, J. H., and M. West. 2022. “STEM Courses Are Harder: Evaluating Inter-Course Grading Disparities with a Calibrated GPA Model.” International Journal of STEM Education 9 (1). https://doi.org/10.1186/s40594-022-00343-1.
- Usher, E. L., A. R. Butz, X.-Y. Chen, C. J. Ford, J. Han, N. A. Mamaril, D. B. Morris, P. Peura, and R. R. Piercey. 2023. “Supporting Self-Efficacy Development from Primary School to the Professions: A Guide for Educators.” Theory into Practice 63 (3): 266–278. https://doi.org/10.1080/00405841.2023.2226559.
- Usher, E. L., C. J. Ford, C. R. Li, and B. L. Weidner. 2019. “Sources of Math and Science Self-Efficacy in Rural Appalachia: A Convergent Mixed Methods Study.” Contemporary Educational Psychology 57:32–53. https://doi.org/10.1016/j.cedpsych.2018.10.003.
- Watt, H. M. 2004. “Development of Adolescents’ Self-Perceptions, Values, and Task Perceptions According to Gender and Domain in 7th- Through 11th-Grade Australian Students.” Child Development 75 (5): 1556–1574. https://doi.org/10.1111/j.1467-8624.2004.00757.x.
- Wigfield, A., K. Muenks, and J. S. Eccles. 2021. “Achievement Motivation: What We Know and Where We Are Going.” Annual Review of Developmental Psychology 3:87–111. https://doi.org/10.1146/annurev-devpsych-050720-103500.